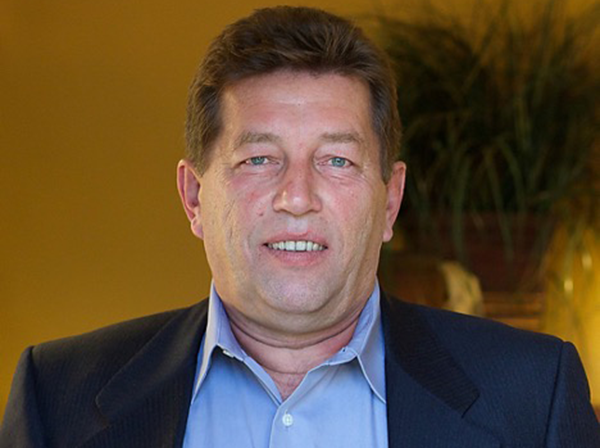
Artificial intelligence has drawn a lot of media attention for everything from taking people’s jobs to spreading disinformation and infringing copyrights, but AI’s impact on cybersecurity may be its most pressing immediate issue.
AI’s impact on security teams is predictably double-edged. When properly applied, it can be a powerful force multiplier for cybersecurity practitioners, through such means as processing vast amounts of data at computer speeds, finding connections between distant data points, discovering patterns, detecting attacks, and predicting attack progressions. But, as security practitioners are well aware, AI is not always properly applied. It intensifies the already imposing lineup of cybersecurity threats, from identity compromise and phishing to ransomware and supply chain attacks.
CISOs and security teams need to understand both the advantages and risks of AI, which requires a substantial rebalancing of skills. Security engineers, for example, must grasp the basics of machine learning, model quality and biases, confidence levels, and performance metrics. Data scientists need to learn cybersecurity fundamentals, attack patterns, and risk modeling to effectively contribute to hybrid teams.
AI Models Need Proper Training to Assist Cybersecurity
The task of dealing with the proliferation of AI-fueled threats compounds the challenges for CISOs and already overworked security teams who must not only deal with new sophisticated phishing campaigns crafted by a large language model (LLM) like ChatGPT, but still have to worry about an unpatched server in the DMZ that could pose a bigger threat.
AI, on the other hand, can save teams a lot of time and effort in risk assessment and detecting threats. It can also help with response – although that must be done carefully. An AI model can shoulder-surf analysts to learn how they triage incidents, and then either perform those tasks on its own or prioritize cases for human review. But teams need to be sure that the right people are giving the AI instruction.
Years ago, for example, I ran an experiment where I had 10 analysts of varying skill levels review 100 cases of suspected data exfiltration. Two senior analysts correctly identified all positives and negatives, three less experienced analysts got almost all of the cases wrong, and the remaining five got random results. No matter how good an AI model is, it would be useless if trained by a team like that.
AI is like a powerful car: It can do wonders in the hands of an experienced driver or a lot of damage in the hands of an inexperienced one. That’s one area where the skills shortage can affect AI’s cybersecurity impact.
How Can CTOs Choose an AI Solution?
Given the hype about AI, organizations might be tempted to simply rush into adopting the technology. But in addition to properly training AI, there are questions CTOs need to answer, starting with suitability issues:
- Does AI fit into the organization’s ecosystem? This includes the platform, external components such as a database and search engine, free and open-source software and licensing, and also the organization’s security and certifications, backup, and failover.
- Does AI scale to the size of the enterprise?
- What skillsets are required for the security team to maintain and operate AI?
CTOs also must address questions specifically for an AI solution:
- Which of the claimed functions of a specific AI product align with your business objectives?
- Can the same functionality be achieved using existing tools?
- Does the solution actually detect threats?
That last question can be difficult to answer because malicious cybersecurity events occur on a minuscule scale compared with legitimate activity. In a limited proof-of-concept study using live data, an AI tool may detect nothing if nothing is there. Vendors often use synthetic data or Red Team attacks to demonstrate an AI’s capability, but the question remains whether it is demonstrating true detection capability or simply validating the assumption under which the indicators were generated.
It’s difficult to determine why an AI thinks something was an attack because AI algorithms are essentially black boxes, still unable to explain how they reached a certain conclusion – as demonstrated by DARPA’s Explainable AI (XAI) program.
Mitigating the Risks of AI
An AI solution is only as good as the data it works with. To ensure ethical behavior, AI models should be trained on ethical data, not on the wholesale collection of garbage that is on the World Wide Web. And any data scientist knows that producing a well-balanced, unbiased, clean dataset to train a model is a difficult, tedious, and unglamorous task.
Because of this, AI models, including LLMs, may eventually be managed in a way similar to how they would best serve cybersecurity – as specialized models (as opposed to “all-knowing” general purpose models) that serve particular fields and are trained on data curated by subject matter experts in the field.
Trying to censor AI in response to the media outcry of the moment will not solve the problem. Only diligent work in creating reliable datasets can do that. Until AI companies – and the VCs that back them – accept this approach as the only way to deliver respectable content, it’s garbage in/garbage out.
Should AI Development Be More Regulated?
AI’s development has generated a lot of legitimate concerns about everything from deepfakes and voice cloning to advanced phishing/vishing/smishing, killer robots, and even the possibility of an AI apocalypse. Eliezer Yudkowsky, one of the most respected names in Artificial General Intelligence (AGI), recently issued a call to “shut it all down,” saying a proposed six-month moratorium wasn’t enough.
But you cannot stop the development of new technologies, a fact that has been evident since the days of alchemists in ancient times. So, from a practical point of view, what can be done to keep AI from growing out of control and to mitigate the risk of an AI-driven extinction event? The answer is many of the same sets of controls employed in other fields that have a potential for weaponization:
- Transparent research. Open-source AI development not only drives innovation and democratizes access, but it also has many safety benefits, from spotting security flaws and dangerous lines of development to creating defenses against potential abuse. Big Tech so far supports open-source efforts, but that could change if competition intensifies. There might be a need for legislative measures to retain open-source access.
- Contain experimentation. All experiments with sufficiently advanced AI need to be sandboxed, with safety and security procedures strictly enforced. These aren’t foolproof measures but might make the difference between a local disturbance and a global catastrophe.
- Kill switches. Like antidotes and vaccines, countermeasures against runaway or destructive AI variants need to be an integral part of the development process. Even ransomware creators build in a kill switch.
- Regulate how it’s used. AI is a technology that can be applied for the good of humanity or abused with disastrous consequences. Regulation of its applications is a task for world governments, and the urgency is much higher than the need to censor the next version of ChatGPT. The EU AI Act is a well-put, concise foundation aimed at preventing misuse without stifling innovation. The U.S. AI Bill of Rights and the recent Executive Order on AI are less specific and seem to focus more on political correctness than on the issues of proper model development, training, and containment. Those measures are just a start, however.
Conclusion
AI is coming to cybersecurity whether CISOs want it or not, and it will bring both substantial benefits and risks to the cybersecurity field, particularly with the eventual arrival of post-quantum cryptography. At a minimum, CISOs should invest the time to understand the benefits of AI-hyped tools and the threats of AI-driven attacks. Whether they invest money in AI depends largely on the tangible benefits of AI security products, the publicized consequences of AI attacks and, to a certain degree, their personal experience with ChatGPT.
The challenge CISOs face is how to implement AI effectively and responsibly.
- SEO Powered Content & PR Distribution. Get Amplified Today.
- PlatoData.Network Vertical Generative Ai. Empower Yourself. Access Here.
- PlatoAiStream. Web3 Intelligence. Knowledge Amplified. Access Here.
- PlatoESG. Carbon, CleanTech, Energy, Environment, Solar, Waste Management. Access Here.
- PlatoHealth. Biotech and Clinical Trials Intelligence. Access Here.
- Source: https://www.dataversity.net/the-impact-of-ai-on-cybersecurity/
- :has
- :is
- :not
- :where
- 10
- 100
- a
- About
- abuse
- abused
- Accept
- access
- achieved
- activity
- actually
- addition
- address
- Adopting
- advanced
- advantages
- affect
- against
- AGI
- ago
- AI
- AI models
- aimed
- algorithms
- align
- All
- almost
- already
- also
- Although
- always
- amounts
- an
- Analysts
- Ancient
- and
- answer
- any
- applications
- applied
- approach
- ARE
- AREA
- arrival
- artificial
- artificial general intelligence
- AS
- assessment
- assist
- assumption
- At
- attack
- Attacks
- attention
- aware
- back
- Backup
- Basics
- BE
- because
- been
- behavior
- benefits
- BEST
- between
- biases
- Big
- big tech
- bigger
- Bill
- Black
- both
- boxes
- bring
- build
- business
- but
- by
- call
- Campaigns
- CAN
- cannot
- capability
- car
- carefully
- cases
- certain
- certifications
- chain
- challenge
- challenges
- change
- ChatGPT
- Choose
- claimed
- clean
- collection
- coming
- Companies
- compared
- competition
- components
- compromise
- computer
- concise
- conclusion
- confidence
- Connections
- Consequences
- Containment
- content
- contribute
- control
- controls
- Copyrights
- correctly
- could
- crafted
- Creating
- creators
- curated
- Cybersecurity
- damage
- Dangerous
- data
- data points
- data scientist
- Database
- datasets
- DATAVERSITY
- Days
- deal
- dealing
- deepfakes
- Degree
- deliver
- democratizes
- demonstrate
- demonstrated
- demonstrating
- depends
- detect
- Detection
- Determine
- Development
- difference
- difficult
- disastrous
- discovering
- disinformation
- Distant
- do
- done
- drawn
- driver
- drives
- ecosystem
- effectively
- effort
- efforts
- either
- employed
- Engine
- Engineers
- enough
- ensure
- Enterprise
- essentially
- ethical
- Europa
- Even
- Event
- events
- eventual
- eventually
- everything
- evident
- example
- executive
- executive order
- exfiltration
- existing
- experience
- experienced
- experiment
- experiments
- experts
- Explain
- external
- extinction
- Face
- fact
- far
- field
- Fields
- finding
- fit
- five
- flaws
- Focus
- For
- Force
- Foundation
- Free
- from
- functionality
- functions
- Fundamentals
- General
- general intelligence
- generated
- Giving
- Global
- good
- got
- Governments
- grasp
- Growing
- had
- hand
- Hands
- Have
- help
- higher
- How
- How To
- However
- HTTPS
- human
- Humanity
- Hybrid
- Hype
- i
- identified
- Identity
- IEEE
- if
- immediate
- Impact
- implement
- imposing
- in
- In other
- incidents
- includes
- Including
- Indicators
- Innovation
- integral
- Intelligence
- Intensifies
- into
- Invest
- issue
- Issued
- issues
- IT
- ITS
- Jobs
- just
- Keep
- Kill
- killer
- knows
- language
- largely
- Last
- LEARN
- learning
- Legislative
- legitimate
- less
- levels
- Licensing
- like
- Limited
- lines
- lineup
- live
- live data
- local
- Lot
- machine
- machine learning
- maintain
- make
- managed
- many
- Matter
- May..
- means
- measures
- Media
- Metrics
- might
- minimum
- misuse
- Mitigate
- model
- modeling
- models
- moment
- money
- moratorium
- more
- most
- much
- must
- names
- Need
- negatives
- New
- New technologies
- next
- nist
- no
- nothing
- objectives
- occur
- of
- often
- on
- ONE
- only
- open source
- Open-source Software
- operate
- opposed
- or
- order
- organizations
- Other
- out
- own
- part
- particular
- particularly
- patterns
- People
- people’s
- perform
- performance
- personal
- phishing
- platform
- plato
- Plato Data Intelligence
- PlatoData
- Point
- Point of View
- points
- political
- possibility
- potential
- powerful
- Practical
- predicting
- pressing
- preventing
- Prioritize
- Problem
- procedures
- process
- processing
- producing
- Product
- Products
- Program
- proper
- properly
- proposed
- purpose
- quality
- question
- Questions
- random
- ransomware
- reached
- rebalancing
- recent
- recently
- Red
- regulated
- Regulation
- reliable
- remaining
- remains
- required
- requires
- research
- respectable
- respected
- response
- responsibly
- Results
- retain
- review
- right
- Risk
- risk assessment
- risks
- robots
- rush
- s
- Safety
- Safety and Security
- same
- Save
- saying
- Scale
- Scientist
- Search
- search engine
- security
- seem
- senior
- serve
- server
- Sets
- shortage
- should
- similar
- simply
- since
- Size
- skill
- skills
- So
- so Far
- Software
- solution
- SOLVE
- something
- sophisticated
- specialized
- specific
- specifically
- speeds
- spotting
- Spreading
- start
- Starting
- Still
- Stop
- Study
- subject
- substantial
- such
- suitability
- supply
- supply chain
- Supports
- sure
- suspected
- Switch
- synthetic
- synthetic data
- taking
- tangible
- Task
- tasks
- team
- teams
- tech
- Technologies
- Technology
- than
- that
- The
- The Basics
- the world
- their
- Them
- then
- There.
- These
- they
- Thinks
- this
- those
- threat
- threats
- three
- Through
- time
- times
- to
- tool
- tools
- Train
- trained
- Training
- triage
- true
- two
- unable
- unbiased
- under
- understand
- until
- urgency
- use
- used
- using
- vaccines
- validating
- varying
- Vast
- VCs
- vendors
- version
- View
- Voice
- want
- was
- Way..
- web
- WELL
- were
- What
- when
- whether
- which
- WHO
- wholesale
- why
- wide
- will
- with
- without
- Work
- works
- world
- world wide web
- worry
- would
- Wrong
- you
- Your
- ZDNET
- zephyrnet