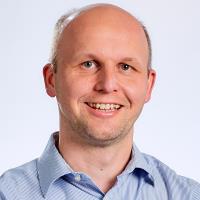
Last year I celebrated two decades of immersion in IT, specifically within the Financial Services sector. During this period I have been a witness to remarkable transformations in banking and technology. The emergence of Fintech companies and their customer-centric
approach, along with significant advancements in software engineering like Agile methodologies, microservices, and cloud computing, have reshaped the landscape. Yet, intriguingly, the back-office operations of many financial service companies have remained
relatively static over these years, still grappling with 手動エンコーディング、反復的なタスク、Excel への依存度の高さ.
金融サービス部門における特に手動でありながら自動化可能なプロセスは、次のとおりです。 マッチングと調整. This process arises in various forms, i.e. from identifying and addressing discrepancies (typically occurring due to issues
or gaps with the integrations) in master-slave integrations to correcting or removing duplicates and semi-automated updates of operational systems with data from external sources.
利用可能であるにもかかわらず、 洗練されたソフトウェア (e.g. FIS IntelliMatch, Calypso Confirmation Matching, Misys CMS, Temenos T24 Confirmation Matching…) for specific reconciliation tasks, such as payment and trade confirmation matching
(often based on SWIFT messages), the 照合タスクの大部分はカスタムまたは手動のソリューションに依存することがよくあります, including Excel or even paper-based methods. Very often automation is also not pertinent, as matching is often involved in one-time actions
like marketing campaigns, data clean-ups, alignment with partners…
より良い調整を理解するには、次のことが必要です そのコンポーネントを分析するすなわち、
-
それはで始まる 比較可能にするために異種データセットを収集および変換する. This consists of recuperating 2 data sets, which can be delivered in different formats, different structures, different scopes and with different names
or enumerations. The data needs to be transformed to make them comparable and loaded into the same tool (e.g. a database or Excel), so that they can be easily compared. -
次のステップでは、 正確なマッチングアルゴリズム. This can be a simple unique key, but it can also a combination of multiple attributes (composite key), a hierarchical rule (i.e. match first on key 1, if no match try on key 2…) or
a fuzzy rule (if key of data set 1 resembles key of data set 2 it is a match). Defining this matching algorithm can be very complex, but it is crucial in the ability to automate the matching and reach a good output quality. -
マッチングアルゴリズムが定義されたら、次のように入力します。 比較フェーズ. For small data sets, this can be done quite simple, but for very large data sets, it can necessitate all kinds of performance optimizations (like indices, segmentation,
parallelism…) in order to execute the comparison in a reasonable time. -
最後に、 特定された矛盾は実用的な出力に変換する必要があるレポート、同僚や第三者へのコミュニケーション、修正措置(相違点を修正するためのファイル、メッセージ、または SQL ステートメントの生成など)など。
金融サービスにおけるマッチングの複雑さは多岐にわたります。 探検してみましょう いくつかの典型的な使用例 金融サービスの分野では:
-
ほとんどの銀行には 有価証券マスターファイル, describing all securities which are in position or can be traded at the bank. This file needs to be integrated with a lot of applications, but also needs to be fed by multiple data sources, like
Telekurs, Reuters, Bloomberg, Moody’s… This means a security needs to be uniquely matched. Unfortunately, there is not 1 unique identifier describing all securities. Publicly traded instruments have a commonly agreed ISIN code, but private and OTC products
like e.g. most derivatives usually do not. Banks have therefore invented internal identifiers, use fake ISIN codes (typically starting with an “X”) or use composite keys to uniquely identify the instrument (e.g. for a derivative this can be combination of
ticker of underlying security, strike price, option type and expiration date). -
リテールバンキングでは明らかに次のことが不可欠です。 特定の物理的な人物を一意に識別して照合する. However even in a developed country like Belgium, this is easier said than done. Every individual in Belgium has a National Register Number,
so this seems the obvious choice for a matching key. Unfortunately, Belgian laws restrict the usage of this number to specific use cases. Additionally this identifier is not existing for foreigners and can change over time (e.g. foreign residents receive first
a temporary National Register number which can change to a definitive, other one later or in case of gender change the National Register Number will change as well). Another option is to use the identity card number, but this is also different for foreigners
and will change every 10 years. Many banks therefore use more complex rules, like a matching based on first name, last name and birth date, but obviously this comes also with all kinds of issues, like duplicates, spelling differences and errors in the names,
use of special characters in the names… -
非常によく似た問題は、 企業、より具体的には店舗のマッチング. In Belgium, each company has a company number, which is similar to the VAT number (without the “BE” prefix), but this is again very national and 1 VAT number can
have multiple locations (e.g. multiple stores). There exists a concept of a “branch number” (“vestigingsnummer” in Dutch), but this concept is not very well known and rarely used. Similar there exists the LEI code (Legal Entity Identifier) which is a code
of a combination of 20 letters and codes, which uniquely identifies a company worldwide. Unfortunately, only large companies have requested a LEI code, so for smaller companies this is not really an option.
Again more complex matchings are often done, like a combination of VAT number, postal code and house number, but obviously this is far from being ideal. In search for a unique and commonly known identifier, the Google ID becomes also more and more in use, but
the dependency with a commercial company might also poses a big operational risk. -
もう一つの興味深い事例は、 VISAカード支払いにおけるオーソリゼーションとクリアリングメッセージの照合. Normally a unique identifier should match both messages, but due to all kinds of exception cases (e.g. offline authorizations or
incremental authorizations), this will not always be correct. Therefore a more complex rule is required, looking at several identifiers, but also to other matching criteria like acquirer ID, merchant ID, terminal ID, PAN (card number), timestamp and/or amount.
この種の照合は、事前承認の完了とその前の事前承認の照合、または払い戻しと以前の購入の照合など、他の支払いのユースケースにも当てはまります。 -
ほぼすべてのビジネスに関係する財務上のユースケースは次のとおりです。 請求書と支払いの照合. When a company issues an invoice, it needs to be able to see when the invoice can be considered as paid. This is important for the accounting, but also
to see if reminders for unpaid invoices should be sent out.
To uniquely match the payment with the invoice, in Belgium typically a structured comment is used in the payment instruction. This unique code with check digit provides a unique matching reference. Unfortunately, customers often forget to put the structured
comment or use the wrong one (e.g. copy/paste of a previous invoice). This means a company needs to have a fallback matching rule in case the unstructured comment is missing or wrong. Typically a combination of payment amount, payment date, IBAN of counterparty
and/or name of counterparty can give an alternative way to match those invoices.
ご覧のとおり、マッチングは決して簡単ではありませんが、基本的な手順を理解することでマッチングを改善することができます。 それまでのところ、制限はあるものの、Excel は依然として (手動) マッチングのための強力なツールです。 したがって、 quick reminder for everyone who wants
to do matching in Excel:
-
照合を実行する VLOOKUP。 ただし、VLOOKUP には、一致しない場合にエラーが発生したり、最初の列でしか検索できないなど、特定の制限があります。 強力な代替手段は、 XLOKUP、その
does not have these limitations. -
あなたが必要な場合 複合検索キー、複合検索キーを使用して検索データ セットに列を追加し (つまり、区切り文字として「#」などを使用してさまざまな属性を連結します)、VLOOKUP/XLOOKUP を使用してこの新しい列を検索します。
-
一部 注意点 VLOOKUP を使用する場合:
-
完全一致を保証するために、関数 VLOOKUP の最後の引数として「false」を追加することを忘れないでください。
-
Ensure that data formats are the same. E.g. a number “123” and the text “123” will not match, so it is important to convert them to the same format first. Idem for identifiers starting with leading 0’s. Often Excel will convert those to numbers, thus removing
the leading 0’s and not resulting in a match. -
Excel では 100.000 行を超えるデータ セットを使用しないでください。 データ セットが大きくなると、Excel のパフォーマンスと安定性に問題が生じます。
大規模なデータ セットで VLOOKUP を使用する場合は、計算モードを「手動」に設定すると興味深い場合があります。そうしないと、データに小さな変更を加えるたびに Excel がすべての VLOOKUP を再計算します。 -
VLOOKUP は XNUMX 番目の引数として返す列番号を持ちます。 この数値は列を追加または削除するときに動的に調整されないため、列を追加または削除するときに必ず調整してください。
-
一致するだけの場合は、式「=IF(ISERROR(VLOOKUP( 、 ,1,false),”一致しません”,”一致”)”
-
これらのトリックは次のことに役立ちます 手動マッチングを高速化しますしかし、明らかに実際の自動化は常に優れています。
金融サービスにおけるマッチングは、 多面的な挑戦, but understanding its fundamental steps is key to improving outcomes. While tools like Excel offer temporary solutions, the future lies in intelligent automation, which can significantly
streamline these processes. For those seeking to delve deeper into matching complexities or automation, leveraging advanced tools and platforms, including AI-driven solutions like ChatGPT, can provide both insights and practical solutions.
私のすべてのブログをチェックしてください https://bankloch.blogspot.com/
- SEO を活用したコンテンツと PR 配信。 今日増幅されます。
- PlatoData.Network 垂直生成 Ai。 自分自身に力を与えましょう。 こちらからアクセスしてください。
- プラトアイストリーム。 Web3 インテリジェンス。 知識増幅。 こちらからアクセスしてください。
- プラトンESG。 カーボン、 クリーンテック、 エネルギー、 環境、 太陽、 廃棄物管理。 こちらからアクセスしてください。
- プラトンヘルス。 バイオテクノロジーと臨床試験のインテリジェンス。 こちらからアクセスしてください。
- 情報源: https://www.finextra.com/blogposting/25297/the-unseen-backbone-of-banking-a-deep-dive-into-matching-and-reconciliation?utm_medium=rssfinextra&utm_source=finextrablogs
- :持っている
- :は
- :not
- $UP
- 000
- 1
- 10
- 100
- 11
- 13
- 14
- 視聴者の38%が
- 20
- 8
- a
- 能力
- できる
- 会計
- 行動
- 適応する
- 適合しました
- 加えます
- 追加
- さらに
- アドレッシング
- 高度な
- 進歩
- 再び
- 使い勝手のいい
- アルゴリズム
- アラインメント
- すべて
- ほとんど
- 沿って
- また
- 代替案
- 常に
- 量
- an
- および
- 別の
- どれか
- 適用
- アプローチ
- です
- 引数
- AS
- At
- 属性
- 承認
- 自動化する
- オートメーション
- 賃貸条件の詳細・契約費用のお見積り等について
- バックボーン
- 銀行
- バンキング
- 銀行
- ベース
- 基本
- BE
- になる
- き
- さ
- ベルギー
- より良いです
- ビッグ
- 出産
- ブログ
- ブルームバーグ
- 両言語で
- ブランチ
- ビジネス
- 焙煎が極度に未発達や過発達のコーヒーにて、クロロゲン酸の味わいへの影響は強くなり、金属を思わせる味わいと乾いたマウスフィールを感じさせます。
- by
- 計算
- キャンペーン
- 缶
- カード
- 場合
- 例
- 祝っ
- 一定
- 変化する
- 文字
- AI言語モデルを活用してコードのデバッグからデータの異常検出まで、
- チェック
- 選択
- 決済
- クラウド
- クラウドコンピューティング
- Cms
- コード
- コード
- 同僚
- コラム
- コラム
- 組み合わせ
- 来ます
- コメント
- コマーシャル
- 一般に
- 通信部
- 企業
- 会社
- 匹敵します
- 比べ
- 比較
- 完成
- 複雑な
- 複雑さ
- コンピューティング
- コンセプト
- 懸念事項
- 確認
- 見なさ
- からなる
- 変換
- 正しい
- カウンターパーティー
- 国
- 基準
- 重大な
- カスタム
- Customers
- データ
- データセット
- データセット
- データベース
- 日付
- 数十年
- 深いです
- ディープダイブ
- より深い
- 定義済みの
- 定義
- 決定的な
- 配信
- 掘り下げる
- 依存関係
- デリバティブ
- デリバティブ
- 記述
- にもかかわらず
- 発展した
- の違い
- 異なります
- 桁
- 異なる
- ダイビング
- 異なる
- do
- ありません
- 行われ
- 原因
- 複製
- 間に
- オランダ語
- 動的に
- e
- 各
- 容易
- 簡単に
- 簡単に
- 出現
- エンコーディング
- エンジニアリング
- 確保
- 入力します
- エンティティ
- エラー
- エラー
- 本質的な
- さらに
- あらゆる
- 誰も
- Excel
- 例外
- 実行します
- 既存の
- 存在
- 期限切れ
- 外部
- 実際
- 偽
- false
- 遠く
- FRBは
- File
- ファイナンシャル
- 金融サービス
- 金融業務
- Finextra
- フィンテック
- フィンテック企業
- 名
- FIS
- 修正する
- 外国の
- 形式でアーカイブしたプロジェクトを保存します.
- フォーム
- 式
- から
- function
- 基本的な
- 未来
- ギャップ
- 性別
- 世代
- 与える
- 与える
- 良い
- でログイン
- 格闘
- 持ってる
- ヘビー
- 助けます
- お家の掃除
- しかしながら
- HTTPS
- i
- ID
- 理想
- 識別子
- 識別子
- 識別する
- 識別する
- 識別
- アイデンティティ
- if
- イマージョン
- 重要
- 改善
- in
- 含めて
- インクリメンタル
- 索引
- 個人
- 洞察
- 楽器
- 楽器
- 統合された
- 統合
- インテリジェント-
- インテリジェントオートメーション
- 興味深い
- 内部
- に
- 複雑さ
- 発明された
- 請求書
- 請求書
- 関係する
- ISIN
- 問題
- IT
- ITS
- JPG
- ただ
- キー
- キー
- 種類
- 既知の
- 風景
- 大
- より大きい
- 姓
- 後で
- 法制
- 主要な
- リーガルポリシー
- 法人
- う
- 活用
- ある
- ような
- 制限
- 場所
- 探して
- たくさん
- make
- マニュアル
- 多くの
- マーケティング
- マーケティングキャンペーン
- マスター
- 一致
- マッチ
- マッチング
- 手段
- その間
- マーチャント
- メッセージ
- メッセージ
- 方法論
- メソッド
- マイクロサービス
- かもしれない
- マイナー
- 行方不明
- モード
- 他には?
- 最も
- の試合に
- しなければなりません
- my
- 名
- 名
- 国民
- 必要
- ニーズ
- 新作
- 次の
- いいえ
- 通常は
- 数
- 番号
- 明白
- 発生する
- of
- 提供
- オンライン
- 頻繁に
- on
- ONE
- の
- オペレーショナル
- 業務執行統括
- オプション
- or
- 注文
- OTC
- その他
- さもないと
- でる
- 成果
- 出力
- が
- 支払われた
- PAN
- 紙ベース
- 特に
- パーティー
- 支払い
- 実行する
- パフォーマンス
- 期間
- 物理的な
- プラットフォーム
- プラトン
- プラトンデータインテリジェンス
- プラトデータ
- ポーズ
- 位置
- 郵便
- 強力な
- 実用的
- 前
- ブランド
- 事前の
- プライベート
- 問題
- プロセス
- ラボレーション
- 製品
- 提供します
- は、大阪で
- 公然と
- 購入
- 置きます
- 品質
- 非常に
- まれに
- リーチ
- リアル
- 本当に
- 合理的な
- 受け取ります
- 和解
- 参照
- 払い戻し
- 登録
- 相対的に
- 依存
- 頼る
- 残った
- 残っている
- 顕著
- 覚えています
- リマインダー
- 除去
- 反復的な
- レポート
- 要求されました
- の提出が必要です
- 似てる
- 住民
- 制限する
- 結果として
- 小売
- リテールバンキング
- return
- ロイター通信社
- リスク
- ルール
- ルール
- 前記
- 同じ
- を検索
- セクター
- 有価証券
- セキュリティ
- を求める
- と思われる
- セグメンテーション
- 送信
- サービス
- サービス
- セッションに
- セット
- いくつかの
- すべき
- 重要
- 著しく
- 同様の
- 簡単な拡張で
- 小さい
- より小さい
- So
- ソフトウェア
- ソフトウェア工学
- ソリューション
- ソース
- 特別
- 特定の
- 特に
- 綴り
- SQL
- 安定性
- 起動
- 開始
- 文
- 手順
- ステップ
- まだ
- 店舗
- 流線
- ストライキ
- 構造化された
- 構造
- そのような
- SWIFT
- システム
- タスク
- テクノロジー
- テメノス
- 一時的
- ターミナル
- 클라우드 기반 AI/ML및 고성능 컴퓨팅을 통한 디지털 트윈의 기초 – Edward Hsu, Rescale CPO 많은 엔지니어링 중심 기업에게 클라우드는 R&D디지털 전환의 첫 단계일 뿐입니다. 클라우드 자원을 활용해 엔지니어링 팀의 제약을 해결하는 단계를 넘어, 시뮬레이션 운영을 통합하고 최적화하며, 궁극적으로는 모델 기반의 협업과 의사 결정을 지원하여 신제품을 결정할 때 데이터 기반 엔지니어링을 적용하고자 합니다. Rescale은 이러한 혁신을 돕기 위해 컴퓨팅 추천 엔진, 통합 데이터 패브릭, 메타데이터 관리 등을 개발하고 있습니다. 이번 자리를 빌려 비즈니스 경쟁력 제고를 위한 디지털 트윈 및 디지털 스레드 전략 개발 방법에 대한 인사이트를 나누고자 합니다.
- より
- それ
- 未来
- 風景
- アプリ環境に合わせて
- それら
- その後
- そこ。
- したがって、
- ボーマン
- 彼ら
- 三番
- 第三者
- この
- それらの
- 従って
- ティッカー
- 時間
- タイムスタンプ
- 〜へ
- ツール
- 豊富なツール群
- トレード
- 取引
- 変換
- 変換
- 変換
- 試します
- 2
- type
- 典型的な
- 一般的に
- 根本的な
- 理解する
- 残念ながら
- ユニーク
- 独特に
- 更新版
- us
- 使用法
- つかいます
- 使用事例
- 中古
- 通常
- さまざまな
- 付加価値税(VAT)
- 非常に
- ビザ
- ビザカード
- 欲しいです
- 望んでいる
- 仕方..
- we
- WELL
- いつ
- which
- while
- 誰
- 意志
- 以内
- 無し
- 目撃者
- ワーキング
- 間違った
- X
- 年
- 年
- まだ
- 貴社
- あなたの
- ゼファーネット