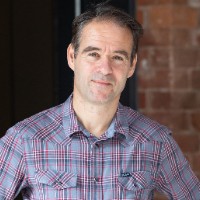
위험 관리가 가장 중요한 금융 서비스 세계에서 우리 모두는 인공 지능과 기계 학습이 환경을 빠르게 변화시키는 것을 목격했습니다. 실제로 최근에는
영란은행과 금융행위감독청(Financial Conduct Authority)의 조사 (FCA)는 이렇게 밝혔다.
영국 금융회사의 72%는 이미 AI/ML 애플리케이션을 사용하거나 개발하고 있습니다., 그리고 이러한 추세는 놀라운 속도로 가속화되고 있습니다.
향후 3.5년 동안 ML 애플리케이션의 평균 수는 XNUMX배로 급증할 것으로 예상됩니다.. This growth is not surprising – AI/ML models hold the promise of unlocking insights from vast amounts of data, enabling financial organisations
to make smarter, more informed decisions, and enhance their risk management strategies.
The survey’s findings are consistent with observations that I’ve made through my work with UK financial services institutions. Although, I have found that the progression towards AI/ML methodologies is more advanced within Fintech and Challenger Banks that,
unlike the High Street Banks, may not suffer from actual limitations due to legacy systems or perceived limitations relating to their IRB status.
Fintechs and Challenger Banks have typically recruited tech-savvy data scientists with deep understandings of the array of alternative advanced techniques that are available. Meanwhile, major banks still hold a significant advantage in terms of experience
and data. They have decades of experience in building credit models, have established model development standards, and have a thorough understanding of the underlying data.
이제 문제는 기존 모델의 개발을 뒷받침하는 원칙이 완전히 다른 방식으로 수학적으로 파생되는 차세대 AI 기반 모델과 전적으로 관련이 있는지 여부입니다.
모델 개발: 기존 VS AI/ML
Traditional scorecard development has long adhered to meticulous sample design, ensuring that the applications during the sample window are both stable and reflective of proposals most recently received. It is typical for Population Stability Indices or Characteristics
Stability Indices to be calculated, and for a detailed investigation of any patterns that extend beyond reasonable expectations of seasonal variation. This approach hinges on the notion of a bespoke development sample tailored to the specific population it
serves. The composition or segmental mix and its specificity is seen as a key factor in the suitability of the model development sample.
Interestingly, we often see that AI/ML models exhibit a significant degree of cross-learning. This is where models display stronger performance when the training sample is extended to include additional observations that might not traditionally be considered
directly relevant. For example, we see superior performance from models trained on an expanded sample window versus equivalent models optimised on a period that simply aligns to the independent test sample. This is unlikely to happen using linear models!
Similar findings can be seen when adjacent segments or groups are added to the training samples. Indeed, AI/ML models thrive when developed upon large and diverse data sets. These phenomena will have implications for sample design and choice of exclusions within
model developments of the future, potentially rewriting conventional wisdom.
Similarly, many credit scorecard developments have incorporated segmentation, whereby a model is built for each of a number of sub-populations (eg. Thin File / Thick File, Clean / Dirty). The benefit of this approach is that, by building multiple models, some
non-linearity can be captured. Of course, the choice of segmentation is not always obvious and is unlikely to be optimal, however some performance uplifts are achieved. Given that AI/ML models are built because of their ability to capture non-linearity, there
is limited need for segmented models here, unless there are fundamental differences in data structure. Therefore, AI/ML models are more complex, fewer of them should be required.
Another area of focus within traditional scorecard development is the process of moving from fine-to-coarse classing. Hereby the modeller seeks to effectively divide continuous data into several ordinal groups so that the underlying bad rate shows a logical
progression and is based on sufficient volume to give a reliable result. Advanced methodologies within AI/ML models eliminate the need for fine-to-coarse classing as the grouping is achieved by the underlying methodology, generating smooth response profiles
rather than the step-changes seen as scorecard attribute boundaries are crossed. Furthermore, many training routines now include the option to add constraints to ensure features have a logical impact on the model predictions.
As the wave of AI/ML model development surges in the coming years, a fusion of deep knowledge of underlying credit data and advanced methodology is key. While new challenges arise in this new generation of models, such as unintended bias and explainability,
historical concerns will become less relevant.
- SEO 기반 콘텐츠 및 PR 배포. 오늘 증폭하십시오.
- PlatoData.Network 수직 생성 Ai. 자신에게 권한을 부여하십시오. 여기에서 액세스하십시오.
- PlatoAiStream. 웹3 인텔리전스. 지식 증폭. 여기에서 액세스하십시오.
- 플라톤ESG. 탄소, 클린테크, 에너지, 환경, 태양광, 폐기물 관리. 여기에서 액세스하십시오.
- PlatoHealth. 생명 공학 및 임상 시험 인텔리전스. 여기에서 액세스하십시오.
- 출처: https://www.finextra.com/blogposting/25517/risk-model-development–the-next-generation?utm_medium=rssfinextra&utm_source=finextrablogs
- :있다
- :이다
- :아니
- :어디
- a
- 능력
- 가속하는
- 달성
- 실제
- 더하다
- 추가
- 추가
- 접착
- 인접
- 많은
- 이점
- AI 기반
- AI / ML
- 정렬
- All
- 이미
- 대안
- 이기는하지만
- 항상
- 금액
- an
- 및
- 어떤
- 어플리케이션
- 접근
- 있군요
- 지역
- 발생
- 배열
- 인조의
- 인공 지능
- 인공 지능과 기계 학습
- AS
- At
- 가능
- 나쁜
- 은행
- 영국 은행
- 은행
- 기반으로
- BE
- 때문에
- 가
- 이익
- 주문품
- 그 너머
- 바이어스
- 두
- 경계
- 건물
- 내장
- by
- 계산 된
- CAN
- 포착
- 캡처
- 도전자
- 도전자 은행
- 과제
- 특성
- 선택
- 황어 무리
- CO
- 오는
- 완전히
- 복잡한
- 구성
- 우려 사항
- 행위
- 고려
- 일관된
- 제약
- 끊임없는
- 전통적인
- 코스
- 신용
- 교차
- 데이터
- 데이터 세트
- 수십 년
- 결정
- 깊은
- 도
- 파생
- 디자인
- 상세한
- 개발
- 개발
- 개발
- 개발
- 차이
- 다른
- 직접
- 디스플레이
- 몇몇의
- 나누다
- 두
- ...동안
- 마다
- 효과적으로
- 제거
- 가능
- 영국
- 강화
- 확인
- 보장
- 동등한
- 확립 된
- 예
- 전시회
- 확대하는
- 기대
- 경험
- 설명 가능성
- 확장
- extended
- 사실
- 인자
- FCA
- 특징
- 적은
- 입양 부모로서의 귀하의 적합성을 결정하기 위해 미국 이민국에
- 금융
- 금융 행위
- 금융 서비스
- 결과
- 파인 트라
- FINTECH
- 기업
- 초점
- 럭셔리
- 발견
- 에
- 기본적인
- 게다가
- 퓨전
- 미래
- 생성
- 세대
- 주기
- 주어진
- 여러 떼
- 성장
- 발생
- 있다
- 여기에서 지금 확인해 보세요.
- 높은
- 경첩
- 역사적인
- 보유
- 그러나
- HTTPS
- i
- 영향
- 의미
- in
- 포함
- Incorporated
- 독립
- 색인
- 정보
- 통찰력
- 기관
- 인텔리전스
- 으로
- 조사
- IT
- 그
- JPG
- 키
- 핵심 요소
- 지식
- 경치
- 넓은
- 배우기
- 유산
- 적게
- 한계
- 제한된
- 논리
- 긴
- 기계
- 기계 학습
- 만든
- 주요한
- 확인
- 구축
- .
- 수학적으로
- XNUMX월..
- 그 동안에
- 방법론
- 방법론
- 세심한
- 수도
- 혼합
- ML
- 모델
- 모델
- 배우기
- 가장
- 움직이는
- 여러
- my
- 필요
- 신제품
- 다음 것
- 개념
- 지금
- 번호
- 관찰
- 분명한
- of
- 자주
- on
- 최적의
- 최적화 됨
- 선택권
- or
- 조직
- 평화
- 최고의
- 패턴
- 감지 된
- 성능
- 기간
- 플라톤
- 플라톤 데이터 인텔리전스
- 플라토데이터
- 인구
- 잠재적으로
- 예측
- 원칙
- 방법
- 프로필
- 진행
- 돌출한
- 약속
- 제안
- 문제
- 빠르게
- 율
- 차라리
- 합리적인
- 접수
- 최근
- 최근에
- 관련된
- 신뢰할 수있는
- 남아
- 필수
- 응답
- 결과
- 공개
- 재 작성
- 위험
- 위험 관리
- 과학자
- 스코어 카드
- 계절의
- 참조
- 탐색
- 본
- 분할
- 세그먼트
- 봉사하다
- 서비스
- 설정
- 몇몇의
- 영상을
- 쇼
- 상당한
- 간단히
- 유성 불꽃
- 똑똑한
- 펴다
- So
- 일부
- 구체적인
- 특성
- 안정
- 안정된
- 기준
- Status
- 아직도
- 전략들
- 거리
- 강한
- 구조
- 이러한
- 충분한
- 적당
- 우수한
- 서지
- 놀라운
- 설문조사
- 시스템은
- 맞춤형
- 기법
- 조건
- test
- 보다
- 그
- XNUMXD덴탈의
- 미래
- 풍경
- 세계
- 그들의
- 그들
- 그곳에.
- 따라서
- Bowman의
- 그들
- 이
- 세
- 사는 보람으로 삼다
- 을 통하여
- 시대
- 에
- 에 대한
- 전통적인
- 전통적으로
- 훈련 된
- 트레이닝
- 변화
- 경향
- 전형적인
- 일반적으로
- Uk
- 밑에 있는
- 지지하다
- 이해
- 같지 않은
- 가능성
- 잠금 해제
- ...에
- 사용
- 거대한
- Ve
- 대
- 음량
- vs
- 웨이브
- 방법..
- we
- 언제
- 여부
- 어느
- 동안
- 전적으로
- 의지
- 창
- 지혜
- 과
- 이내
- 작업
- 세계
- 년
- 제퍼 넷