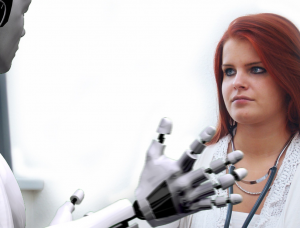
Artificial intelligence (AI) has revolutionized various industries, offering numerous benefits and opportunities. However, concerns have emerged regarding the potential for AI to perpetuate discrimination and biases. This article explores the topic of AI discrimination, shedding light on the challenges of identifying and addressing biases embedded within AI systems. Industry insiders express doubts about the moral and ethical implications of AI, citing worries about misinformation, biases in algorithms, and the generation of misleading content. As debates surrounding AI intensify, there is a growing call for meaningful regulation to ensure transparency, accountability, and the protection of fundamental rights.
Challenges for Financial Industries with AI
According to Nabil Manji, the head of crypto and Web3 at Worldpay by FIS, the effectiveness of AI products depends heavily on the quality of the source material used for training. In an interview with CNBC, Manji explained that two main factors contribute to the performance of AI: the data it has access to and the capabilities of the large language model.
To illustrate the significance of data, Manji mentioned that companies like Reddit have publicly declared restrictions on data scraping, requiring payment for access. In the financial services sector, he highlighted the challenge of fragmented data systems in various languages and formats. This lack of consolidation and harmonization limits the effectiveness of AI-driven products, especially when compared to industries with standardized and modernized data infrastructure.
According to Manji, the utilization of blockchain or distributed ledger technology may offer a potential solution to tackle this problem. This innovative approach can provide enhanced transparency into the fragmented data stored within the intricate systems of conventional banks. However, he acknowledged that the highly regulated and slow-moving nature of banks might impede their ability to swiftly adopt new AI tools, unlike more agile tech companies such as Microsoft and Google, which have been at the forefront of driving innovation for the past few decades.
Considering these factors, it becomes evident that the financial industry faces unique challenges in leveraging AI due to the complexities of data integration and the inherent nature of the banking sector.
According to Rumman Chowdhury, a former head of machine learning ethics, transparency, and accountability at Twitter, lending is a notable example of how bias in AI systems can adversely affect marginalized communities. Speaking at a panel discussion in Amsterdam, Chowdhury highlighted the historical practice of “redlining” in Chicago during the 1930s. Redlining involved denying loans to predominantly African American neighborhoods based on racial demographics.
Chowdhury explained that although modern algorithms may not explicitly include race as a data point, biases can still be implicitly encoded. When developing algorithms to assess the riskiness of districts and individuals for lending purposes, historical data that contains biases can inadvertently perpetuate discrimination.
Angle Bush, the visionary behind Black Women in Artificial Intelligence, highlighted the importance of acknowledging the dangers associated with reproducing biases embedded in historical data when employing AI systems to make loan approval determinations. Such a practice can lead to the automatic rejection of loan applications from marginalized communities, thereby perpetuating racial or gender inequalities.
Frost Li, an experienced AI developer, pointed out the challenges of personalization in AI integration. Selecting “core features” for training AI models can sometimes involve unrelated factors that may lead to biased outcomes. Li provided an example of how fintech startups targeting foreigners might face different credit assessment criteria compared to local banks, which are more familiar with the local schools and communities.
Niklas Guske, the COO of Taktile, a startup specializing in automating decision-making for fintechs, clarified that generative AI is not typically used for creating credit scores or risk scoring of consumers. On the contrary, its strength lies in the preprocessing of unstructured data, like text files, to enhance the data quality for conventional underwriting models.
In summary, the use of AI in lending and financial services raises concerns about bias and discrimination. The historical biases embedded in data and the selection of irrelevant features during AI training can lead to unfair outcomes. It is crucial for banks and financial institutions to recognize and address these issues to prevent the inadvertent perpetuation of discrimination when implementing AI solutions.
Prove of AI-Discrimination
Proving AI-based discrimination can be challenging, as highlighted by examples such as the case involving Apple and Goldman Sachs. The New York State Department of Financial Services dismissed the allegations of imposing lower limits on the Apple Card for women, citing a lack of substantiating evidence.
Kim Smouter, director of the European Network Against Racism, points out that the mass deployment of AI brings about opacity in decision-making processes, making it difficult for individuals to identify and address discrimination.
Smouter explains that individuals often have limited knowledge of how AI systems operate, making it challenging to detect instances of discrimination or systemic biases. It becomes even more complex when the discrimination is part of a broader issue that affects multiple individuals. Smouter references the Dutch child welfare scandal, where a large number of benefit claims were wrongly labeled as fraudulent due to institutional bias. The discovery of such dysfunctions is challenging, and obtaining redress can be difficult and time-consuming, leading to significant and sometimes irreversible harm.
These examples illustrate the inherent difficulties in substantiating AI-based discrimination and obtaining remedies when such discrimination occurs. The complexity of AI systems and the lack of transparency in decision-making processes can make it challenging for individuals to recognize and address instances of discrimination effectively.
According to Chowdhury, there is a pressing need for a global regulatory body similar to the United Nations to address the risks associated with AI. While AI has shown remarkable innovation, concerns have been raised by technologists and ethicists regarding its moral and ethical implications. These concerns encompass issues such as misinformation, embedded racial and gender biases in AI algorithms, and the generation of misleading content by tools like ChatGPT.
Chowdhury expresses worry about entering a post-truth world where online information, including text, video, and audio, becomes untrustworthy due to generative AI. This raises questions about how we can ensure the integrity of information and how we can rely on it for making informed decisions. With the European Union’s AI Act as an example, meaningful regulation of AI is crucial at this moment. However, there are concerns about the lengthy timeline it takes for regulatory proposals to become effective, potentially delaying necessary actions.
Smouter emphasizes the need for greater transparency and accountability in AI algorithms. This includes making algorithms more understandable for non-experts, conducting tests and publishing results, establishing independent complaint processes, conducting periodic audits and reporting, and involving racialized communities in the design and deployment of technology. Enforcement of the AI Act, which takes a fundamental rights perspective and introduces concepts like redress, is anticipated to commence in approximately two years. Reducing this timeline would be advantageous to uphold transparency and accountability as integral aspects of innovation.
- SEO Powered Content & PR Distribution. Get Amplified Today.
- PlatoData.Network Vertical Generative Ai. Empower Yourself. Access Here.
- PlatoAiStream. Web3 Intelligence. Knowledge Amplified. Access Here.
- PlatoESG. Automotive / EVs, Carbon, CleanTech, Energy, Environment, Solar, Waste Management. Access Here.
- BlockOffsets. Modernizing Environmental Offset Ownership. Access Here.
- Source: https://www.forexnewsnow.com/fintech/the-urgency-of-addressing-ai-discrimination-transparency-accountability-and-regulatory-timelines/
- :has
- :is
- :not
- :where
- a
- ability
- About
- access
- accountability
- acknowledged
- Act
- actions
- address
- addressing
- adopt
- advantageous
- adversely
- affect
- African
- against
- agile
- AI
- AI Act
- AI systems
- AI training
- algorithms
- Allegations
- Although
- American
- amsterdam
- an
- and
- Anticipated
- Apple
- Apple Card
- applications
- approach
- approval
- approximately
- ARE
- article
- artificial
- artificial intelligence
- AS
- aspects
- assess
- assessment
- associated
- At
- audio
- audits
- Automatic
- automating
- Banking
- banking sector
- Banks
- based
- BE
- become
- becomes
- been
- behind
- benefit
- benefits
- bias
- biased
- biases
- Black
- blockchain
- body
- Brings
- broader
- by
- call
- CAN
- capabilities
- card
- case
- challenge
- challenges
- challenging
- ChatGPT
- chicago
- child
- claims
- clarified
- CNBC
- Communities
- Companies
- compared
- complaint
- complex
- complexities
- complexity
- concepts
- Concerns
- conducting
- consolidation
- Consumers
- contains
- content
- contrary
- contribute
- conventional
- coo
- Creating
- credit
- criteria
- crucial
- crypto
- dangers
- data
- data infrastructure
- data integration
- data quality
- debates
- decades
- Decision Making
- decisions
- Demographics
- Department
- depends
- deployment
- Design
- Developer
- developing
- different
- difficult
- difficulties
- Director
- discovery
- Discrimination
- discussion
- distributed
- Distributed Ledger
- distributed ledger technology
- driving
- due
- during
- Dutch
- Effective
- effectively
- effectiveness
- embedded
- emerged
- emphasizes
- encompass
- enforcement
- enhance
- enhanced
- ensure
- entering
- especially
- establishing
- ethical
- ethics
- European
- Even
- evidence
- evident
- example
- examples
- experienced
- explained
- Explains
- explores
- express
- Face
- faces
- factors
- familiar
- Features
- few
- Files
- financial
- financial industries
- Financial institutions
- financial services
- fintech
- fintech startups
- fintechs
- FIS
- For
- forefront
- Former
- fragmented
- fraudulent
- from
- fundamental
- Gender
- generation
- generative
- Generative AI
- Global
- goldman
- Goldman Sachs
- greater
- Growing
- harm
- Have
- he
- head
- heavily
- Highlighted
- highly
- historical
- How
- However
- HTTPS
- identify
- identifying
- implementing
- implications
- importance
- imposing
- in
- include
- includes
- Including
- independent
- individuals
- industries
- industry
- inequalities
- information
- informed
- Infrastructure
- inherent
- Innovation
- innovative
- Institutional
- institutions
- integral
- integration
- integrity
- Intelligence
- Interview
- into
- Introduces
- involve
- involved
- involving
- issue
- issues
- IT
- ITS
- knowledge
- Lack
- language
- Languages
- large
- lead
- leading
- learning
- Ledger
- lending
- leveraging
- li
- lies
- light
- like
- Limited
- limits
- loan
- Loans
- local
- LOCAL BANKS
- lower
- machine
- machine learning
- Main
- make
- Making
- Mass
- material
- May..
- meaningful
- mentioned
- Microsoft
- might
- Misinformation
- misleading
- model
- models
- Modern
- moment
- moral
- more
- multiple
- Nations
- Nature
- necessary
- Need
- network
- New
- New York
- New York state
- New York State Department of Financial Services
- non-experts
- notable
- number
- numerous
- numerous benefits
- obtaining
- of
- offer
- offering
- often
- on
- online
- operate
- opportunities
- or
- out
- outcomes
- panel
- panel discussion
- part
- past
- payment
- performance
- periodic
- personalization
- perspective
- plato
- Plato Data Intelligence
- PlatoData
- Point
- points
- potential
- potentially
- practice
- predominantly
- pressing
- prevent
- Problem
- processes
- Products
- Proposals
- protection
- provide
- provided
- publicly
- Publishing
- purposes
- quality
- Questions
- Race
- racism
- raised
- raises
- recognize
- reducing
- references
- regarding
- regulated
- Regulation
- regulatory
- rely
- remarkable
- Reporting
- restrictions
- Results
- revolutionized
- rights
- Risk
- risks
- Sachs
- Scandal
- Schools
- scores
- scoring
- scraping
- sector
- selecting
- selection
- Services
- shown
- significance
- significant
- similar
- solution
- Solutions
- Source
- speaking
- specializing
- startup
- Startups
- State
- State Department
- Still
- stored
- strength
- such
- SUMMARY
- Surrounding
- swiftly
- systemic
- Systems
- tackle
- takes
- targeting
- tech
- tech companies
- technologists
- Technology
- tests
- that
- The
- The Source
- their
- There.
- thereby
- These
- this
- time-consuming
- timeline
- timelines
- to
- tools
- topic
- Training
- Transparency
- two
- typically
- understandable
- underwriting
- unfair
- unique
- United
- united nations
- unlike
- Uphold
- urgency
- use
- used
- various
- Video
- visionary
- we
- Web3
- Welfare
- were
- when
- which
- while
- with
- within
- Women
- world
- WorldPay
- worry
- would
- years
- york
- zephyrnet