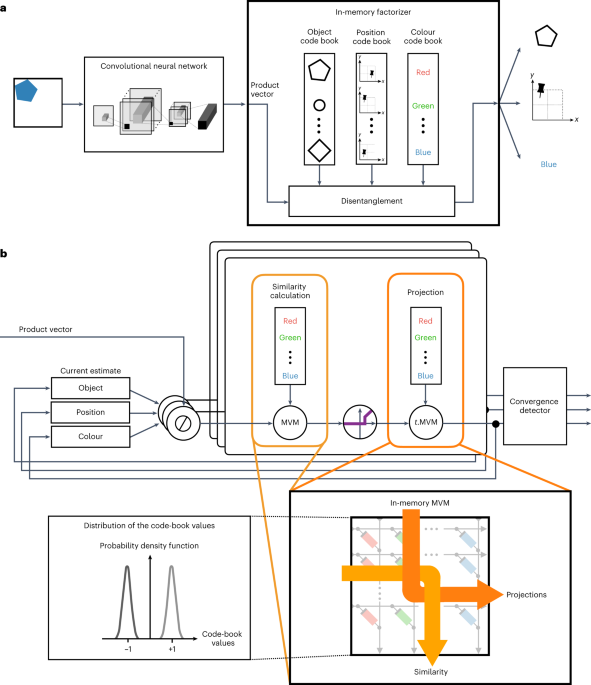
Feldman, J. The neural binding problem(s). Cogn. Neurodyn. 7, 1–11 (2013).
Land, E. H. & McCann, J. J. Lightness and retinex theory. J. Opt. Soc. Am. 61, 1–11 (1971).
Barrow, H. G. & Tenenbaum, J. M. in Computer Vision Systems 3–26 (Academic Press, 1978).
Adelson, E. & Pentland, A. in The Perception of Shading and Reflectance 409–424 (Cambridge Univ. Press, 1996).
Barron, J. T. & Malik, J. Shape, illumination and reflectance from shading. IEEE Trans. Pattern Anal. Mach. Intell. 37, 1670–1687 (2015).
Memisevic, R. & Hinton, G. E. Learning to represent spatial transformations with factored higher-order Boltzmann machines. Neural Comput. 22, 1473–1492 (2010).
Burak, Y., Rokni, U., Meister, M. & Sompolinsky, H. Bayesian model of dynamic image stabilization in the visual system. Proc. Natl Acad. Sci. USA 107, 19525–19530 (2010).
Cadieu, C. F. & Olshausen, B. A. Learning intermediate-level representations of form and motion from natural movies. Neural Comput. 24, 827–866 (2012).
Anderson, A. G., Ratnam, K., Roorda, A. & Olshausen, B. A. High-acuity vision from retinal image motion. J. Vision 20, 34 (2020).
Smolensky, P. Tensor product variable binding and the representation of symbolic structures in connectionist systems. Artif. Intell. 46, 159–216 (1990).
Jackendoff, R. Foundations of Language: Brain, Meaning, Grammar, Evolution (Oxford Univ. Press, 2002).
Hummel, J. E. & Holyoak, K. J. Distributed representations of structure: a theory of analogical access and mapping. Psychol. Rev. 104, 427–466 (1997).
Kanerva, P. in Advances in Analogy Research: Integration of Theory and Data from the Cognitive, Computational and Neural Sciences 164–170 (New Bulgarian Univ., 1998).
Kanerva, P. Pattern completion with distributed representation. In International Joint Conference on Neural Networks 1416–1421 (IEEE, 1998).
Plate, T. A. Analogy retrieval and processing with distributed vector representations. Expert Syst. Int. J. Knowledge Eng. Neural Netw. 17, 29–40 (2000).
Gayler, R. W. & Levy, S. D. A distributed basis for analogical mapping: new frontiers in analogy research. In New Frontiers in Analogy Research, Second International Conference on the Analogy 165–174 (New Bulgarian University Press, 2009).
Gayler, R. W. Vector symbolic architectures answer Jackendoff’s challenges for cognitive neuroscience. In Joint International Conference on Cognitive Science 133–138 (Springer, 2003).
Plate, T. A. Holographic reduced representations. IEEE Trans. Neural Netw. 6, 623–641 (1995).
Plate, T. A. Holographic Reduced Representations: Distributed Representation for Cognitive Structures (Stanford Univ., 2003).
Kanerva, P. Hyperdimensional computing: an introduction to computing in distributed representation with high-dimensional random vectors. Cogn. Comput. 1, 139–159 (2009).
Frady, E. P., Kent, S. J., Olshausen, B. A. & Sommer, F. T. Resonator networks, 1: an efficient solution for factoring high-dimensional, distributed representations of data structures. Neural Comput. 32, 2311–2331 (2020).
Hersche, M., Zeqiri, M., Benini, L., Sebastian, A. & Rahimi, A. A neuro-vector-symbolic architecture for solving Raven’s progressive matrices. Nat. Mach. Intell. https://doi.org/10.1038/s42256-023-00630-8 (2023).
Lanza, M. et al. Memristive technologies for data storage, computation, encryption and radio-frequency communication. Science 376, eabj9979 (2022).
Sebastian, A., Le Gallo, M., Khaddam-Aljameh, R. & Eleftheriou, E. Memory devices and applications for in-memory computing. Nat. Nanotechnol. 15, 529–544 (2020).
Wang, Z. et al. Resistive switching materials for information processing. Nat. Rev. Mater. 5, 173–195 (2020).
Kent, S. J., Frady, E. P., Sommer, F. T. & Olshausen, B. A. Resonator networks, 2: factorization performance and capacity compared to optimization-based methods. Neural Comput. 32, 2332–2388 (2020).
Wong, H.-S. P. & Salahuddin, S. Memory leads the way to better computing. Nat. Nanotechnol. 10, 191–194 (2015).
Chua, L. Resistance switching memories are memristors. Appl. Phys. A 102, 765–783 (2011).
Shin, J. H., Jeong, Y. J., Zidan, M. A., Wang, Q. & Lu, W. D. Hardware acceleration of simulated annealing of spin glass by RRAM crossbar array. In Proc. IEEE International Electron Devices Meeting 3.3.1–3.3.4 (IEEE, 2018).
Bojnordi, M. N. & Ipek, E. Memristive Boltzmann machine: a hardware accelerator for combinatorial optimization and deep learning. In Proc. IEEE International Symposium on High Performance Computer Architecture 1–13 (IEEE, 2016).
Mahmoodi, M. R., Prezioso, M. & Strukov, D. B. Versatile stochastic dot product circuits based on nonvolatile memories for high performance neurocomputing and neurooptimization. Nat. Commun. 10, 5113 (2019).
Borders, W. A. et al. Integer factorization using stochastic magnetic tunnel junctions. Nature 573, 390–393 (2019).
Wan, W. et al. 33.1 A 74 TMACS/W CMOS-RRAM neurosynaptic core with dynamically reconfigurable dataflow and in-situ transposable weights for probabilistic graphical models. In Proc. IEEE International Solid-State Circuits Conference 498–500 (IEEE, 2020).
Kumar, S., Strachan, J. P. & Williams, R. S. Chaotic dynamics in nanoscale NbO2 Mott memristors for analogue computing. Nature 548, 318–321 (2017).
Cai, F. et al. Power-efficient combinatorial optimization using intrinsic noise in memristor Hopfield neural networks. Nat. Electron. 3, 409–418 (2020).
Yang, K. et al. Transiently chaotic simulated annealing based on intrinsic nonlinearity of memristors for efficient solution of optimization problems. Sci. Adv. 6, eaba9901 (2020).
Khaddam-Aljameh, R. et al. Hermes core—a 14nm CMOS and PCM-based in-memory compute core using an array of 300ps/LSB linearized CCO-based ADCs and local digital processing. In 2021 Symposium on VLSI Circuits 1–2 (IEEE, 2021).
Tuma, T., Pantazi, A., Le Gallo, M., Sebastian, A. & Eleftheriou, E. Stochastic phase-change neurons. Nat. Nanotechnol. 11, 693–699 (2016).
Le Gallo, M., Krebs, D., Zipoli, F., Salinga, M. & Sebastian, A. Collective structural relaxation in phase-change memory devices. Adv. Electron. Mater. 4, 1700627 (2018).
Le Gallo, M. & Sebastian, A. An overview of phase-change memory device physics. J. Phys. D Appl. Phys. 53, 213002 (2020).
Zhang, C., Gao, F., Jia, B., Zhu, Y. & Zhu, S.-C. RAVEN: a dataset for relational and analogical visual reasoning. In Proc. IEEE/CVF Conference on Computer Vision and Pattern Recognition (CVPR) 5312–5322 (IEEE, 2019).
Kent, S. Multiplicative Coding and Factorization in Vector Symbolic Models of Cognition. PhD thesis, Univ. California (2020).
Kleyko, D. et al. Integer factorization with compositional distributed representations. In Proc. 9th Annual Neuro-Inspired Computational Elements Conference 73–80 (ACM, 2022).
Li, J. et al. Low angle annular dark field scanning transmission electron microscopy analysis of phase change material. In Proc. International Symposium for Testing and Failure Analysis 2021 206–210 (ASM, 2021).
- SEO Powered Content & PR Distribution. Get Amplified Today.
- Platoblockchain. Web3 Metaverse Intelligence. Knowledge Amplified. Access Here.
- Source: https://www.nature.com/articles/s41565-023-01357-8
- ][p
- 1
- 10
- 11
- 1996
- 1998
- 2011
- 2012
- 2014
- 2016
- 2017
- 2018
- 2019
- 2020
- 2021
- 2022
- 2023
- 28
- 39
- 7
- 70
- 8
- 9
- a
- academic
- acceleration
- accelerator
- access
- ACM
- AL
- analysis
- and
- annual
- answer
- applications
- architecture
- ARE
- Array
- article
- based
- basis
- Bayesian
- Better
- binding
- Bulgarian
- by
- california
- cambridge
- Capacity
- challenges
- change
- click
- Coding
- cognitive
- Collective
- Communication
- compared
- completion
- computation
- Compute
- computer
- Computer Vision
- computing
- Conference
- Core
- CVPR
- Dark
- data
- data storage
- deep
- deep learning
- device
- Devices
- digital
- distributed
- DOT
- dynamic
- dynamically
- dynamics
- e
- efficient
- elements
- encryption
- Ether (ETH)
- Failure
- field
- For
- form
- from
- Frontiers
- GAO
- glass
- Hardware
- hermes
- High
- holographic
- http
- HTTPS
- IEEE
- image
- in
- information
- International
- intrinsic
- Introduction
- joint
- knowledge
- Leads
- learning
- LINK
- local
- Low
- machine
- Machines
- mapping
- material
- materials
- Memories
- Memory
- methods
- Microscopy
- model
- models
- motion
- Movies
- Natural
- Nature
- networks
- Neural
- neural networks
- Neurons
- Neuroscience
- New
- Noise
- of
- on
- optimization
- overview
- Oxford
- Pattern
- perception
- performance
- phase
- Physics
- plato
- Plato Data Intelligence
- PlatoData
- press
- problems
- processing
- Product
- progressive
- random
- recognition
- Reduced
- relaxation
- represent
- representation
- research
- Resistance
- s
- scanning
- SCI
- Shape
- solution
- Solving
- Spatial
- Spin
- stanford
- storage
- structural
- structure
- Symposium
- system
- Systems
- Technologies
- Testing
- The
- to
- transformations
- university
- versatile
- vision
- W
- Way..
- with
- zephyrnet