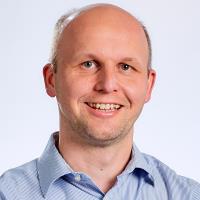
Last year I celebrated two decades of immersion in IT, specifically within the Financial Services sector. During this period I have been a witness to remarkable transformations in banking and technology. The emergence of Fintech companies and their customer-centric
approach, along with significant advancements in software engineering like Agile methodologies, microservices, and cloud computing, have reshaped the landscape. Yet, intriguingly, the back-office operations of many financial service companies have remained
relatively static over these years, still grappling with manuell kodning, repetitiva uppgifter och starkt beroende av Excel.
En särskilt manuell och ändå automatiserbar process inom finanssektorn är matchning och avstämning. This process arises in various forms, i.e. from identifying and addressing discrepancies (typically occurring due to issues
or gaps with the integrations) in master-slave integrations to correcting or removing duplicates and semi-automated updates of operational systems with data from external sources.
Trots tillgången till sofistikerad programvara (e.g. FIS IntelliMatch, Calypso Confirmation Matching, Misys CMS, Temenos T24 Confirmation Matching…) for specific reconciliation tasks, such as payment and trade confirmation matching
(often based on SWIFT messages), the majoriteten av matchande uppgifter är ofta beroende av anpassade eller manuella lösningar, including Excel or even paper-based methods. Very often automation is also not pertinent, as matching is often involved in one-time actions
like marketing campaigns, data clean-ups, alignment with partners…
Förstå bättre avstämning kräver dissekera dess komponenter, Det vill säga
-
Det börjar med samla in och omvandla de olika datamängderna för jämförbarhet. This consists of recuperating 2 data sets, which can be delivered in different formats, different structures, different scopes and with different names
or enumerations. The data needs to be transformed to make them comparable and loaded into the same tool (e.g. a database or Excel), so that they can be easily compared. -
Nästa steg är att definiera en exakt matchningsalgoritm. This can be a simple unique key, but it can also a combination of multiple attributes (composite key), a hierarchical rule (i.e. match first on key 1, if no match try on key 2…) or
a fuzzy rule (if key of data set 1 resembles key of data set 2 it is a match). Defining this matching algorithm can be very complex, but it is crucial in the ability to automate the matching and reach a good output quality. -
När matchningsalgoritmen är definierad anger vi jämförelsefas. For small data sets, this can be done quite simple, but for very large data sets, it can necessitate all kinds of performance optimizations (like indices, segmentation,
parallelism…) in order to execute the comparison in a reasonable time. -
Slutligen identifierade avvikelser måste översättas till handlingsbara resultat, såsom rapporter, kommunikation till kollegor eller tredje part eller korrigerande åtgärder (t.ex. generering av filer, meddelanden eller SQL-satser för att åtgärda skillnaderna).
Inveckladigheterna med matchning inom finansiella tjänster är olika. Låt oss utforska några typiska användningsfall i landskapet för finansiella tjänster:
-
De flesta banker har en Värdepappers huvudfil, describing all securities which are in position or can be traded at the bank. This file needs to be integrated with a lot of applications, but also needs to be fed by multiple data sources, like
Telekurs, Reuters, Bloomberg, Moody’s… This means a security needs to be uniquely matched. Unfortunately, there is not 1 unique identifier describing all securities. Publicly traded instruments have a commonly agreed ISIN code, but private and OTC products
like e.g. most derivatives usually do not. Banks have therefore invented internal identifiers, use fake ISIN codes (typically starting with an “X”) or use composite keys to uniquely identify the instrument (e.g. for a derivative this can be combination of
ticker of underlying security, strike price, option type and expiration date). -
Inom Retail banking är det självklart viktigt att unikt identifiera och matcha en specifik fysisk person. However even in a developed country like Belgium, this is easier said than done. Every individual in Belgium has a National Register Number,
so this seems the obvious choice for a matching key. Unfortunately, Belgian laws restrict the usage of this number to specific use cases. Additionally this identifier is not existing for foreigners and can change over time (e.g. foreign residents receive first
a temporary National Register number which can change to a definitive, other one later or in case of gender change the National Register Number will change as well). Another option is to use the identity card number, but this is also different for foreigners
and will change every 10 years. Many banks therefore use more complex rules, like a matching based on first name, last name and birth date, but obviously this comes also with all kinds of issues, like duplicates, spelling differences and errors in the names,
use of special characters in the names… -
Ett mycket liknande problem är matcha ett företag eller mer specifikt en butik. In Belgium, each company has a company number, which is similar to the VAT number (without the “BE” prefix), but this is again very national and 1 VAT number can
have multiple locations (e.g. multiple stores). There exists a concept of a “branch number” (“vestigingsnummer” in Dutch), but this concept is not very well known and rarely used. Similar there exists the LEI code (Legal Entity Identifier) which is a code
of a combination of 20 letters and codes, which uniquely identifies a company worldwide. Unfortunately, only large companies have requested a LEI code, so for smaller companies this is not really an option.
Again more complex matchings are often done, like a combination of VAT number, postal code and house number, but obviously this is far from being ideal. In search for a unique and commonly known identifier, the Google ID becomes also more and more in use, but
the dependency with a commercial company might also poses a big operational risk. -
Ett annat intressant fall är matchning av en behörighet och clearingmeddelandet i en VISA-kortbetalning. Normally a unique identifier should match both messages, but due to all kinds of exception cases (e.g. offline authorizations or
incremental authorizations), this will not always be correct. Therefore a more complex rule is required, looking at several identifiers, but also to other matching criteria like acquirer ID, merchant ID, terminal ID, PAN (card number), timestamp and/or amount.
Denna typ av matchning gäller även för andra betalningsanvändningsfall, som t.ex. matchning av ett förauktorisationsuppfyllande med dess föregående förauktorisation eller en återbetalning vid ett tidigare köp. -
Ett ekonomiskt användningsfall som berör nästan alla företag är matchning av faktura och betalning. When a company issues an invoice, it needs to be able to see when the invoice can be considered as paid. This is important for the accounting, but also
to see if reminders for unpaid invoices should be sent out.
To uniquely match the payment with the invoice, in Belgium typically a structured comment is used in the payment instruction. This unique code with check digit provides a unique matching reference. Unfortunately, customers often forget to put the structured
comment or use the wrong one (e.g. copy/paste of a previous invoice). This means a company needs to have a fallback matching rule in case the unstructured comment is missing or wrong. Typically a combination of payment amount, payment date, IBAN of counterparty
and/or name of counterparty can give an alternative way to match those invoices.
Som du kan se är matchning långt ifrån lätt, men att förstå de grundläggande stegen kan hjälpa till med bättre matchningar. Under tiden, trots sina begränsningar, förblir Excel ett kraftfullt verktyg för (manuell) matchning. Därför a quick reminder for everyone who wants
to do matching in Excel:
-
Använda VLOOKUP för att utföra matchning. VLOOKUP har dock vissa begränsningar, som att det ger ett fel om det inte finns någon matchning och att du bara kan söka i den första kolumnen. Ett kraftfullt alternativ är att använda XLOOKUP, Vilket
does not have these limitations. -
Om du behöver en sammansatt söknyckel, lägg till en kolumn i din sökdatauppsättning, med den sammansatta söknyckeln (d.v.s. sammanfoga de olika attributen, med t.ex. "#" som avgränsare) och använd sedan VLOOKUP/XLOOKUP för att söka i denna nya kolumn.
-
Några uppmärksamhetspunkter när du använder VLOOKUP:
-
Glöm inte att lägga till "false" som det sista argumentet för funktionen VLOOKUP för att säkerställa en exakt matchning.
-
Ensure that data formats are the same. E.g. a number “123” and the text “123” will not match, so it is important to convert them to the same format first. Idem for identifiers starting with leading 0’s. Often Excel will convert those to numbers, thus removing
the leading 0’s and not resulting in a match. -
Använd inte datamängder med fler än 100.000 XNUMX rader i Excel. Större datamängder är problematiska för prestanda och stabilitet i Excel.
Det kan också vara intressant att sätta beräkningsläge till "Manuell" om du arbetar med VLOOKUP på stora datamängder, annars kommer Excel att räkna om alla VLOOKUPs varje gång du gör en mindre ändring av datan. -
VLOOKUP har kolumnnumret att returnera som tredje argument. Detta nummer anpassas inte dynamiskt när du lägger till eller tar bort kolumner, så kom ihåg att anpassa när du lägger till eller tar bort kolumner.
-
Om du bara vill ha en matchning kan du använda formeln "=OM(FEL(VLOOKUP(,,1,falskt),,"INGEN MATCH","MATCH")"
-
Dessa knep kan hjälpa till snabba upp dina manuella matchningar, men uppenbarligen är verklig automatisering alltid bättre.
Matchning inom finansiella tjänster är en mångfacetterad utmaning, but understanding its fundamental steps is key to improving outcomes. While tools like Excel offer temporary solutions, the future lies in intelligent automation, which can significantly
streamline these processes. For those seeking to delve deeper into matching complexities or automation, leveraging advanced tools and platforms, including AI-driven solutions like ChatGPT, can provide both insights and practical solutions.
Kolla in alla mina bloggar på https://bankloch.blogspot.com/
- SEO-drivet innehåll och PR-distribution. Bli förstärkt idag.
- PlatoData.Network Vertical Generative Ai. Styrka dig själv. Tillgång här.
- PlatoAiStream. Web3 Intelligence. Kunskap förstärkt. Tillgång här.
- Platoesg. Kol, CleanTech, Energi, Miljö, Sol, Avfallshantering. Tillgång här.
- PlatoHealth. Biotech och kliniska prövningar Intelligence. Tillgång här.
- Källa: https://www.finextra.com/blogposting/25297/the-unseen-backbone-of-banking-a-deep-dive-into-matching-and-reconciliation?utm_medium=rssfinextra&utm_source=finextrablogs
- : har
- :är
- :inte
- $UPP
- 000
- 1
- 10
- 100
- 11
- 13
- 14
- 15%
- 20
- 8
- a
- förmåga
- Able
- Redovisning
- åtgärder
- anpassa
- anpassat
- lägga till
- tillsats
- Dessutom
- adresse
- avancerat
- framsteg
- igen
- smidig
- algoritm
- uppriktning
- Alla
- nästan
- längs
- också
- alternativ
- alltid
- mängd
- an
- och
- Annan
- vilken som helst
- tillämpningar
- applicerar
- tillvägagångssätt
- ÄR
- Argumentet
- AS
- At
- attribut
- tillstånd
- automatisera
- Automation
- tillgänglighet
- Backbone
- Bank
- Banking
- Banker
- baserat
- grundläggande
- BE
- blir
- varit
- Där vi får lov att vara utan att konstant prestera,
- Belgien
- Bättre
- Stor
- födelse
- bloggar
- Direkt
- båda
- Branch
- företag
- men
- by
- beräkning
- Kampanjer
- KAN
- kortet
- Vid
- fall
- firade
- vissa
- byta
- tecken
- ChatGPT
- ta
- val
- Rensa
- cloud
- cloud computing
- cms
- koda
- koder
- kollegor
- Kolumn
- Kolonner
- kombination
- kommer
- kommentar
- kommersiella
- vanligen
- Trygghet i vårdförloppet
- Företag
- företag
- jämförbar
- jämfört
- jämförelse
- fullbordan
- komplex
- komplexiteter
- databehandling
- begrepp
- oro
- bekräftelse
- anses
- består
- konvertera
- korrekt
- motpart
- land
- kriterier
- avgörande
- beställnings
- Kunder
- datum
- datauppsättning
- datauppsättningar
- Databas
- Datum
- årtionden
- djup
- djupdykning
- djupare
- definierade
- definierande
- slutgiltig
- levereras
- gräva
- Dependency
- derivat
- Derivat
- beskriver
- Trots
- utvecklade
- skillnader
- olika
- Siffra
- disparat
- Dyk
- flera
- do
- gör
- gjort
- grund
- dubbletter
- under
- Dutch
- dynamiskt
- e
- varje
- lättare
- lätt
- lätt
- uppkomst
- kodning
- Teknik
- säkerställa
- ange
- enhet
- fel
- fel
- väsentlig
- Även
- Varje
- alla
- excel
- undantag
- exekvera
- befintliga
- finns
- utgångs
- extern
- Faktum
- fejka
- falsk
- långt
- Fed
- Fil
- Filer
- finansiella
- finansiell tjänst
- finansiella tjänster
- Finextra
- fintech
- Fintech-företag
- Förnamn
- FIS
- Fast
- För
- utländska
- format
- former
- formeln
- från
- fungera
- grundläggande
- framtida
- luckor
- Kön
- generering
- Ge
- ger
- god
- kämpar
- Har
- tung
- hjälpa
- Huset
- Men
- HTTPS
- i
- ID
- idealisk
- identifierare
- identifierare
- identifierar
- identifiera
- identifiera
- Identitet
- if
- nedsänkning
- med Esport
- förbättra
- in
- Inklusive
- steg
- index
- individuellt
- insikter
- Instrumentet
- instrument
- integrerade
- integrationer
- Intelligent
- Intelligent automatisering
- intressant
- inre
- in
- intrikat
- uppfann
- faktura
- fakturor
- involverade
- ÄR I
- problem
- IT
- DESS
- jpg
- bara
- Nyckel
- nycklar
- Snäll
- känd
- liggande
- Large
- större
- Efternamn
- senare
- Lagar
- ledande
- Adress
- juridisk enhet
- Låt
- hävstångs
- ligger
- tycka om
- begränsningar
- platser
- du letar
- Lot
- göra
- manuell
- många
- Marknadsföring
- Marknadsföringskampanjer
- Master
- Match
- matchas
- matchande
- betyder
- under tiden
- Merchant
- meddelande
- meddelanden
- metoder
- metoder
- microservices
- kanske
- mindre
- saknas
- Mode
- mer
- mest
- multipel
- måste
- my
- namn
- namn
- nationell
- Behöver
- behov
- Nya
- Nästa
- Nej
- normalt
- antal
- nummer
- Uppenbara
- förekommande
- of
- erbjudanden
- offline
- Ofta
- on
- ONE
- endast
- operativa
- Verksamhet
- Alternativet
- or
- beställa
- OTC
- Övriga
- annat
- ut
- utfall
- produktion
- över
- betalas
- PANORERA
- pappersbaserad
- särskilt
- parter
- betalning
- utföra
- prestanda
- perioden
- fysisk
- Plattformar
- plato
- Platon Data Intelligence
- PlatonData
- utgör
- placera
- post
- den mäktigaste
- Praktisk
- föregående
- pris
- Innan
- privat
- Problem
- process
- processer
- Produkter
- ge
- ger
- publicly
- inköp
- sätta
- kvalitet
- ganska
- sällan
- nå
- verklig
- verkligen
- rimlig
- motta
- avstämning
- referens
- återbetala
- registrera
- relativt
- tillit
- förlita
- förblev
- resterna
- anmärkningsvärd
- ihåg
- påminnelse
- bort
- repetitiva
- Rapport
- begärda
- Obligatorisk
- Liknar
- invånare
- begränsa
- resulterande
- detaljhandeln
- Detaljhandel
- avkastning
- Reuters
- Risk
- Regel
- regler
- Nämnda
- Samma
- Sök
- sektor
- Värdepapper
- säkerhet
- se
- söker
- verkar
- segmentering
- skickas
- service
- Tjänster
- in
- uppsättningar
- flera
- skall
- signifikant
- signifikant
- liknande
- Enkelt
- Small
- mindre
- So
- Mjukvara
- mjukvaruutveckling
- Lösningar
- Källor
- speciell
- specifik
- specifikt
- stavning
- SQL
- Stabilitet
- Starta
- startar
- uttalanden
- Steg
- Steg
- Fortfarande
- lagrar
- effektivisera
- strejka
- strukturerade
- strukturer
- sådana
- SWIFT
- System
- uppgifter
- Teknologi
- Temenos
- temporär
- terminal
- text
- än
- den där
- Smakämnen
- Framtiden
- Landskapet
- deras
- Dem
- sedan
- Där.
- därför
- Dessa
- de
- Tredje
- utomstående
- detta
- de
- Således
- ticker
- tid
- tidsstämpel
- till
- verktyg
- verktyg
- handla
- handlas
- transformationer
- transformerad
- omvandla
- prova
- två
- Typ
- typisk
- typiskt
- underliggande
- förståelse
- tyvärr
- unika
- unikt
- Uppdateringar
- us
- Användning
- användning
- användningsfall
- Begagnade
- med hjälp av
- vanligen
- olika
- MOMS
- mycket
- visum
- Visa kort
- vill
- vill
- Sätt..
- we
- VÄL
- när
- som
- medan
- VEM
- kommer
- med
- inom
- utan
- Vittne
- arbetssätt
- inom hela sverige
- Fel
- X
- år
- år
- ännu
- dig
- Din
- zephyrnet