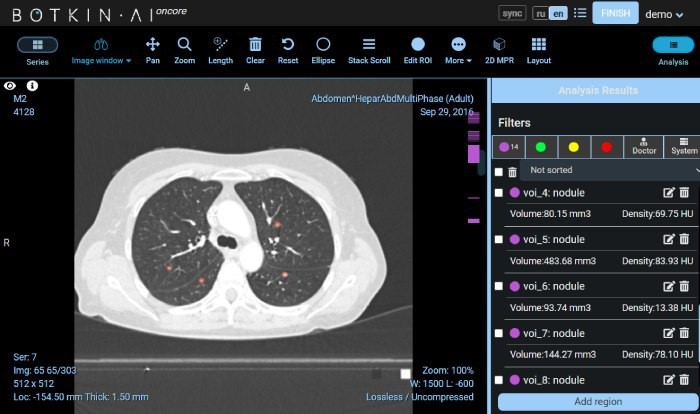
NMPA released the “Clinical Evaluation Guideline on Artificial Intelligence-assisted Detection Medical Devices (Software)” on November 7, 2023.
The guideline refers to FDA guidance of “Computer-Assisted Detection Devices Applied to Radiology Images and Radiology Device Data” and “Clinical Performance Assessment: Considerations for Computer-Assisted Detection Devices Applied to Radiology Images and Radiology Device Data”.
For an English copy of the guideline, please email info@ChinaMedDevice.com. We charge a nominal fee for the translation.
For ultrasound imaging AI software guideline, please click HERE
For approval of Ischemic stroke detection AI software, click HERE
Application Scope
AI-assisted detection devices refer to computer-based AI algorithms that can include functions such as pattern recognition and data analysis. They assist clinical physicians in making corresponding diagnostic and treatment decisions by identifying, marking, and highlighting possible areas of anomalies or lesions. They can be standalone software or embedded software. They are Class III devices. Common examples include those for the detection of lesions or anomalies related to lung nodules, breast nodules, fractures, vascular narrowing, colon polyps.
The products may also include non-assistive decision functions, such as structured report generation, before-and-after image comparisons, segmentation of normal anatomical structures (such as lung lobes, ribs, etc.), dimension measurements, CT value measurements, and other clinical functions, as well as data storage, transmission, and other non-clinical functions.
Clinical Trial Design
The trials aim to evaluate the diagnostic performance of such products within their intended applications, including their usability and safety. Here’s a summary of the key points:
Clinical Trial Objectives: Clinical trials for AI-assisted diagnostic products primarily focus on enhancing the accuracy of disease detection by healthcare professionals. They may involve controlled trials, including randomized parallel, crossover, or multiple reader multiple case (MRMC) designs.
Study Subjects:
- Imaging Samples: Trials often use defined inclusion and exclusion criteria to collect imaging samples from the target population. Real-world data from clinical practice can be considered.
- Reader Variation: Due to variability among readers and their interaction with patient samples, clinical trials often include radiologists as study subjects, especially for non-real-time imaging products.
Evaluation Metrics: Key evaluation metrics should align with the product’s design characteristics. Sensitivity, specificity, and ROC or related diagnostic accuracy measures are preferred as primary endpoints due to their robustness against disease prevalence variations.
Clinical Reference Standards: Applicants must explain the selection and construction of clinical reference standards. Options include using clinically confirmed results or expert panels’ judgments as reference standards, with clear criteria for expert qualifications and decision-making processes.
Sample Size Estimation and Statistical Analysis: Sample size calculations should consider trial design, primary endpoints, and statistical requirements. Transparency about formulas, parameters, and software used is essential. MRMC trial design specifics should be outlined, including effect sizes, power, alpha levels, and the basis for determining efficacy/non-inferiority thresholds.
Clinical Trial Training: Training for readers, including radiologists and expert panels, is crucial to minimize bias. It should cover trial procedures, terminology, and data sample evaluation standards. Maintaining consistency between trial training and real-world product use is recommended.
Quality Control of Image Review: Measures should ensure blind review by readers, diverse reader representation, and data blinding. Cross-over designs and appropriate washout periods are recommended for controlled trials. The choice of design should align with the clinical application and product scope.
Non-diagnostic Functions
These functions include structured report generation, image comparison, anatomical structure segmentation, workflow optimization, size measurements, and CT value measurements. They can be assessed for safety and effectiveness through clinical trials or by providing clinical evaluation data. Validation methods may include testing on specific datasets, stress testing, and results from well-qualified databases. Clinical trials can establish secondary endpoints using clinical reference standards or established academic methods. Proper evaluation ensures the reliability and performance of these non-assistive clinical functions in AI-assisted diagnostic devices.
IFU related to Clinical Evaluation
Clinical Trial Summary: This section summarizes the essential information from the clinical trials, including the basic clinical data, evaluation metrics, and results, possibly including subgroup analyses when necessary.
Scope of Application: For AI-assisted diagnostic products, the scope of application should be explicitly defined. This includes specifying the indications for which the product assists in detection (e.g., lung nodules, fractures), the types of images it is based on (e.g., chest CT or colonoscopy images), the product’s other key functions (e.g., image display, processing, measurement, and analysis), and the product’s clinical role (emphasizing that it cannot be solely used for clinical decision-making).
The document also recommends that applicants include the following warning and cautionary notes to ensure the safe and appropriate use of the product:
Physician’s Responsibility: The software serves as an aid to physicians in lesion detection and may produce false positives or false negatives. It is vital for healthcare professionals to consider the patient’s medical history, symptoms, signs, and other diagnostic results when making final decisions about lesion detection and further diagnostic and treatment actions.
Guideline Updates: Users are advised that the product’s design is based on specific guidelines from a certain year (e.g., “Chest CT Lung Nodule Data Labeling and Quality Control Expert Consensus (2018)”). If there are updates to these guidelines, users should carefully assess the potential risks associated with any differences in guidelines when using the product.
Boundary Segmentation Accuracy: The document should make it clear that the product’s clinical trials did not assess the accuracy of lesion boundary segmentation. In cases where a medical decision, such as surgical intervention or biopsy, is based on the software’s detection results, physicians should thoroughly evaluate the associated risks.
The guideline also gives the following examples as an annex:
- SEO Powered Content & PR Distribution. Get Amplified Today.
- PlatoData.Network Vertical Generative Ai. Empower Yourself. Access Here.
- PlatoAiStream. Web3 Intelligence. Knowledge Amplified. Access Here.
- PlatoESG. Carbon, CleanTech, Energy, Environment, Solar, Waste Management. Access Here.
- PlatoHealth. Biotech and Clinical Trials Intelligence. Access Here.
- Source: https://chinameddevice.com/nmpa-ai-assisted-software/
- :is
- :not
- :where
- 2018
- 2023
- 7
- a
- About
- academic
- accuracy
- actions
- advised
- against
- AI
- Aid
- aim
- algorithms
- align
- Alpha
- also
- among
- an
- analyses
- analysis
- and
- Annex
- any
- applicants
- Application
- applications
- applied
- appropriate
- approval
- ARE
- areas
- artificial
- AS
- assess
- assessed
- assessment
- assist
- assists
- associated
- based
- basic
- basis
- BE
- between
- bias
- blind
- by
- CAN
- cannot
- carefully
- case
- cases
- Cautionary
- certain
- characteristics
- charge
- choice
- class
- clear
- click
- Clinical
- clinical trials
- clinically
- collect
- COM
- Common
- comparison
- comparisons
- CONFIRMED
- Consensus
- Consider
- considerations
- considered
- construction
- control
- controlled
- Corresponding
- cover
- criteria
- crucial
- data
- data analysis
- data storage
- databases
- datasets
- decision
- Decision Making
- decisions
- defined
- Design
- designs
- Detection
- determining
- device
- Devices
- diagnostic
- DID
- differences
- Dimension
- Disease
- Display
- diverse
- document
- due
- e
- effect
- effectiveness
- embedded
- emphasizing
- endpoints
- English
- enhancing
- ensure
- ensures
- especially
- essential
- establish
- established
- etc
- evaluate
- evaluation
- examples
- expert
- Explain
- explicitly
- false
- fda
- fee
- final
- Focus
- following
- For
- from
- functions
- further
- generation
- gives
- guidance
- guidelines
- healthcare
- highlighting
- history
- HTTPS
- identifying
- if
- iii
- image
- images
- Imaging
- in
- include
- includes
- Including
- inclusion
- indications
- information
- intended
- interaction
- intervention
- involve
- Issued
- IT
- jpg
- judgments
- Key
- labeling
- levels
- maintaining
- make
- Making
- marking
- May..
- measurement
- measurements
- measures
- medical
- medical devices
- methods
- Metrics
- minimize
- multiple
- must
- necessary
- negatives
- normal
- Notes
- November
- of
- often
- on
- optimization
- Options
- or
- Other
- outlined
- panels
- Parallel
- parameters
- patient
- Pattern
- performance
- periods
- plato
- Plato Data Intelligence
- PlatoData
- please
- points
- population
- possible
- possibly
- potential
- power
- practice
- preferred
- prevalence
- primarily
- primary
- procedures
- processes
- processing
- produce
- Product
- Products
- professionals
- proper
- providing
- qualifications
- quality
- radiology
- Randomized
- Reader
- readers
- real world
- recognition
- recommended
- recommends
- refer
- reference
- refers
- related
- released
- reliability
- report
- representation
- Requirements
- Results
- review
- risks
- robustness
- Role
- safe
- Safety
- scope
- secondary
- Section
- segmentation
- selection
- Sensitivity
- serves
- should
- Signs
- Size
- sizes
- Software
- solely
- specific
- specificity
- specifics
- standalone
- standards
- statistical
- storage
- stress
- structure
- structured
- structures
- Study
- such
- SUMMARY
- surgical
- Symptoms
- Target
- terminology
- Testing
- that
- The
- their
- There.
- These
- they
- this
- thoroughly
- those
- Through
- to
- Training
- Translation
- Transparency
- treatment
- trial
- trials
- types
- ultrasound
- Updates
- usability
- use
- used
- users
- using
- validation
- value
- variations
- vital
- warning
- we
- WELL
- when
- which
- with
- within
- workflow
- year
- zephyrnet