Abstract
The presence of noise is currently one of the main obstacles to achieving large-scale quantum computation. Strategies to characterise and understand noise processes in quantum hardware are a critical part of mitigating it, especially as the overhead of full error correction and fault-tolerance is beyond the reach of current hardware. Non-Markovian effects are a particularly unfavourable type of noise, being both harder to analyse using standard techniques and more difficult to control using error correction. In this work we develop a set of efficient algorithms, based on the rigorous mathematical theory of Markovian master equations, to analyse and evaluate unknown noise processes. In the case of dynamics consistent with Markovian evolution, our algorithm outputs the best-fit Lindbladian, i.e., the generator of a memoryless quantum channel which best approximates the tomographic data to within the given precision. In the case of non-Markovian dynamics, our algorithm returns a quantitative and operationally meaningful measure of non-Markovianity in terms of isotropic noise addition. We provide a Python implementation of all our algorithms, and benchmark these on a range of 1- and 2-qubit examples of synthesised noisy tomography data, generated using the Cirq platform. The numerical results show that our algorithms succeed both in extracting a full description of the best-fit Lindbladian to the measured dynamics, and in computing accurate values of non-Markovianity that match analytical calculations.
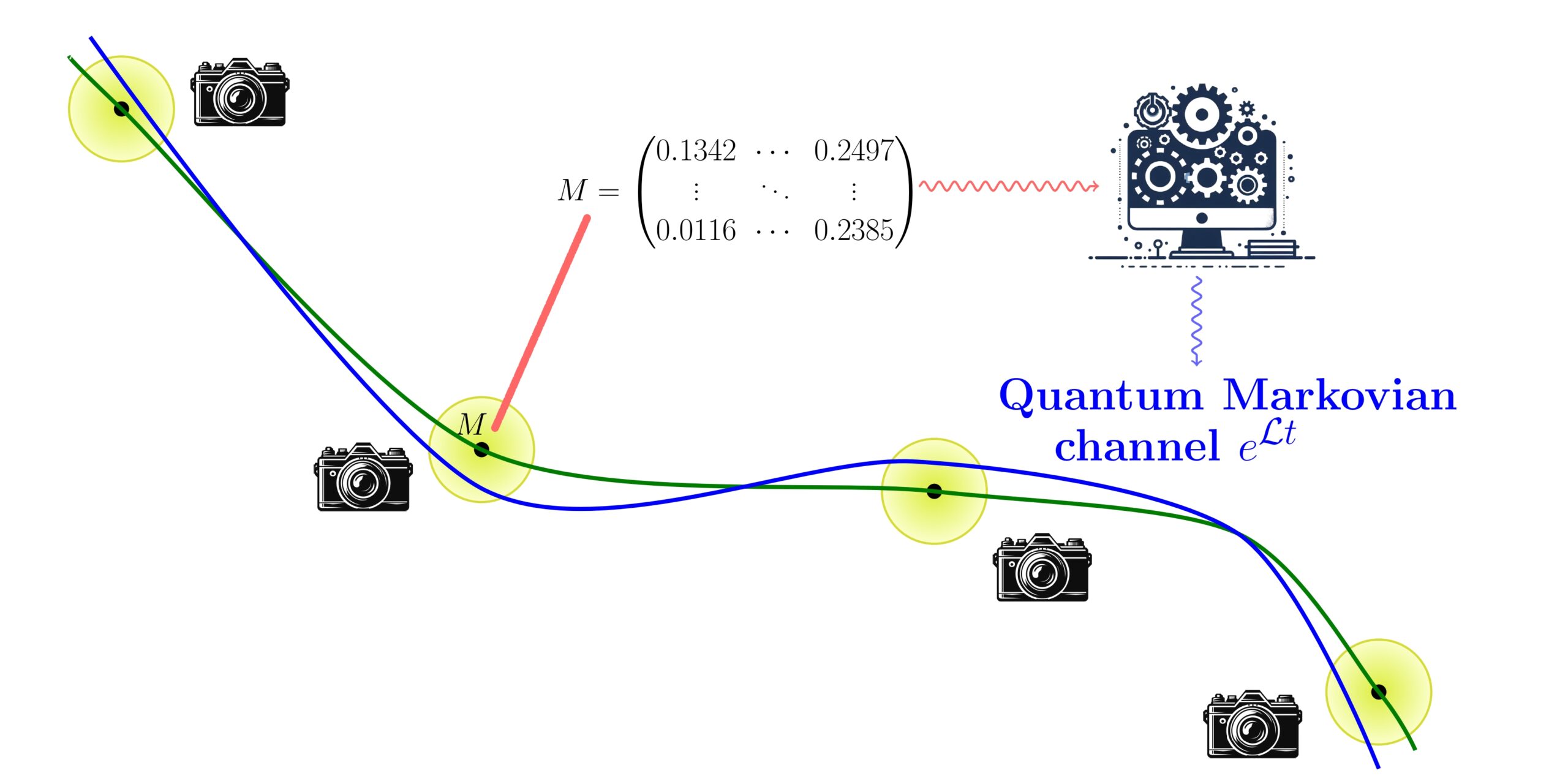
Featured image: Extracting the best-fit Lindblad generator from tomographic data of a quantum channel.
Popular summary
► BibTeX data
► References
[1] John Preskill. “Quantum Computing in the NISQ era and beyond”. In: Quantum 2 (2018), p. 79. https://doi.org/10.22331/q-2018-08-06-79.
https://doi.org/10.22331/q-2018-08-06-79
[2] Jens Eisert et al. “Quantum certification and benchmarking”. In: Nature Reviews Physics 2 (7 2020), pp. 382–390. https://doi.org/10.1038/s42254-020-0186-4.
https://doi.org/10.1038/s42254-020-0186-4
[3] G. Lindblad. “On the generators of quantum dynamical semigroups”. In: Comm. Math. Phys. 48.2 (1976), pp. 119–130. https://doi.org/10.1007/BF01608499.
https://doi.org/10.1007/BF01608499
[4] Vittorio Gorini, Andrzej Kossakowski, and E. C. G. Sudarshan. “Completely positive dynamical semigroups of N-level systems”. In: Journal of Mathematical Physics 17.5 (1976), pp. 821–825. https://doi.org/10.1063/1.522979.
https://doi.org/10.1063/1.522979
[5] Barbara M. Terhal and Guido Burkard. “Fault-tolerant quantum computation for local non-Markovian noise”. In: Physical Review A 71.1 (2005). https://doi.org/10.1103/physreva.71.012336.
https://doi.org/10.1103/physreva.71.012336
[6] Dorit Aharonov, Alexei Kitaev, and John Preskill. “Fault-Tolerant Quantum Computation with Long-Range Correlated Noise”. In: Physical Review Letters 96.5 (2006). https://doi.org/10.1103/physrevlett.96.050504.
https://doi.org/10.1103/physrevlett.96.050504
[7] Hui Khoon Ng and John Preskill. “Fault-tolerant quantum computation versus Gaussian noise”. In: Physical Review A 79.3 (2009). https://doi.org/10.1103/physreva.79.032318.
https://doi.org/10.1103/physreva.79.032318
[8] M. M. Wolf, J. Eisert, T. S. Cubitt, and J. I. Cirac. “Assessing Non-Markovian Quantum Dynamics”. In: Phys. Rev. Lett. 101 (15 2008), p. 150402. https://doi.org/10.1103/PhysRevLett.101.150402.
https://doi.org/10.1103/PhysRevLett.101.150402
[9] G. W. Stewart and Ji-guang Sun. Matrix Perturbation Theory. Academic Press, 1990.
[10] https://github.com/quantumlib/Cirq.
https://github.com/quantumlib/Cirq
[11] Ángel Rivas, Susana F Huelga, and Martin B Plenio. “Quantum non-Markovianity: characterization, quantification and detection”. In: Reports on Progress in Physics 77.9 (2014), p. 094001. https://doi.org/10.1088/0034-4885/77/9/094001.
https://doi.org/10.1088/0034-4885/77/9/094001
[12] Carole Addis, Bogna Bylicka, Dariusz Chruscinski, and Sabrina Maniscalco. “Comparative study of non-Markovianity measures in exactly solvable one- and two-qubit models”. In: Phys. Rev. A 90 (5 2014), p. 052103. https://doi.org/10.1103/PhysRevA.90.052103.
https://doi.org/10.1103/PhysRevA.90.052103
[13] Li Li, Michael J.W. Hall, and Howard M. Wiseman. “Concepts of quantum non-Markovianity: A hierarchy”. In: Physics Reports 759 (2018). Concepts of quantum non-Markovianity: A hierarchy, pp. 1 –51. https://doi.org/10.1016/j.physrep.2018.07.001.
https://doi.org/10.1016/j.physrep.2018.07.001
[14] Dariusz Chruscinski and Sabrina Maniscalco. “Degree of Non-Markovianity of Quantum Evolution”. In: Phys. Rev. Lett. 112 (12 2014), p. 120404. https://doi.org/10.1103/PhysRevLett.112.120404.
https://doi.org/10.1103/PhysRevLett.112.120404
[15] Michael M. Wolf and J. Ignacio Cirac. “Dividing Quantum Channels”. In: Communications in Mathematical Physics 279 (1 2008), pp. 147–168. https://doi.org/10.1007/s00220-008-0411-y.
https://doi.org/10.1007/s00220-008-0411-y
[16] S. C. Hou, X. X. Yi, S. X. Yu, and C. H. Oh. “Alternative non-Markovianity measure by divisibility of dynamical maps”. In: Phys. Rev. A 83 (6 2011), p. 062115. https://doi.org/10.1103/PhysRevA.83.062115.
https://doi.org/10.1103/PhysRevA.83.062115
[17] Simon Milz, M. S. Kim, Felix A. Pollock, and Kavan Modi. “Completely Positive Divisibility Does Not Mean Markovianity”. In: Phys. Rev. Lett. 123 (4 2019), p. 040401. https://doi.org/10.1103/PhysRevLett.123.040401.
https://doi.org/10.1103/PhysRevLett.123.040401
[18] Toby Cubitt, Jens Eisert, and Michael Wolf. “The Complexity of Relating Quantum Channels to Master Equations”. In: Communications in Mathematical Physics 310 (2 2009), pp. 383–418. https://doi.org/10.1007/s00220-011-1402-y.
https://doi.org/10.1007/s00220-011-1402-y
[19] Johannes Bausch and Toby Cubitt. “The complexity of divisibility”. In: Linear Algebra and its Applications 504 (2016), pp. 64–107. https://doi.org/10.1016/j.laa.2016.03.041.
https://doi.org/10.1016/j.laa.2016.03.041
[20] Ángel Rivas, Susana F. Huelga, and Martin B. Plenio. “Entanglement and Non-Markovianity of Quantum Evolutions”. In: Physical Review Letters 105.5 (2010). https://doi.org/10.1103/physrevlett.105.050403.
https://doi.org/10.1103/physrevlett.105.050403
[21] Kang-Da Wu et al. “Detecting non-Markovianity via quantified coherence: theory and experiments”. In: npj Quantum Information 6 (1 2020), p. 55. https://doi.org/10.1038/s41534-020-0283-3.
https://doi.org/10.1038/s41534-020-0283-3
[22] A. R. Usha Devi, A. K. Rajagopal, and Sudha. “Open-system quantum dynamics with correlated initial states, not completely positive maps, and non-Markovianity”. In: Phys. Rev. A 83 (2 2011), p. 022109. https://doi.org/10.1103/PhysRevA.83.022109.
https://doi.org/10.1103/PhysRevA.83.022109
[23] Shunlong Luo, Shuangshuang Fu, and Hongting Song. “Quantifying non-Markovianity via correlations”. In: Phys. Rev. A 86 (4 2012), p. 044101. https://doi.org/10.1103/PhysRevA.86.044101.
https://doi.org/10.1103/PhysRevA.86.044101
[24] Elsi-Mari Laine, Jyrki Piilo, and Heinz-Peter Breuer. “Measure for the non-Markovianity of quantum processes”. In: Physical Review A 81.6 (2010). https://doi.org/10.1103/physreva.81.062115.
https://doi.org/10.1103/physreva.81.062115
[25] Xiao-Ming Lu, Xiaoguang Wang, and C. P. Sun. “Quantum Fisher information flow and non-Markovian processes of open systems”. In: Phys. Rev. A 82 (4 2010), p. 042103. https://doi.org/10.1103/PhysRevA.82.042103.
https://doi.org/10.1103/PhysRevA.82.042103
[26] Heinz-Peter Breuer, Elsi-Mari Laine, and Jyrki Piilo. “Measure for the Degree of Non-Markovian Behavior of Quantum Processes in Open Systems”. In: Physical Review Letters 103.21 (2009). https://doi.org/10.1103/physrevlett.103.210401.
https://doi.org/10.1103/physrevlett.103.210401
[27] Bogna Bylicka, Dariusz Chruscinski, and Sabrina Maniscalco. Non-Markovianity as a Resource for Quantum Technologies. 2013. arXiv: 1301.2585 [quant-ph].
arXiv:1301.2585
[28] Salvatore Lorenzo, Francesco Plastina, and Mauro Paternostro. “Geometrical characterization of non-Markovianity”. In: Phys. Rev. A 88 (2 2013), p. 020102. https://doi.org/10.1103/PhysRevA.88.020102.
https://doi.org/10.1103/PhysRevA.88.020102
[29] Felix A. Pollock, César Rodríguez-Rosario, Thomas Frauenheim, Mauro Paternostro, and Kavan Modi. “Operational Markov Condition for Quantum Processes”. In: Phys. Rev. Lett. 120 (4 2018), p. 040405. https://doi.org/10.1103/PhysRevLett.120.040405.
https://doi.org/10.1103/PhysRevLett.120.040405
[30] Kade Head-Marsden, Stefan Krastanov, David A. Mazziotti, and Prineha Narang. “Capturing non-Markovian dynamics on near-term quantum computers”. In: Phys. Rev. Research 3 (1 2021), p. 013182. https://doi.org/10.1103/PhysRevResearch.3.013182.
https://doi.org/10.1103/PhysRevResearch.3.013182
[31] Murphy Yuezhen Niu et al. Learning Non-Markovian Quantum Noise from Moire-Enhanced Swap Spectroscopy with Deep Evolutionary Algorithm. 2019. arXiv: 1912.04368 [quant-ph].
arXiv:1912.04368
[32] I. A. Luchnikov, S. V. Vintskevich, D. A. Grigoriev, and S. N. Filippov. “Machine Learning Non-Markovian Quantum Dynamics”. In: Physical Review Letters 124.14 (2020). https://doi.org/10.1103/physrevlett.124.140502.
https://doi.org/10.1103/physrevlett.124.140502
[33] I. A. Luchnikov et al. Probing non-Markovian quantum dynamics with data-driven analysis: Beyond ‘black-box’ machine learning models. Phys. Rev. Research 4, 043002, 2022. [quant-ph].
https://doi.org/10.1103/PhysRevResearch.4.043002
[34] Stephen Boyd and Lieven Vandenberghe. Convex Optimization. Cambridge University Press, 2004. https://doi.org/10.1017/CBO9780511804441.
https://doi.org/10.1017/CBO9780511804441
[35] Steven Diamond and Stephen Boyd. “CVXPY: A Python-embedded modeling language for convex optimization”. In: Journal of Machine Learning Research 17.83 (2016), pp. 1–5.
[36] Akshay Agrawal, Robin Verschueren, Steven Diamond, and Stephen Boyd. “A rewriting system for convex optimization problems”. In: Journal of Control and Decision 5.1 (2018), pp. 42–60.
[37] E. Davies. “Embeddable Markov Matrices”. In: Electron. J. Probab. 15 (2010), pp. 1474–1486. https://doi.org/10.1214/EJP.v15-733.
https://doi.org/10.1214/EJP.v15-733
[38] Kamil Korzekwa and Matteo Lostaglio. “Quantum Advantage in Simulating Stochastic Processes”. In: Phys. Rev. X 11 (2 2021), p. 021019. https://doi.org/10.1103/PhysRevX.11.021019.
https://doi.org/10.1103/PhysRevX.11.021019
[39] David E. Evans. “Conditionally completely positive maps on operator algebras”. In: The Quarterly Journal of Mathematics 28.3 (1977), pp. 271–283. https://doi.org/10.1093/qmath/28.3.271.
https://doi.org/10.1093/qmath/28.3.271
[40] Jyrki Piilo, Sabrina Maniscalco, Kari Härkönen, and Kalle-Antti Suominen. “Non-Markovian Quantum Jumps”. In: Phys. Rev. Lett. 100 (18 2008), p. 180402. https://doi.org/10.1103/PhysRevLett.100.180402.
https://doi.org/10.1103/PhysRevLett.100.180402
[41] https://gitlab.com/TamaraKohler/non-markovianity.
https://gitlab.com/TamaraKohler/non-markovianity.
[42] Z. Hradil. “Quantum-state estimation”. In: Phys. Rev. A 55 (3 1997), R1561–R1564. https://doi.org/10.1103/PhysRevA.55.R1561.
https://doi.org/10.1103/PhysRevA.55.R1561
[43] Daniel F. V. James, Paul G. Kwiat, William J. Munro, and Andrew G. White. “Measurement of qubits”. In: Phys. Rev. A 64 (5 2001), p. 052312. https://doi.org/10.1103/PhysRevA.64.052312.
https://doi.org/10.1103/PhysRevA.64.052312
[44] Robin Blume-Kohout. “Optimal, reliable estimation of quantum states”. In: New Journal of Physics 12.4 (2010), p. 043034. https://doi.org/10.1088/1367-2630/12/4/043034.
https://doi.org/10.1088/1367-2630/12/4/043034
[45] V. I. Danilov and V. V. Shokurov. Algebraic Geometry I. Algebraic Curves, Algebraic Manifolds and Schemes. Vol. 23. Springer-Verlag Berlin Heidelberg, 1994. https://doi.org/10.1007/978-3-642-57878-6.
https://doi.org/10.1007/978-3-642-57878-6
[46] S. H. Weintraub. Jordan Canonical Form: Theory and Practice. Synthesis Lectures on Mathematics and Statistics. Morgan and Claypool Publishers, 2009. https://doi.org/10.2200/S00218ED1V01Y200908MAS006.
https://doi.org/10.2200/S00218ED1V01Y200908MAS006
[47] Erika Andersson, James D. Cresser, and Michael J. W. Hall. “Finding the Kraus decomposition from a master equation and vice versa”. In: Journal of Modern Optics 54.12 (2007), pp. 1695–1716. https://doi.org/10.1080/09500340701352581.
https://doi.org/10.1080/09500340701352581
[48] Gabriel O. Samach et al. Lindblad Tomography of a Superconducting Quantum Processor. Phys. Rev. Applied 18, 064056, 2022. [quant-ph].
https://doi.org/10.1103/PhysRevApplied.18.064056
[49] Tosio Kato. Perturbation theory for linear operators. Vol. 132. Springer-Verlag Berlin Heidelberg, 1995. https://doi.org/10.1007/978-3-642-66282-9.
https://doi.org/10.1007/978-3-642-66282-9
[50] D. J. Hartfiel. “Dense Sets of Diagonalizable Matrices”. In: Proceedings of the American Mathematical Society 123.6 (1995), pp. 1669–1672.
[51] David Pérez-García, Michael M. Wolf, Denes Petz, and Mary Beth Ruskai. “Contractivity of positive and trace-preserving maps under Lp norms”. In: Journal of Mathematical Physics 47.8 (2006), p. 083506. https://doi.org/10.1063/1.2218675.
https://doi.org/10.1063/1.2218675
[52] Alexander Schnell, André Eckardt, and Sergey Denisov. “Is there a Floquet Lindbladian?” In: Phys. Rev. B 101 (10 2020), p. 100301. https://doi.org/10.1103/PhysRevB.101.100301.
https://doi.org/10.1103/PhysRevB.101.100301
[53] Alexander Schnell, Sergey Denisov, and André Eckardt. “High-frequency expansions for time-periodic Lindblad generators”. In: Phys. Rev. B 104 (16 2021), p. 165414. https://doi.org/10.1103/PhysRevB.104.165414.
https://doi.org/10.1103/PhysRevB.104.165414
[54] Leonid Khachiyan and Lorant Porkolab. “Computing integral points in convex semi-algebraic sets”. In: Proceedings 38th Annual Symposium on Foundations of Computer Science. IEEE. 1997, pp. 162–171.
[55] John E. Mitchell. “Integer Programming: Branch and Cut Algorithms”. In: Encyclopedia of Optimization. Ed. by Christodoulos A. Floudas and Panos M. Pardalos. Boston, MA: Springer US, 2009, pp. 1643–1650. https://doi.org/10.1007/978-0-387-74759-0287.
https://doi.org/10.1007/978-0-387-74759-0_287
Cited by
[1] Christiane P. Koch, Ugo Boscain, Tommaso Calarco, Gunther Dirr, Stefan Filipp, Steffen J. Glaser, Ronnie Kosloff, Simone Montangero, Thomas Schulte-Herbrüggen, Dominique Sugny, and Frank K. Wilhelm, "Quantum optimal control in quantum technologies. Strategic report on current status, visions and goals for research in Europe", arXiv:2205.12110, (2022).
[2] Ryan Levy, Di Luo, and Bryan K. Clark, "Classical Shadows for Quantum Process Tomography on Near-term Quantum Computers", arXiv:2110.02965, (2021).
[3] Dominik Hangleiter, Ingo Roth, Jens Eisert, and Pedram Roushan, "Precise Hamiltonian identification of a superconducting quantum processor", arXiv:2108.08319, (2021).
[4] Gabriel O. Samach, Ami Greene, Johannes Borregaard, Matthias Christandl, Joseph Barreto, David K. Kim, Christopher M. McNally, Alexander Melville, Bethany M. Niedzielski, Youngkyu Sung, Danna Rosenberg, Mollie E. Schwartz, Jonilyn L. Yoder, Terry P. Orlando, Joel I. -Jan Wang, Simon Gustavsson, Morten Kjaergaard, and William D. Oliver, "Lindblad Tomography of a Superconducting Quantum Processor", Physical Review Applied 18 6, 064056 (2022).
[5] Miha Papič and Inés de Vega, "Neural-network-based qubit-environment characterization", Physical Review A 105 2, 022605 (2022).
[6] James Sud, Jeffrey Marshall, Zhihui Wang, Eleanor Rieffel, and Filip A. Wudarski, "Dual-map framework for noise characterization of quantum computers", Physical Review A 106 1, 012606 (2022).
[7] Brian Doolittle, Tom Bromley, Nathan Killoran, and Eric Chitambar, "Variational Quantum Optimization of Nonlocality in Noisy Quantum Networks", arXiv:2205.02891, (2022).
[8] Markus Hasenöhrl and Matthias C. Caro, "Quantum and classical dynamical semigroups of superchannels and semicausal channels", Journal of Mathematical Physics 63 7, 072204 (2022).
[9] Emilio Onorati, Tamara Kohler, and Toby S. Cubitt, "Fitting time-dependent Markovian dynamics to noisy quantum channels", arXiv:2303.08936, (2023).
The above citations are from SAO/NASA ADS (last updated successfully 2023-12-05 14:26:01). The list may be incomplete as not all publishers provide suitable and complete citation data.
Could not fetch Crossref cited-by data during last attempt 2023-12-05 14:25:59: Could not fetch cited-by data for 10.22331/q-2023-12-05-1197 from Crossref. This is normal if the DOI was registered recently.
This Paper is published in Quantum under the Creative Commons Attribution 4.0 International (CC BY 4.0) license. Copyright remains with the original copyright holders such as the authors or their institutions.
- SEO Powered Content & PR Distribution. Get Amplified Today.
- PlatoData.Network Vertical Generative Ai. Empower Yourself. Access Here.
- PlatoAiStream. Web3 Intelligence. Knowledge Amplified. Access Here.
- PlatoESG. Carbon, CleanTech, Energy, Environment, Solar, Waste Management. Access Here.
- PlatoHealth. Biotech and Clinical Trials Intelligence. Access Here.
- Source: https://quantum-journal.org/papers/q-2023-12-05-1197/
- :is
- :not
- ][p
- 001
- 01
- 07
- 1
- 10
- 100
- 11
- 12
- 120
- 13
- 14
- 15%
- 16
- 17
- 19
- 1994
- 1995
- 20
- 2001
- 2005
- 2006
- 2008
- 2010
- 2011
- 2012
- 2013
- 2014
- 2016
- 2018
- 2019
- 2020
- 2021
- 2022
- 2023
- 22
- 23
- 24
- 25
- 26
- 27
- 28
- 29
- 30
- 31
- 32
- 33
- 35%
- 36
- 39
- 40
- 41
- 43
- 46
- 49
- 50
- 51
- 52
- 53
- 54
- 7
- 77
- 8
- 9
- 90
- a
- above
- ABSTRACT
- academic
- access
- accurate
- achieving
- addition
- ADvantage
- affiliations
- Aid
- AL
- Alexander
- algorithm
- algorithms
- All
- American
- analyse
- analysis
- Analytical
- and
- Andrew
- annual
- applications
- applied
- approximates
- ARE
- AS
- attempt
- author
- authors
- b
- based
- BE
- behavior
- behaviour
- being
- Benchmark
- berlin
- BEST
- beth
- Beyond
- boston
- both
- Branch
- Break
- Brian
- Bryan
- by
- cambridge
- CAN
- carried
- carrying
- case
- certain
- Certification
- Channel
- channels
- Christopher
- College
- comm
- comment
- Commons
- Communications
- complete
- completely
- complexity
- computation
- computer
- computer science
- computers
- computing
- concepts
- condition
- consistent
- control
- Convex
- copyright
- correlated
- could
- counterparts
- critical
- crucial
- Current
- Currently
- Cut
- Daniel
- data
- data-driven
- David
- deal
- decision
- deep
- Degree
- Den
- Department
- description
- designing
- develop
- device
- Devices
- Diamond
- difficult
- discuss
- does
- during
- dynamics
- e
- E&T
- ed
- effects
- efficient
- Engineers
- equations
- Era
- eric
- Erika
- error
- Errors
- especially
- Ether (ETH)
- Europe
- evaluate
- evolution
- exactly
- examples
- experimental
- experiments
- far
- faster
- fitting
- flow
- For
- form
- Foundations
- Framework
- frank
- from
- fu
- full
- fundamental
- generated
- generator
- generators
- geometry
- given
- Goals
- Hall
- harder
- Hardware
- harvard
- help
- hierarchy
- holders
- howard
- However
- HTTPS
- i
- Identification
- IEEE
- if
- image
- implementation
- in
- information
- initial
- institutions
- integral
- interesting
- International
- IT
- ITS
- james
- JavaScript
- jeffrey
- John
- Jordan
- journal
- jpg
- Kim
- knowledge
- Koch
- language
- large-scale
- Last
- learning
- Leave
- lectures
- levy
- li
- License
- List
- local
- London
- LP
- machine
- machine learning
- Main
- Maps
- Martin
- master
- Match
- materials
- math
- mathematical
- mathematics
- Matrix
- matthias
- max-width
- May..
- mean
- meaningful
- measure
- measured
- measures
- methods
- Michael
- mitigating
- modeling
- models
- Modern
- Month
- more
- Morgan
- most
- Nature
- networks
- New
- no
- Noise
- normal
- obstacles
- of
- offer
- oh
- oliver
- on
- ONE
- open
- operator
- operators
- optics
- optimal
- optimization
- or
- original
- Orlando
- our
- out
- output
- outputs
- pages
- Paper
- part
- particularly
- Paul
- People
- physical
- Physics
- platform
- plato
- Plato Data Intelligence
- PlatoData
- points
- positive
- possibility
- practice
- precise
- Precision
- presence
- press
- prevalent
- problems
- Proceedings
- process
- processes
- Processor
- Programming
- Progress
- provide
- published
- publisher
- publishers
- Python
- quantification
- quantitative
- Quantum
- quantum algorithms
- quantum computers
- quantum computing
- quantum information
- quantum networks
- quickly
- R
- range
- reach
- recently
- references
- registered
- reliable
- remains
- report
- Reports
- research
- resistant
- resource
- Results
- returns
- review
- Reviews
- rewriting
- rigorous
- Robin
- Ryan
- s
- schemes
- Science
- set
- Sets
- show
- Simon
- Society
- song
- Spectroscopy
- standard
- States
- statistics
- Status
- stefan
- Stephen
- Steps
- steven
- stewart
- Strategic
- strategies
- Study
- succeed
- Successfully
- such
- suitable
- Sun
- susceptible
- swap
- Symposium
- synthesis
- system
- T
- Take
- taken
- tamara
- tasks
- techniques
- Technologies
- terms
- than
- that
- The
- their
- then
- theory
- There.
- therefore
- These
- this
- Title
- to
- tom
- tomography
- type
- types
- under
- underlying
- understand
- university
- unknown
- updated
- URL
- us
- using
- Values
- Versus
- very
- via
- vice
- visions
- volume
- W
- wang
- want
- was
- we
- which
- white
- will
- william
- with
- within
- Wolf
- Work
- wu
- X
- year
- zephyrnet