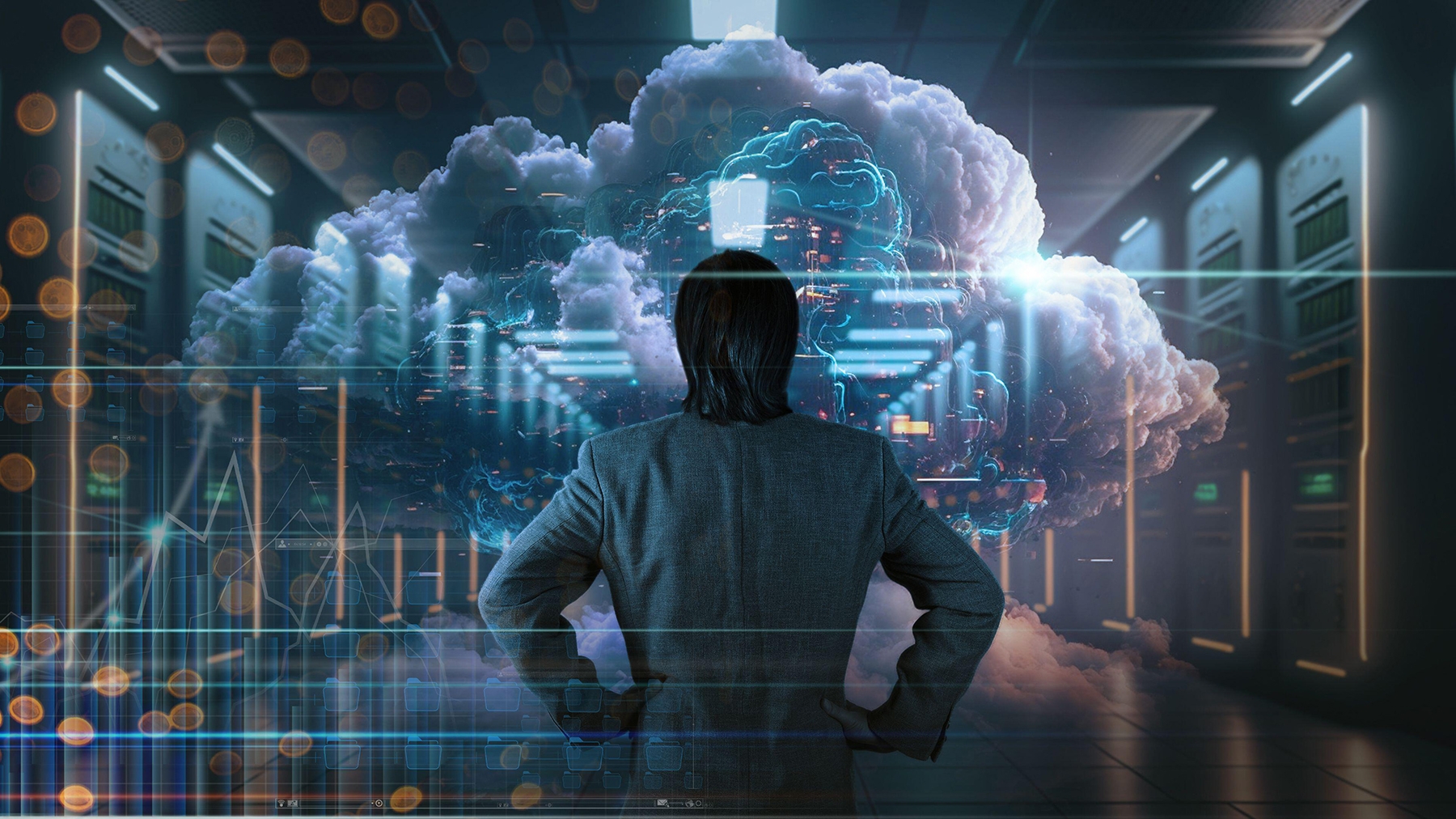
인공지능(AI)은 금융, 제조, 광고, 의료 등 다양한 산업을 변화시키고 있습니다. IDC predicts global spending on AI will exceed $300 billion by 2026. Companies spend millions of dollars building AI models, which are considered priceless intellectual property, and the parameters and model weights are closely guarded secrets. Even knowing some of the parameters in a competitor’s model is considered valuable intelligence.
이러한 모델을 훈련하는 데 사용되는 데이터 세트도 기밀이며 경쟁 우위를 창출할 수 있습니다. 결과적으로 데이터 및 모델 소유자는 이러한 자산을 도난이나 규정 준수 위반으로부터 보호하기 위해 노력하고 있습니다. 기밀성과 무결성을 보장해야 합니다.
이는 우리를 기밀 AI라는 새로운 분야로 안내합니다. 기밀 AI의 목표는 훈련 데이터의 모델 생성, 훈련, 전처리 및 큐레이션과 수명 주기 전반에 걸친 모델 및 데이터의 실행이 정지 상태에서 손상, 변조 및 노출로부터 보호되도록 하는 것입니다. 대중교통, 사용중입니다. 누구로부터 보호받나요? 인프라 제공자, 불량 시스템 관리자, 모델 소유자, 데이터 소유자 및 모델이나 데이터의 중요한 요소를 훔치거나 변경할 수 있는 기타 행위자 등이 포함됩니다. Confidential AI는 강력한 정책 시행을 강조하고 제로 트러스트 원칙.
기밀 AI 사용 사례
Confidential AI에는 다양한 기술과 기능이 필요하며 일부 새로운 기능과 기존 하드웨어 및 소프트웨어의 일부 확장 기능이 필요합니다. 여기에는 다음이 포함됩니다 기밀 컴퓨팅 같은 기술 신뢰할 수 있는 실행 환경(TEE) CPU뿐만 아니라 GPU와 같은 다른 플랫폼 구성 요소에서 사용 중인 데이터를 안전하게 유지하는 데 도움이 되며, CPU 및 GPU TEE에 대한 신뢰 증명을 확인하고 제공하는 데 사용되는 증명 및 정책 서비스도 제공됩니다. 또한 올바른 데이터 세트를 소싱하고, 전처리하고, 정리하고, 레이블을 지정하는 서비스도 포함됩니다. 마지막으로 키 관리, 키 중개 및 배포 서비스는 모델, 데이터, 프롬프트 및 컨텍스트가 TEE 내부에 액세스되거나 실행을 위해 전달되기 전에 암호화되도록 보장합니다.
Let’s look at four of the top confidential AI scenarios.
1. 기밀 추론
이는 기밀 AI의 가장 일반적인 사용 사례입니다. 모델이 훈련되고 배포됩니다. 소비자 또는 클라이언트는 모델과 상호 작용하여 결과를 예측하고, 결과를 생성하고, 통찰력을 얻는 등의 작업을 수행합니다.
모델 소유자와 개발자는 모델이 배포된 인프라, 즉 클라우드 공급자, 서비스 공급자, 심지어 자체 관리자로부터 모델 IP를 보호하기를 원합니다. 이를 위해서는 모델과 데이터가 항상 해당 소유자가 제어하는 키로 암호화되고 사용 시 증명 서비스를 받아야 합니다. 실제 암호 해독 키가 보관되어 있는 키 브로커 서비스는 보안 채널을 통해 암호 해독 키를 TEE에 공개하기 전에 증명 결과를 확인해야 합니다. 그런 다음 추론이 발생하기 전에 모델과 데이터가 TEE 내부에서 해독됩니다.
이 사용 사례의 다양한 변형이 가능합니다. 예를 들어 추론 데이터는 TEE로 직접 스트리밍되는 실시간 데이터로 암호화될 수 있습니다. 또는 생성 AI의 경우 모델이 작동 중일 때 사용자의 프롬프트와 컨텍스트가 TEE 내부에서만 표시됩니다. 마지막으로 추론의 결과는 암호화가 필요할 수도 있고 필요하지 않을 수도 있는 요약 정보일 수 있습니다. 출력은 시각화 또는 모니터링 환경으로 다운스트림으로 공급될 수도 있습니다.
2. 기밀 교육
Before any models are available for inferencing, they must be created and then trained over significant amounts of data. For most scenarios, model training requires massive amounts of compute power, memory, and storage. A cloud infrastructure is well-suited for this, but it requires strong security guarantees for data at rest, in transit, and in use. The requirements presented for confidential inferencing also apply to confidential training, to provide evidence to the model builder and the data owner that the model (including the parameters, weights, checkpoint data, etc.) and the training data aren’t visible outside the TEEs.
An often-stated requirement about confidential AI is, “I want to train the model in the cloud, but would like to deploy it to the edge with the same level of security. No one other than the model owner should see the model.” The approach presented for confidential training and confidential inference work in tandem to accomplish this. Once the training is done, the updated model is encrypted inside the TEE with the same key that was used to decrypt it before the training process, the one belonging to the model owner’s.
This encrypted model is then deployed, along with the AI inference application, to the edge infrastructure into a TEE. Realistically, it’s downloaded from the cloud to the model owner, and then it is deployed with the AI inferencing application to the edge. It follows the same workflow as confidential inference, and the decryption key is delivered to the TEEs by the key broker service at the model owner, after verifying the attestation reports of the edge TEEs.
3. 연합 학습
This technique provides an alternative to a centralized training architecture, where the data is not moved and aggregated from its sources due to security and privacy concerns, data residency requirements, size and volume challenges, and more. Instead, the model moves to the data, where it follows a precertified and accepted process for distributed training. The data is housed in the client’s infrastructure, and the model moves to all the clients for training; a central governor/aggregator (housed by the model owner) collects the model changes from each of the clients, aggregates them, and generates a new updated model version.
여기서 모델 소유자의 가장 큰 관심사는 모델이 훈련되는 클라이언트 인프라에서 모델 IP가 손상될 수 있다는 것입니다. 마찬가지로 데이터 소유자는 모델 작성자/소유자가 모델 그라데이션 업데이트를 볼 수 있는지 걱정하는 경우가 많습니다. 연합 학습과 기밀 컴퓨팅을 결합하면 더 강력한 보안과 개인정보 보호가 보장되고 제로 트러스트 아키텍처가 가능해집니다.
Doing this requires that machine learning models be securely deployed to various clients from the central governor. This means the model is closer to data sets for training, the infrastructure is not trusted, and models are trained in TEE to help ensure data privacy and protect IP. Next, an attestation service is layered on that verifies TEE trustworthiness of each client’s infrastructure and confirms that the TEE environments can be trusted where the model is trained. Finally, trained models are sent back to the aggregator or governor from different clients. Model aggregation happens inside the TEEs, the model is updated and processes repeatedly until stable, and then the final model is used for inference.
4. 기밀 튜닝
AI에 대한 새로운 시나리오는 기업이 일반적인 AI 모델을 취하고 일반적으로 조직에 비공개인 비즈니스 도메인별 데이터를 사용하여 이를 조정하려는 것입니다. 주요 근거는 일련의 도메인별 작업에 대한 모델의 정밀도를 미세 조정하고 향상시키는 것입니다. 예를 들어, IT 지원 및 서비스 관리 회사는 기존 LLM을 활용하여 IT 지원 및 헬프데스크 관련 데이터로 교육을 원할 수도 있고, 금융 회사는 독점 재무 데이터를 사용하여 기본 LLM을 세부 조정할 수도 있습니다.
This fine-tuning most likely would require an external cloud infrastructure, given the huge demands on compute, memory, and storage. A confidential training architecture can help protect the organization’s confidential and proprietary data, as well as the model that’s tuned with that proprietary data.
- SEO 기반 콘텐츠 및 PR 배포. 오늘 증폭하십시오.
- PlatoData.Network 수직 생성 Ai. 자신에게 권한을 부여하십시오. 여기에서 액세스하십시오.
- PlatoAiStream. 웹3 인텔리전스. 지식 증폭. 여기에서 액세스하십시오.
- 플라톤ESG. 탄소, 클린테크, 에너지, 환경, 태양광, 폐기물 관리. 여기에서 액세스하십시오.
- PlatoHealth. 생명 공학 및 임상 시험 인텔리전스. 여기에서 액세스하십시오.
- 출처: https://www.darkreading.com/cloud-security/confidential-ai-protect-data-models-across-cloud
- :이다
- :아니
- :어디
- 2026
- a
- 소개
- 접수
- 액세스
- 달성
- 가로질러
- 배우
- 실제
- 관리자
- 이점
- 광고
- 후
- 집합
- 애그리 게이터 (aggregator)
- AI
- AI 모델
- All
- 따라
- 또한
- 대안
- 항상
- 금액
- an
- 및
- 어떤
- 어플리케이션
- 신청
- 접근
- 아키텍처
- 있군요
- AS
- 자산
- At
- 가능
- 뒤로
- BE
- 전에
- 존재
- 귀속
- 큰
- 억원
- 돋보이게
- 브로커
- 건축업자
- 건물
- 사업
- 비자 면제 프로그램에 해당하는 국가의 시민권을 가지고 있지만
- by
- CAN
- 기능
- 케이스
- 가지 경우
- 중심적인
- 중앙
- 과제
- 변경
- 채널
- 클라이언트
- 클라이언트
- 면밀히
- 자세히
- 클라우드
- 클라우드 인프라
- 수집하다
- 결합
- 기업
- 회사
- 경쟁력
- 경쟁자
- compliance
- 구성 요소들
- 타협
- 계산
- 컴퓨팅
- 관심
- 우려 사항
- 기밀 유지
- 고려
- 소비자
- 문맥
- 통제
- 수
- CPU
- 만들
- 만든
- 창조
- 임계
- 큐 레이션
- 주기
- 데이터
- 데이터 프라이버시
- 데이터 세트
- 해독
- 전달
- 요구
- 배포
- 배포
- 드리프트
- 개발자
- 다른
- 직접
- 분산
- 분산 교육
- 분포
- 달러
- 한
- 두
- 마다
- Edge
- 요소
- 신흥
- 강조하다
- 수
- 암호화
- 암호화
- 시행
- 확인
- 환경
- 환경
- 등
- 에테르 (ETH)
- 조차
- 증거
- 예
- 넘다
- 실행
- 현존하는
- 노출 시간
- 확장
- 외부
- 연방 준비 은행
- 들
- 최후의
- 최종적으로
- 재원
- 금융
- 재무 데이터
- 다음
- 럭셔리
- 기초
- 사
- 에
- 생성
- 생성
- 생성적인
- 제너레이티브 AI
- 점점
- 주어진
- 글로벌
- 골
- 지사
- GPU
- GPU
- 보증
- 발생
- 하드웨어
- 건강 관리
- 도움
- 여기에서 지금 확인해 보세요.
- 고도로
- HTTPS
- 거대한
- i
- IDC
- 개선
- in
- 포함
- 포함
- 산업
- 정보
- 인프라
- 내부
- 통찰력
- 를 받아야 하는 미국 여행자
- 보전
- 지적인
- 지적 재산권
- 인텔리전스
- 상호 작용하는
- 으로
- IP
- IT
- IT 지원
- 그
- JPG
- 다만
- 유지
- 키
- 키
- 아는
- 성
- 계층
- 배우기
- 레벨
- 생활
- 처럼
- 아마도
- 보기
- 찾고
- 기계
- 기계 학습
- 구축
- 제조
- 거대한
- XNUMX월..
- 방법
- 메모리
- 수도
- 수백만
- 모델
- 모델
- 모니터링
- 배우기
- 가장
- 움직이는
- 이동
- 절대로 필요한 것
- 필요
- 신제품
- 다음 것
- 아니
- of
- 자주
- on
- 일단
- ONE
- 만
- 운영
- or
- 조직
- 기타
- 결과
- 출력
- 외부
- 위에
- 자신의
- 소유자
- 소유자
- 매개 변수
- 플랫폼
- 플라톤
- 플라톤 데이터 인텔리전스
- 플라토데이터
- 정책
- 가능한
- 가능성
- 힘
- Precision
- 예측
- 예측
- 제시
- 아주 재미있는
- 일차
- 개인 정보 보호
- 사설
- 방법
- 프로세스
- 프롬프트
- 증명
- 재산
- 소유권
- 보호
- 보호
- 보호
- 제공
- 제공
- 제공
- 이론적 해석
- 실시간
- 실시간 데이터
- 발매
- 자꾸
- 보고서
- 필요
- 요구 사항
- 요구조건 니즈
- 필요
- 그
- REST
- 결과
- 결과
- 연락해주세요
- s
- 가장 안전한 따뜻함
- 같은
- 대본
- 시나리오
- 비밀
- 안전해야합니다.
- 안전하게
- 보안
- 참조
- 전송
- 서비스
- 서비스 제공자
- 서비스
- 세트
- 설정
- 영상을
- 상당한
- 비슷하게
- 크기
- 소프트웨어
- 일부
- 소싱
- 지우면 좋을거같음 . SM
- 지출
- 지출
- 안정된
- 저장
- 스트리밍
- 강한
- 강한
- 이러한
- SUPPORT
- 체계
- T
- 받아
- 자전거
- 작업
- 기술
- 기술
- 보다
- 그
- XNUMXD덴탈의
- 절도
- 그들의
- 그들
- 그때
- Bowman의
- 그들
- 이
- 을 통하여
- 에
- 상단
- Train
- 훈련 된
- 트레이닝
- 변화
- 운송
- 믿어
- 신뢰할 수있는
- 신뢰성
- 전형적인
- 일반적으로
- 까지
- 업데이트
- 업데이트
- ...에
- us
- 사용
- 유스 케이스
- 익숙한
- 사용자
- 사용
- 독점 사용
- 가치 있는
- 변화
- 종류
- 여러
- 확인
- 확인하는
- 버전
- 위반
- 가시성
- 눈에 보이는
- 심상
- 음량
- 필요
- 였다
- 잘
- 언제
- 어느
- 동안
- 누구
- 누구에게
- 의지
- 과
- 작업
- 워크플로우
- 겠지
- 제퍼 넷