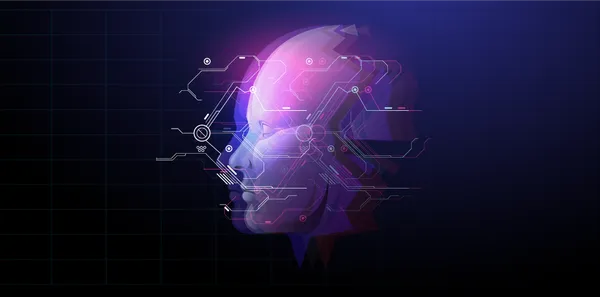
Introduction: The Era of Generative AI
Generative AI has gained significant traction in recent years, with the potential to revolutionize the way we create content, design products, and interact with technology. Generative Adversarial Networks, or GANs, is a type of deep learning model that can generate new, original data based on patterns it has learned from existing data. It plays an important role in content creation, improving Natural Language Processing, etc. This article aims to provide a comprehensive understanding of generative AI for laypeople, explaining its core concepts, applications, and potential impact on various industries.
Also Read: Amazon Introduces Groundbreaking AI Tools to Revolutionize Generative AI and Software Development
What is Generative AI?
Generative AI refers to a type of artificial intelligence that can create new, original content, such as images, text, music, or even entire product designs. By using advanced algorithms and machine learning techniques, generative AI models learn patterns from existing data and generate novel content based on those patterns. This creative process enables AI to develop unique outputs that have never been seen or heard before. This makes it a powerful tool in numerous fields.
How Does It Work?
At the heart of generative AI are two primary components: the generative model and the discriminative model. These two models work together in a process called Generative Adversarial Networks (GANs), which is one of the most popular techniques for creating generative AI.
- Generative Model: The generative model is responsible for creating new content. It learns patterns from existing data and uses these patterns to generate new, unique outputs. This model acts like an artist, creating new content by using the information it has learned from the data it has been trained on.
- Discriminative Model: The discriminative model acts as a critic or evaluator, assessing the quality of the content created by the generative model. It distinguishes between real data and the content generated by the generative model. The discriminative model’s feedback helps the generative model improve its outputs over time, refining its creations until they closely resemble the original data.
The GANs process involves the generative and discriminative models working in tandem, continuously refining and improving the generated content. This iterative process results in high-quality, unique outputs that can be challenging to distinguish from real data.
What Are the Applications?
Generative AI has wide-ranging applications across various industries, including:
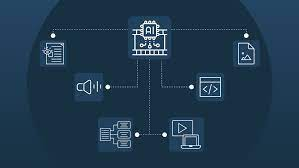
Source: LeewayHertz
- Art and Design: AI-generated artwork, music, and product designs are gaining popularity. Also, artists and designers are leveraging generative AI to create innovative and unique pieces that push the boundaries of human creativity.
- Natural Language Processing: Generative AI models are used in chatbots and language translation applications. This enables more human-like, contextually relevant responses, and translations.
- Medicine and Drug Discovery: Generative AI can analyze vast amounts of medical data and generate potential new drug compounds, streamlining the drug discovery process and potentially saving time and resources.
- Gaming: AI-generated environments, characters, and narratives are used in video games. This makes them more immersive and dynamic. Moreover, as they gain experience, they adapt to players’ actions and preferences.
- Marketing and Advertising: Generative AI can create personalized advertisements and promotional content tailored to individual users. This enhances customer engagement and improves conversion rates.
Also Read: How to Use Generative AI to Create Beautiful Pictures For Free?
Explaining Complex Terms
Generative AI involves several complex terms that might be confusing to laypeople. Here’s a simple explanation of some of these terms:
- Generative Adversarial Networks (GANs): Generative Adversarial Networks or GANs are a type of AI model that consists of two parts. Part one is a generative model that creates content. And part two is a discriminative model that evaluates the quality of the generated content. The two models work together in a continuous process to refine and improve the generated content.
- Machine Learning: Machine learning is a subset of artificial intelligence that involves teaching computers to learn patterns from data. This allows them to make decisions and predictions or generate content without requiring explicit programming.
- Algorithms: Algorithms are a series of instructions or rule a computer follows to solve a problem or perform a task. In generative AI, algorithms help the Artificial Intelligence model learn patterns and create new content based on those patterns.
- Training Data: Training data is the initial set of information used to teach a machine learning model, such as a generative AI, to recognize patterns and make predictions. The quality and quantity of the training data directly impact the model’s performance and the outputs it generates.
Challenges and Ethical Considerations
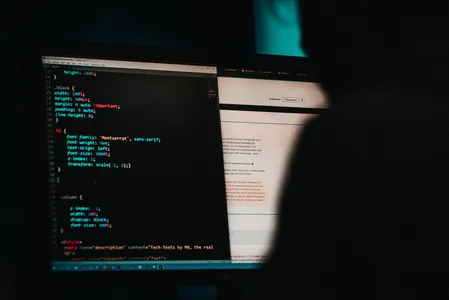
Despite the numerous benefits and applications of generative AI, there are also challenges and ethical considerations, such as:
- Data Privacy: These models require vast amounts of data to train. Hence it raises concerns about data privacy and the potential misuse of sensitive information.
- Intellectual Property: The question of ownership and intellectual property rights for content generated by AI is still an evolving area of discussion, as it challenges traditional notions of authorship and creativity.
- Quality Control: Ensuring the quality and accuracy of AI-generated content, especially in critical fields like medicine and drug discovery, is essential to prevent potential harm or misinformation.
- Bias and Discrimination: Artificial Intelligence models can inadvertently perpetuate biases present in the training data. Thus, leading to biased and potentially discriminatory outputs. We must address the crucial challenge of ensuring fairness and eliminating biases in generative AI models.
The Future of Generative AI
Generative AI holds immense potential to revolutionize various industries, from art and design to medicine and marketing. By understanding its core concepts, applications, and challenges, we can better harness its power to create a future filled with innovative, unique, and valuable content. As it continues to evolve, it will be crucial to address ethical considerations and potential challenges. Additionally, we must ensure that this technology benefits society as a whole.
Related
- SEO Powered Content & PR Distribution. Get Amplified Today.
- Platoblockchain. Web3 Metaverse Intelligence. Knowledge Amplified. Access Here.
- Minting the Future w Adryenn Ashley. Access Here.
- Source: https://www.analyticsvidhya.com/blog/2023/04/what-is-generative-ai-the-creative-intelligence-behind-chatgpt/
- :is
- a
- About
- accuracy
- across
- actions
- acts
- adapt
- Additionally
- address
- advanced
- adversarial
- Advertising
- AI
- aims
- algorithms
- allows
- amounts
- analyze
- and
- applications
- ARE
- AREA
- Art
- article
- artificial
- artificial intelligence
- artist
- Artists
- artwork
- AS
- Assessing
- Authorship
- based
- BE
- beautiful
- before
- behind
- benefits
- Better
- between
- boundaries
- by
- called
- CAN
- challenge
- challenges
- challenging
- characters
- chatbots
- ChatGPT
- closely
- complex
- components
- comprehensive
- computer
- computers
- concepts
- Concerns
- confusing
- considerations
- content
- content-creation
- continues
- continuous
- continuously
- control
- Conversion
- Core
- create
- created
- creates
- Creating
- creation
- creations
- Creative
- creativity
- critical
- crucial
- customer
- Customer Engagement
- data
- data privacy
- decisions
- deep
- deep learning
- Design
- designers
- designs
- develop
- directly
- discover
- discovery
- Discrimination
- discussion
- distinguish
- drug
- drug discovery
- dynamic
- eliminating
- enables
- engagement
- Enhances
- ensure
- ensuring
- Entire
- environments
- Era
- especially
- essential
- etc
- ethical
- Even
- evolve
- evolving
- existing
- experience
- explaining
- explanation
- fairness
- feedback
- Fields
- filled
- follows
- For
- Free
- from
- future
- Gain
- gaining
- Games
- GANs
- generate
- generated
- generates
- generative
- generative adversarial networks
- Generative AI
- generative model
- groundbreaking
- Have
- heard
- Heart
- help
- helps
- here
- high-quality
- holds
- HTTPS
- human
- images
- immense
- immersive
- Impact
- important
- improve
- improves
- improving
- in
- Including
- individual
- industries
- information
- initial
- innovative
- instructions
- intellectual
- intellectual property
- Intelligence
- interact
- Introduces
- Introduction
- IT
- ITS
- language
- leading
- LEARN
- learned
- learning
- leveraging
- like
- machine
- machine learning
- Machine Learning Techniques
- make
- MAKES
- Marketing
- medical
- medical data
- medicine
- might
- Misinformation
- model
- models
- more
- Moreover
- most
- Most Popular
- Music
- narratives
- networks
- New
- novel
- numerous
- numerous benefits
- of
- on
- ONE
- original
- ownership
- part
- parts
- patterns
- perform
- performance
- Personalized
- Pictures
- pieces
- plato
- Plato Data Intelligence
- PlatoData
- Popular
- popularity
- potential
- potentially
- power
- powerful
- Predictions
- preferences
- present
- prevent
- primary
- privacy
- Problem
- process
- processing
- Product
- Products
- Programming
- promotional
- property
- Property Rights
- provide
- Push
- quality
- quantity
- question
- raises
- Rates
- Read
- real
- recent
- recognize
- refers
- refine
- refining
- relevant
- require
- Resources
- responsible
- Results
- revolutionize
- rights
- Role
- Rule
- saving
- sensitive
- Series
- set
- several
- significant
- Simple
- Society
- Software
- SOLVE
- some
- Still
- streamlining
- such
- tailored
- Tandem
- Task
- Teaching
- techniques
- Technology
- terms
- that
- The
- the information
- Them
- These
- time
- to
- together
- tool
- tools
- traction
- traditional
- Train
- trained
- Training
- Translation
- Translations
- understanding
- unique
- us
- use
- users
- Valuable
- variety
- various
- Vast
- Video
- video games
- Way..
- What
- What is
- which
- will
- with
- without
- Work
- work together
- working
- years
- zephyrnet