With AI models able to detect patterns and make predictions that would be difficult or impossible for a human to do manually, the potential applications for tools such as ChatGPT across the healthcare, finance and customer service industries are huge.
Yet while organisations’ priorities around AI should be to assess the opportunities generative AI tools offer their business in terms of competitive advantage, the topic of data privacy has become a prime concern. Managing the responsible use of AI, with its potential to produce biased outcomes, needs careful consideration.
While the potential benefits of these models are immense, organisations should carefully examine the ethical and practical considerations to use AI in a responsible way with safe and secure AI data protection. By optimising their overall user experience with ChatGPT, organisations can improve their AI trustworthiness.
AI privacy concerns
Just as many other cutting-edge technologies, AI will undoubtedly raise some questions and challenges for those looking to deploy it in their tech stacks. In fact, a survey by Progress revealed that 65% of businesses and IT executives currently believe there is data bias in their respective organisations and 78% say this will worsen as AI adoption increases.
Probably the biggest privacy concern is around using private company data in tandem with publicly facing and internal AI platforms. For instance, this might be a healthcare organisation storing confidential patient data or the employee payroll data of a large corporation.
For AI to be most effective, you need a large sample size of high-quality public and/or private data and organisations with access to confidential data, such as healthcare companies with medical records, have a competitive advantage when building AI-based solutions. Above all, these organisations with such sensitive data must consider ethical and regulatory requirements surrounding data privacy, fairness, explainability, transparency, robustness and access.
Large language models (LLM) are powerful AI models trained on text data to perform various natural language processing tasks, including language translation, question answering, summarisation and sentiment analysis. These models are designed to analyse language in a way that mimics human intelligence, allowing them to process, understand and generate human speech.
Risks for private data when using AI
However, with these complex models come ethical and technical challenges which can pose risks for data accuracy, copyright infringement and potential libel cases. Some of the challenges for using chatbot AIs effectively include:
- Hallucinations – In AI, a hallucination is when it reports error-filled answers to the user and these are all too common. The way the LLMs predict the next word makes answers sound plausible, while the information may be incomplete or false. For instance, if a user asks a chatbot for the average revenue of a competitor, these numbers could be way off.
- Data bias – LLMs can also exhibit biases, which means they can produce results that reflect the biases in the training data rather than objective reality. For example, a language model trained on a predominantly male dataset might produce biased output regarding gendered topics.
- Reasoning/Understanding – LLMs may also need help with tasks that require deeper reasoning or understanding of complex concepts. A LLM can be trained to answer questions that require a nuanced understanding of culture or history. It is possible for models to perpetuate stereotypes or provide misinformation if not trained and monitored effectively.
In addition to these, other risks can include Data Cutoffs, which is when a model’s memory tends to be out of date. Another possible challenge is to understand how the LLM generated its response as the AI is not trained effectively to show its reasoning used to construct a response.
Using semantic knowledge to deliver trustworthy data
Tech teams are looking for assistance with using private data for ChatGPT. Despite the increase in accuracy and efficiency, LLMs, not to mention their users, can still need help with answers. Especially since the data can lack context and meaning. A strong, secure, transparent, governed AI knowledge management solution is the answer. With a semantic data platform, users can increase accuracy and efficiency while introducing governance.
By achieving an answer that is a combination of ChatGPT’s answer validated with semantic knowledge from a semantic data platform, the combined results will allow LLMs and users to easily access and fact check the results against the source content and the captured SME knowledge.
This allows the AI tool to store and query structured and unstructured data as well as to capture subject matter expert (SME) content via its intuitive GUI. By extracting facts found within the data and tagging the private data with semantic knowledge, user questions or inputs and specific ChatGPT answers can also be tagged with this knowledge.
Protecting sensitive data can unlock AI’s true potential
As with all technologies, guarding against unexpected inputs or situations is even more important with LLMs. In successfully addressing these challenges, the trustworthiness of our solutions will increase along with user satisfaction ultimately leading to the solution’s success.
As a first step in exploring the use of AI for their organisation, IT and security pros must look for ways to protect sensitive data while leveraging it to optimise outcomes for their organisation and its customers.
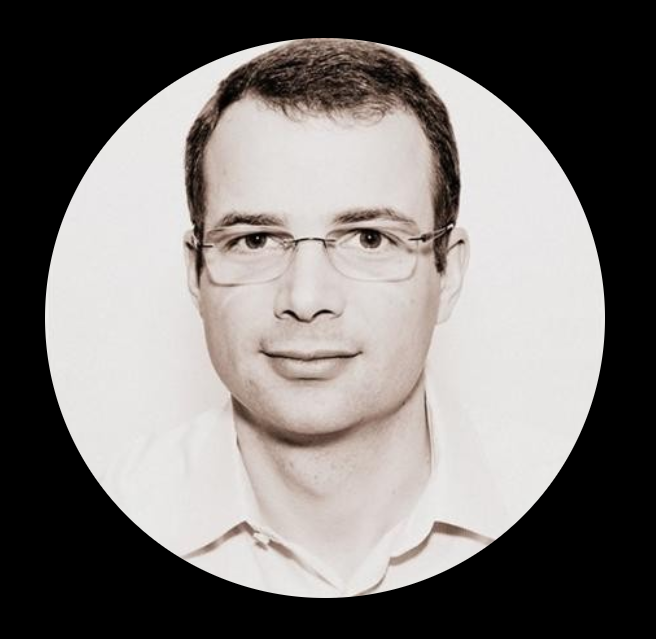
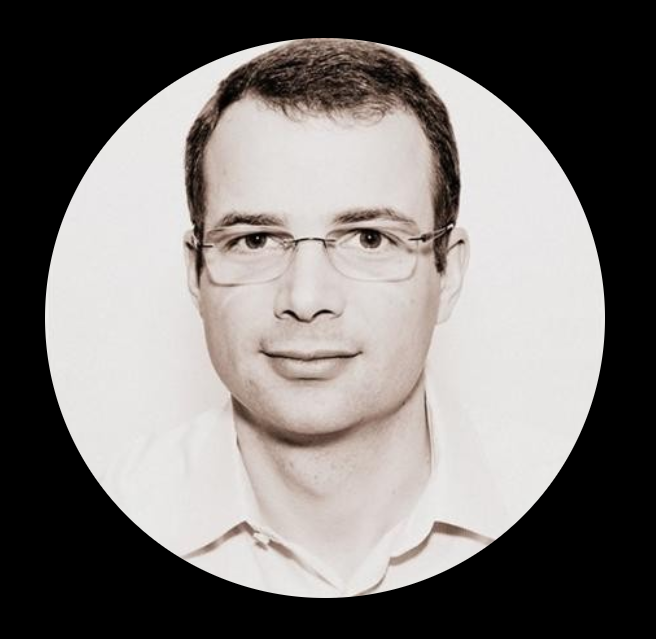
Article by Matthieu Jonglez, a VP technology – application and data platform at Progress.
Comment on this article below or via X: @IoTNow_
- SEO Powered Content & PR Distribution. Get Amplified Today.
- PlatoData.Network Vertical Generative Ai. Empower Yourself. Access Here.
- PlatoAiStream. Web3 Intelligence. Knowledge Amplified. Access Here.
- PlatoESG. Carbon, CleanTech, Energy, Environment, Solar, Waste Management. Access Here.
- PlatoHealth. Biotech and Clinical Trials Intelligence. Access Here.
- Source: https://www.iot-now.com/2024/01/18/141953-using-your-own-data-to-mitigate-ai-privacy-issues-and-improve-ai-trust/
- :has
- :is
- :not
- 195
- 200
- a
- Able
- above
- access
- accuracy
- achieving
- across
- addition
- addressing
- Adoption
- ADvantage
- against
- AI
- AI adoption
- AI Data
- AI models
- All
- allow
- Allowing
- allows
- along
- also
- an
- analyse
- analysis
- and
- Another
- answer
- answers
- Application
- applications
- ARE
- around
- article
- AS
- assess
- Assistance
- At
- average
- BE
- become
- believe
- below
- benefits
- bias
- biased
- biases
- Biggest
- Building
- business
- businesses
- by
- CAN
- capture
- captured
- careful
- carefully
- cases
- challenge
- challenges
- chatbot
- ChatGPT
- check
- combination
- combined
- come
- Common
- Companies
- company
- competitive
- competitor
- complex
- concepts
- Concern
- Consider
- consideration
- considerations
- construct
- content
- context
- copyright
- copyright infringement
- CORPORATION
- could
- Culture
- Currently
- customer
- Customer Service
- Customers
- cutting-edge
- Cutting-Edge Technologies
- data
- data bias
- Data Platform
- data privacy
- data protection
- Date
- deeper
- deliver
- deploy
- designed
- Despite
- detect
- difficult
- do
- easily
- Effective
- effectively
- efficiency
- Employee
- especially
- Ether (ETH)
- ethical
- Even
- examine
- example
- executives
- exhibit
- experience
- expert
- Explainability
- Exploring
- facing
- fact
- Fact Check
- facts
- fairness
- false
- finance
- First
- For
- found
- from
- generate
- generated
- generative
- Generative AI
- governance
- governed
- Have
- healthcare
- help
- high-quality
- history
- How
- HTTPS
- huge
- human
- human intelligence
- if
- immense
- important
- impossible
- improve
- in
- include
- Including
- Increase
- Increases
- industries
- information
- infringement
- inputs
- instance
- Intelligence
- internal
- introducing
- intuitive
- iot
- issues
- IT
- ITS
- knowledge
- Knowledge Management
- Lack
- language
- large
- leading
- leveraging
- Look
- looking
- make
- MAKES
- management
- Management Solution
- managing
- manually
- many
- Matter
- max-width
- May..
- meaning
- means
- medical
- Memory
- mention
- might
- Misinformation
- Mitigate
- model
- models
- monitored
- more
- most
- must
- Natural
- Natural Language
- Natural Language Processing
- Need
- needs
- news
- next
- now
- numbers
- objective
- of
- off
- offer
- on
- opportunities
- optimise
- optimising
- or
- organisation
- Organisations
- Other
- our
- out
- outcomes
- output
- overall
- own
- patient
- patient data
- patterns
- Payroll
- perform
- platform
- Platforms
- plato
- Plato Data Intelligence
- PlatoData
- plausible
- possible
- potential
- powerful
- Practical
- predict
- Predictions
- predominantly
- Prime
- privacy
- private
- process
- processing
- produce
- Progress
- PROS
- protect
- protection
- provide
- public
- publicly
- question
- Questions
- raise
- rather
- Reality
- records
- reflect
- regarding
- regulatory
- Reports
- require
- Requirements
- respective
- response
- responsible
- Results
- revenue
- risks
- robustness
- safe
- satisfaction
- say
- secure
- security
- sensitive
- sentiment
- service
- should
- show
- since
- situations
- Size
- SME
- solution
- Solutions
- some
- Sound
- Source
- specific
- speech
- Stacks
- Step
- Still
- strong
- structured
- structured and unstructured data
- subject
- success
- Successfully
- such
- Surrounding
- Survey
- Tandem
- tasks
- teams
- tech
- Technical
- Technologies
- Technology
- tends
- terms
- text
- than
- that
- The
- the information
- The Source
- their
- Them
- There.
- These
- they
- this
- those
- to
- too
- tool
- tools
- topic
- Topics
- trained
- Training
- Translation
- Transparency
- transparent
- true
- Trust
- trustworthiness
- trustworthy
- Ultimately
- understand
- understanding
- undoubtedly
- Unexpected
- unlock
- use
- used
- User
- User Experience
- users
- using
- validated
- various
- via
- vp
- Way..
- ways
- WELL
- when
- which
- while
- will
- with
- within
- Word
- worsen
- would
- X
- you
- Your
- zephyrnet