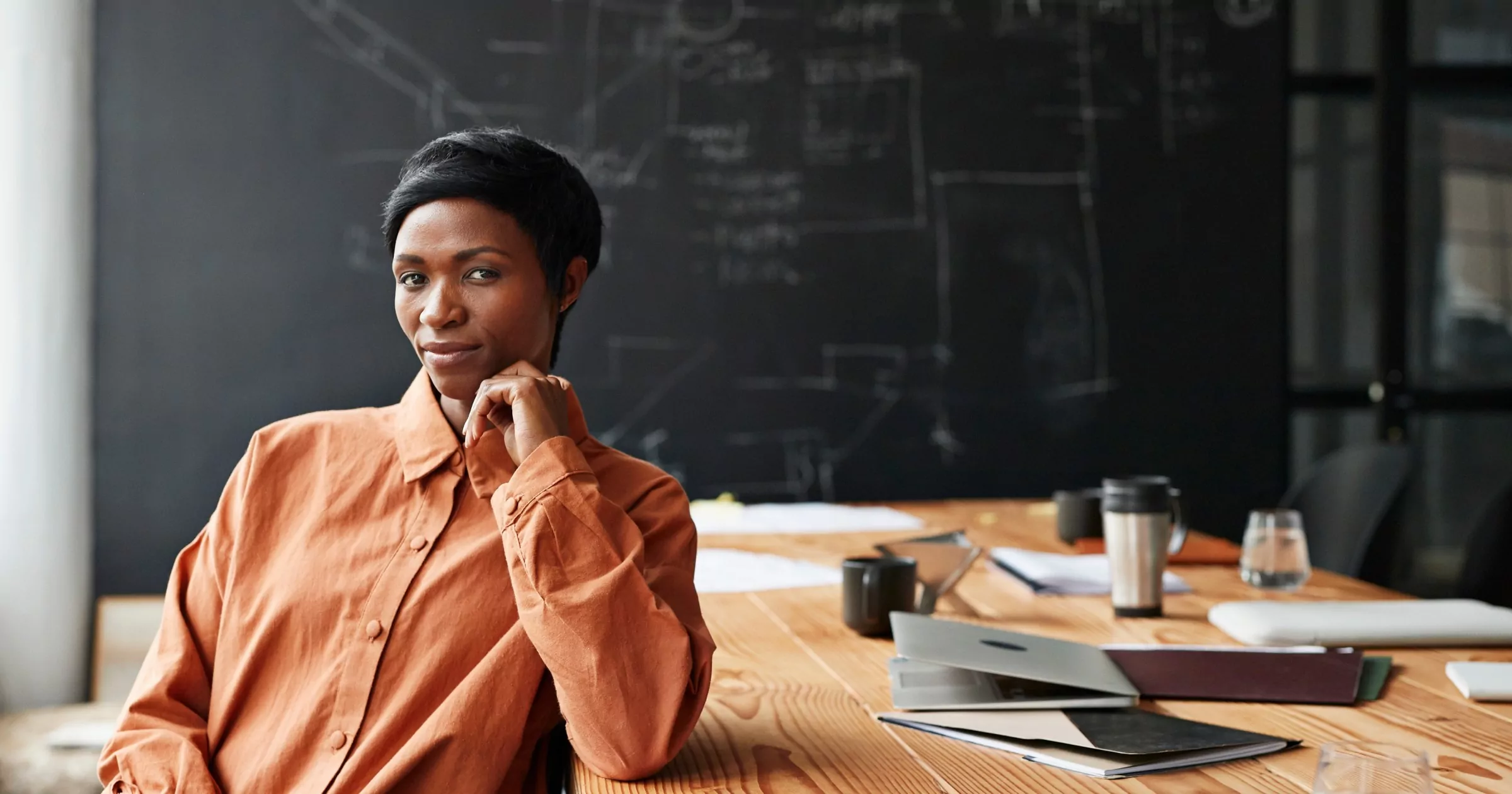
We all want to see our ideal human values reflected in our technologies. We expect technologies such as artificial intelligence (AI) to not lie to us, to not discriminate, and to be safe for us and our children to use. Yet many AI creators are currently facing backlash for the biases, inaccuracies and problematic data practices being exposed in their models. These issues require more than a technical, algorithmic or AI-based solution. In reality, a holistic, socio-technical approach is required.
The math demonstrates a powerful truth
All predictive models, including AI, are more accurate when they incorporate diverse human intelligence and experience. This is not an opinion; it has empirical validity. Consider the diversity prediction theorem. Simply put, when the diversity in a group is large, the error of the crowd is small — supporting the concept of “the wisdom of the crowd.” In an influential study, it was shown that diverse groups of low-ability problem solvers can outperform groups of high-ability problem solvers (Hong & Page, 2004).
In mathematical language: the wider your variance, the more standard your mean. Ecuația arată astfel:
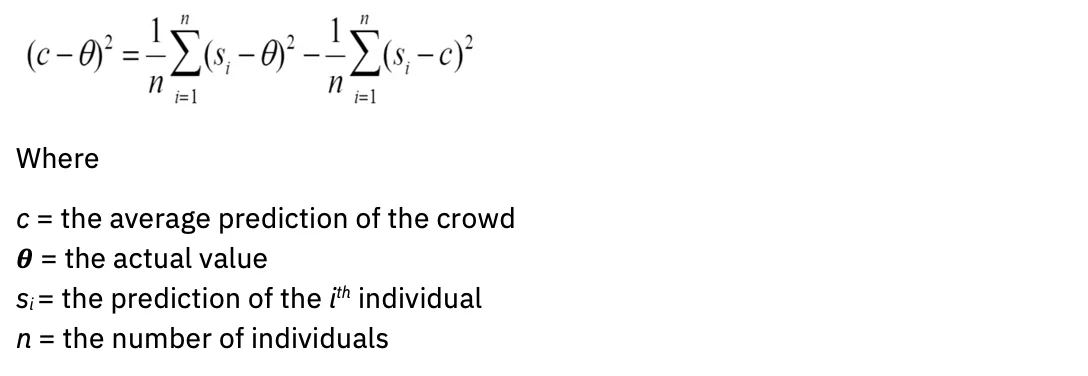
A continuarea studiilor provided more calculations that refine the statistical definitions of a wise crowd, including ignorance of other members’ predictions and inclusion of those with maximally different (negatively correlated) predictions or judgements. So, it’s not just volume, but diversity that improves predictions. How might this insight affect evaluation of AI models?
Model (in)accuracy
To quote a common aphorism, all models are wrong. This holds true in the areas of statistics, science and AI. Models created with a lack of domain expertise can lead to eronat ieșiri.
Today, a tiny homogeneous group of people determine what data to use to train generative AI models, which is drawn from sources that greatly overrepresent English. “For most of the over 6,000 languages in the world, the text data available is not enough to train a large-scale foundation model” (from “On the Opportunities and Risks of Foundation Models,” Bommasani et al., 2022).
Additionally, the models themselves are created from limited architectures: “Almost all state-of-the-art NLP models are now adapted from one of a few foundation models, such as BERT, RoBERTa, BART, T5, etc. While this homogenization produces extremely high leverage (any improvements in the foundation models can lead to immediate benefits across all of NLP), it is also a liability; all AI systems might inherit the same problematic biases of a few foundation models (Bommasani et al.) "
For generative AI to better reflect the diverse communities it serves, a far wider variety of human beings’ data must be represented in models.
Evaluating model accuracy goes hand-in-hand with evaluating bias. We must ask, what is the intent of the model and for whom is it optimized? Consider, for example, who benefits most from content-recommendation algorithms and search engine algorithms. Stakeholders may have widely different interests and goals. Algorithms and models require targets or proxies for Bayes error: the minimum error that a model must improve upon. This proxy is often a person, such as a subject matter expert with domain expertise.
A very human challenge: Assessing risk before model procurement or development
Emerging AI regulations and action plans are increasingly underscoring the importance of algorithmic impact assessment forms. The goal of these forms is to capture critical information about AI models so that governance teams can assess and address their risks before deploying them. Typical questions include:
- What is your model’s use case?
- What are the risks for disparate impact?
- How are you assessing fairness?
- How are you making your model explainable?
Though designed with good intentions, the issue is that most AI model owners do not understand how to evaluate the risks for their use case. A common refrain might be, “How could my model be unfair if it is not gathering personally identifiable information (PII)?” Consequently, the forms are rarely completed with the thoughtfulness necessary for governance systems to accurately flag risk factors.
Thus, the socio-technical nature of the solution is underscored. A model owner—an individual—cannot simply be given a list of checkboxes to evaluate whether their use case will cause harm. Instead, what is required is groups of people with widely varying lived-world experiences coming together in communities that offer psychological safety to have difficult conversations about disparate impact.
Welcoming broader perspectives for trustworthy AI
IBM® believes in taking a “client zero” approach, implementing the recommendations and systems it would make for its own clients across consulting and product-led solutions. This approach extends to ethical practices, which is why IBM created a Trustworthy AI Center of Excellence (COE).
As explained above, diversity of experiences and skillsets is critical to properly evaluate the impacts of AI. But the prospect of participating in a Center of Excellence could be intimidating in a company bursting with AI innovators, experts and distinguished engineers, so cultivating a community of psychological safety is needed. IBM communicates this clearly by saying, “Interested in AI? Interested in AI ethics? You have a seat at this table.”
The COE offers training in AI ethics to practitioners at every level. Both synchronous learning (teacher and students in class settings) and asynchronous (self-guided) programs are offered.
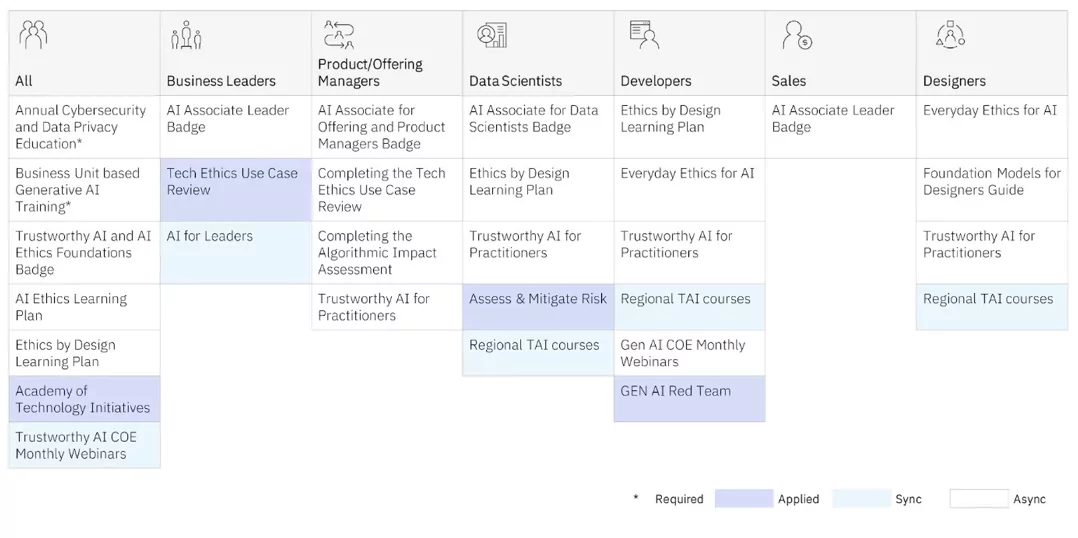
But it’s the COE’s aplicat training that gives our practitioners the deepest insights, as they work with global, diverse, multidisciplinary teams on real projects to better understand disparate impact. They also leverage design thinking frameworks that IBM’s Design pentru AI group uses internally and with clients to assess the unintended effects of AI models, keeping those who are often marginalized top of mind. (See Sylvia Duckworth’s Wheel of Power and Privilege for examples of how personal characteristics intersect to privilege or marginalize people.) IBM also donated many of the frameworks to the open-source community Design Ethically.
Below are a few of the reports IBM have publicly published on these projects:
Automated AI model governance tools are required to glean important insights about how your AI model is performing. But note, capturing risk well before your model has been developed and is in production is optimal. By creating communities of diverse, multidisciplinary practitioners that offer a safe space for people to have tough conversations about disparate impact, you can begin your journey to operationalizing your principles and develop AI responsibly.
In practice, when you are hiring for AI practitioners, consider that well over 70% of the effort in creating models is curating the right data. You want to hire people who know how to gather data that is representative and but that is also gathered with consent. You also want people who know to work closely with domain experts to make certain that they have the correct approach. Ensuring these practitioners have the emotional intelligence to approach the challenge of responsibly curating AI with humility and discernment is key. We must be intentional about learning how to recognize how and when AI systems can exacerbate inequity just as much as they can augment human intelligence.
Reinventează modul în care funcționează afacerea ta cu AI
A fost util acest articol?
DaNu
Mai multe de la Inteligența artificială
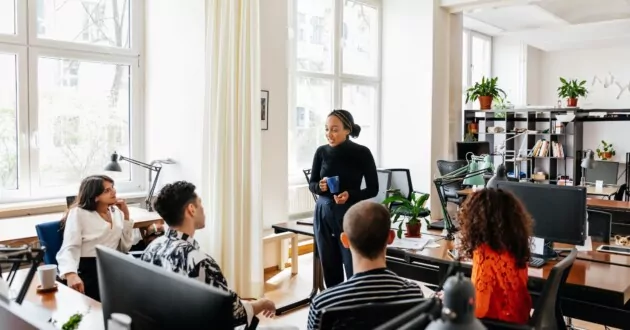
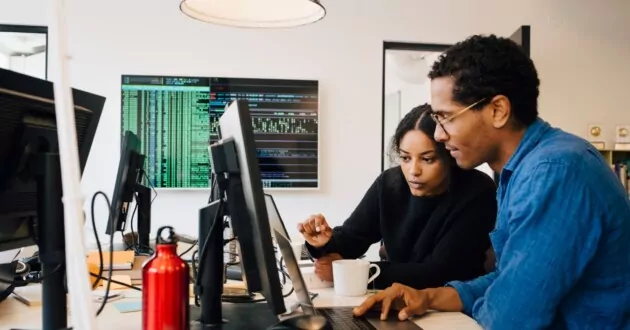
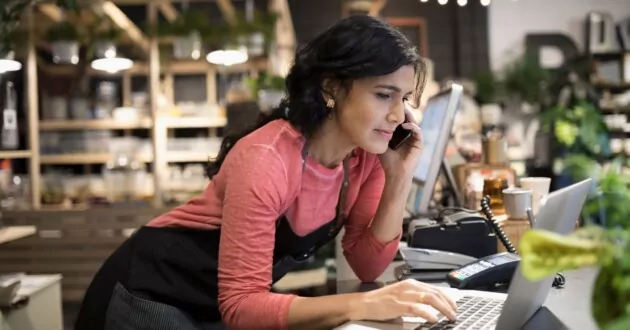
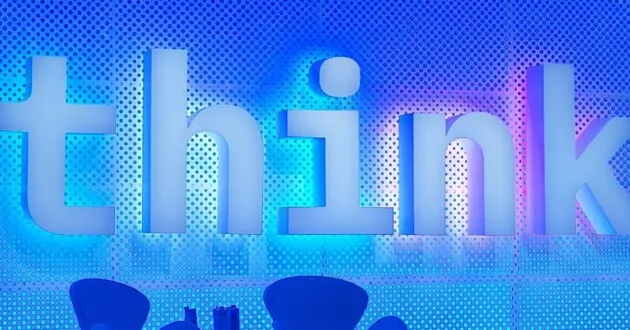
Buletine informative IBM
Primiți buletinele noastre informative și actualizările subiectelor care oferă cele mai recente idei de lider și perspective despre tendințele emergente.
Abonează-te acum
Mai multe buletine informative
- Distribuție de conținut bazat pe SEO și PR. Amplifică-te astăzi.
- PlatoData.Network Vertical Generative Ai. Împuterniciți-vă. Accesați Aici.
- PlatoAiStream. Web3 Intelligence. Cunoștințe amplificate. Accesați Aici.
- PlatoESG. carbon, CleanTech, Energie, Mediu inconjurator, Solar, Managementul deșeurilor. Accesați Aici.
- PlatoHealth. Biotehnologie și Inteligență pentru studii clinice. Accesați Aici.
- Sursa: https://www.ibm.com/blog/why-we-need-diverse-multidisciplinary-coes-for-model-risk/
- :are
- :este
- :nu
- :Unde
- $UP
- 000
- 1
- 16
- 2022
- 2024
- 23
- 25
- 28
- 29
- 30
- 300
- 32
- 39
- 40
- 400
- 65
- 7
- 9
- a
- capacitate
- Despre Noi
- mai sus
- AC
- accelera
- precizie
- precis
- precis
- peste
- Acțiune
- adaptate
- adresa
- admite
- adopta
- Promovare
- sfat
- afecta
- agenţi
- AI
- Modele AI
- Sisteme AI
- urmări
- AL
- algoritmică
- algoritmi
- TOATE
- de asemenea
- mereu
- amp
- an
- Google Analytics
- și
- Orice
- aplicație
- aplicatii
- abordare
- SUNT
- domenii
- articol
- artificial
- inteligență artificială
- Inteligența artificială (AI)
- AS
- cere
- evalua
- evaluarea
- evaluare
- asistenți
- asistarea
- At
- audio
- spori
- autor
- disponibil
- evita
- înapoi
- de echilibrare
- BE
- fost
- înainte
- începe
- fiind
- consideră că
- Beneficiile
- Mai bine
- Dincolo de
- părtinire
- distorsiunilor
- Blog
- bloguri
- Albastru
- boston
- atât
- mai larg
- Clădire
- afaceri
- funcții de afaceri
- întreprinderi
- dar
- buton
- by
- CAN
- captura
- capturarea
- carbon
- card
- Carduri
- pasă
- caz
- CAT
- Categorii
- Provoca
- Centru
- Centrul de excelență
- central
- sigur
- contesta
- Caracteristici
- chatbots
- verifica
- copil
- Copii
- cerc
- CSI
- clasă
- clar
- clientii
- îndeaproape
- Cloud
- culoare
- combinaţie
- venire
- Comun
- Comunități
- comunitate
- Companii
- companie
- Terminat
- concept
- consimţământ
- prin urmare
- Lua în considerare
- consultant
- Recipient
- continua
- conversații
- Nucleu
- corecta
- corelat
- A costat
- ar putea
- a creat
- Crearea
- Creatorii
- critic
- mulţime
- CSS
- curatoriale
- În prezent
- personalizat
- client
- experienta clientului
- clienţii care
- de date
- Data
- Deciziile
- cea mai adâncă
- Mod implicit
- Definitii
- livra
- demonstrează
- Implementarea
- descriere
- Amenajări
- Design gândire
- proiectat
- Determina
- dezvolta
- dezvoltat
- diferit
- dificil
- digital
- Transformarea digitală
- nebunie
- Distins
- diferit
- Diversitate
- do
- domeniu
- donat
- elaborate
- conduce
- E&T
- efecte
- efort
- eliminarea
- Încorporarea
- îmbrăţişare
- șmirghel
- de angajați
- permite
- captivant
- Motor
- inginerii
- Engleză
- suficient de
- asigurare
- Intrați
- eroare
- etc
- Eter (ETH)
- etic
- etică
- evalua
- evaluarea
- evaluare
- Chiar
- Fiecare
- pretutindeni
- exacerba
- exemplu
- exemple
- Excelență
- Ieşire
- aștepta
- experienţă
- Experiențe
- expert
- expertiză
- experți
- a explicat
- expus
- extinde
- extrem
- facebook messenger
- facilita
- cu care se confruntă
- factori
- cinste
- fals
- departe
- FAST
- puțini
- Găsi
- Concentra
- urma
- următor
- fonturi
- Pentru
- frunte
- formulare
- Fundație
- cadre
- din
- faţă
- funcții
- aduna
- s-au adunat
- culegere
- generativ
- AI generativă
- generator
- obține
- dat
- oferă
- Caritate
- scop
- Goluri
- Merge
- bine
- guvernare
- Gramatică
- foarte mult
- Grilă
- grup
- Grupului
- Creștere
- ghida
- rău
- Avea
- Rubrică
- auzi
- înălțime
- ajutor
- util
- Înalt
- -l
- închiriere
- angajarea
- lui
- deține
- holistică
- Acasă
- Cum
- Cum Pentru a
- HTTPS
- uman
- inteligența umană
- umilinţă
- Hibrid
- Hibrid cloud
- i
- BOLNAV
- IBM
- ICO
- ICON
- ideal
- identificat
- if
- Ignoranță
- imagine
- imediat
- Impactul
- Impacturi
- punerea în aplicare a
- Punere în aplicare a
- importanță
- important
- îmbunătăţi
- îmbunătățiri
- îmbunătăţeşte
- in
- include
- Inclusiv
- includere
- incorpora
- tot mai mult
- incrementală
- index
- industrie
- Influent
- informații
- Inovaţie
- inovatori
- intrare
- înţelegere
- perspective
- imediat
- in schimb
- Institut
- asigurare
- asigurătorii
- Inteligență
- Inteligent
- scop
- Intenționat
- intenţiile
- interesat
- interese
- intern
- se intersectează
- intervenţie
- intimidant
- intrinsec
- ISN
- problema
- probleme de
- IT
- ESTE
- ianuarie
- alătura
- Alăturaţi-ne
- călătorie
- jpg
- doar
- păstrare
- Cheie
- Cunoaște
- cunoscut
- lipsă
- limbă
- Limbă
- mare
- pe scară largă
- Ultimele
- conduce
- lider
- Conducere
- învăţare
- lăsa
- Nivel
- Pârghie
- răspundere
- minciună
- ca
- Limitat
- Listă
- local
- localizare
- Se pare
- face
- Efectuarea
- administrare
- manual
- multe
- matematica
- matematic
- materie
- max-width
- Mai..
- me
- însemna
- Întâlni
- Mesager
- ar putea
- minute
- minte
- minim
- minute
- Mobil
- model
- Modele
- modernizare
- mai mult
- cele mai multe
- muta
- mult
- multidisciplinare
- trebuie sa
- my
- Natură
- Navigare
- necesar
- Nevoie
- necesar
- nevoilor
- negativ
- Nou
- produse noi
- buletine de știri
- nlp
- Nu.
- nota
- nimic
- acum
- numeroși
- of
- de pe
- oferi
- oferit
- promoții
- de multe ori
- on
- ONE
- open-source
- Opinie
- Oportunităţi
- optimă
- optimizate
- or
- organizații
- Altele
- al nostru
- outperform
- iesiri
- peste
- propriu
- Proprietarii
- pagină
- participante
- trece
- oameni
- efectuarea
- persoană
- personal
- Personal
- perspective
- PHP
- PII
- Planurile
- Plato
- Informații despre date Platon
- PlatoData
- conecteaza
- Politica
- poziţie
- Post
- potenţial
- potențiali clienți
- putere
- puternic
- practică
- practicile
- prezicere
- Predictii
- predictivă
- primar
- Principiile
- Prioritizarea
- privilegiu
- Problemă
- de achiziții publice
- produce
- producere
- productivitate
- Produse
- Programe
- Proiecte
- cum se cuvine
- perspectivă
- prevăzut
- furnizarea
- proxy-uri
- împuternicit
- psihologic
- public
- publicat
- pune
- Întrebări
- repede
- cita
- rareori
- promptitudine
- Citind
- real
- Realitate
- recunoaște
- Recomandări
- reducerea
- rafina
- reflecta
- reflectat
- regulament
- Rapoarte
- reprezentant
- reprezentate
- cereri de
- necesita
- necesar
- cercetare
- răspunde
- responsabil
- responsabil
- sensibil
- venituri
- cresterea veniturilor
- dreapta
- Risc
- factori de risc
- Riscurile
- drum
- roboţi
- sigur
- Siguranţă
- Said
- acelaşi
- spunând
- Scară
- scalare
- Ştiinţă
- Ecran
- script-uri
- Caută
- motor de cautare
- sigur
- vedea
- SEO
- servește
- setări
- să
- indicat
- pur şi simplu
- teren
- moale
- mic
- inteligent
- Boxe inteligente
- SMS-uri
- So
- soluţie
- soluţii
- unele
- Surse
- Spaţiu
- difuzoare
- Sponsorizat
- pătrate
- părțile interesate
- standard
- Începe
- de ultimă oră
- statistic
- statistică
- Elevi
- Studiu
- subiect
- subscrie
- astfel de
- a sustine
- De sprijin
- SVG
- sisteme
- T
- tabel
- luare
- obiective
- profesor
- echipe
- Tehnic
- Tehnologii
- terţiar
- a) Sport and Nutrition Awareness Day in Manasia Around XNUMX people from the rural commune Manasia have participated in a sports and healthy nutrition oriented activity in one of the community’s sports ready yards. This activity was meant to gather, mainly, middle-aged people from a Romanian rural community and teach them about the benefits that sports have on both their mental and physical health and on how sporting activities can be used to bring people from a community closer together. Three trainers were made available for this event, so that the participants would get the best possible experience physically and so that they could have the best access possible to correct information and good sports/nutrition practices. b) Sports Awareness Day in Poiana Țapului A group of young participants have taken part in sporting activities meant to teach them about sporting conduct, fairplay, and safe physical activities. The day culminated with a football match.
- decât
- Mulțumiri
- acea
- lumea
- lor
- Lor
- temă
- se
- Acestea
- ei
- crede
- Gândire
- acest
- aceste
- gândit
- conducerea gândirii
- trei
- Prin
- timp
- Titlu
- la
- împreună
- Unelte
- top
- subiect
- greu
- Tren
- Pregătire
- Transformare
- Tendinţe
- adevărat
- demn de încredere
- stare de nervozitate
- Două
- tip
- tipic
- înţelege
- nedrept
- unic
- deblocare
- actualizări
- pe
- URL-ul
- us
- utilizare
- carcasa de utilizare
- utilizări
- Utilizand
- valoare
- Valori
- varietate
- variabil
- foarte
- Virtual
- volum
- W
- vrea
- a fost
- we
- BINE
- Ce
- Ce este
- cand
- dacă
- care
- în timp ce
- OMS
- pe cine
- de ce
- pe larg
- mai larg
- voi
- înţelepciune
- ÎNŢELEPT
- cu
- Cuvânt
- WordPress
- Apartamente
- fabrică
- lume
- ar
- scris
- Greșit
- încă
- tu
- Ta
- zephyrnet