介绍
对于许多印度人来说,农业不仅仅是一份工作;这是一种生活方式。这是他们维持生计并为印度经济做出巨大贡献的手段。确定粘土、沙子和淤泥颗粒各自比例的土壤类型对于选择合适的作物和识别杂草的生长非常重要。发现深度学习在农业中的潜力。了解土壤类型和杂草检测对印度的重要性。
深入学习 是一项在各个领域都有帮助的新兴技术。深度学习已广泛应用于各种规模的智能农业,包括田间监测、田间操作、机器人技术、预测土壤、水、气候条件以及景观级土地和作物类型监测。我们可以将土壤照片输入深度学习架构,引导其学习检测特征,然后使用深度学习架构对土壤进行分类。
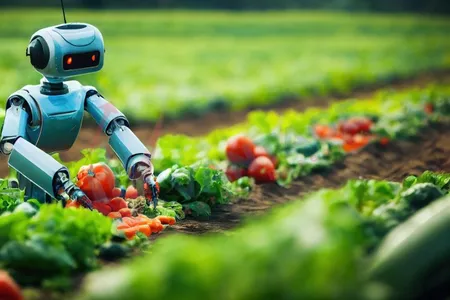
在本博客中,我们将讨论土壤在农业中的重要性。我们将使用机器学习和深度学习模型对土壤进行分类。
学习目标
- 您将了解土壤在农业中的重要性。
- 您将了解机器学习算法如何对土壤类型进行分类。
- 您将在农业中实施深度学习模型来对土壤类型进行分类。
- 探索多堆栈集成学习的概念,以提高我们预测的准确性。
这篇文章是作为 数据科学博客马拉松。
目录
土壤在农业中的作用
植物和动物排出的有机物、矿物质、气体、液体和其他物质形成了重要的土壤,是农业的基础。农业的基础在于来自植物和动物的气体、矿物质、有机物和其他物质,形成土壤系统。
印度经济纯粹依赖农业;土壤对农作物很重要,由于其肥力,土壤会导致杂草的生长。
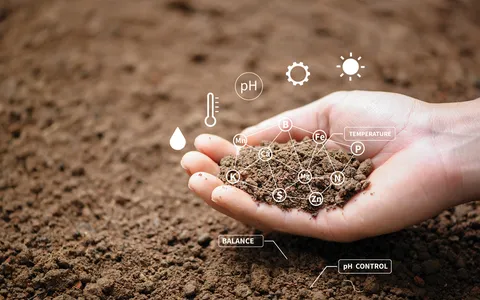
水分和温度是影响土壤中孔隙和颗粒形成的物理变量,影响根系生长、水渗透和植物出苗速度。
但土壤主要含有沙子和粘土颗粒。在勘探现场普遍存在的土壤颗粒中,粘土含量丰富。粘土颗粒在表面的可用性是由于提供了丰富的营养。泥炭和壤土几乎不存在。粘土型土壤之间宽敞,水分被保留在其中。
数据集
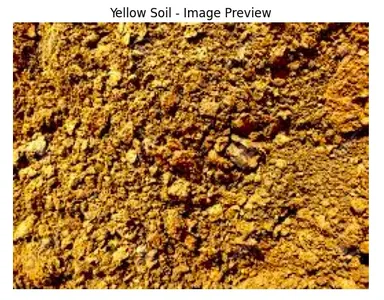
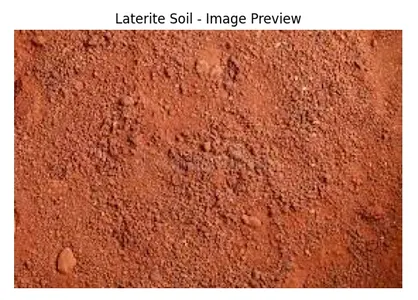
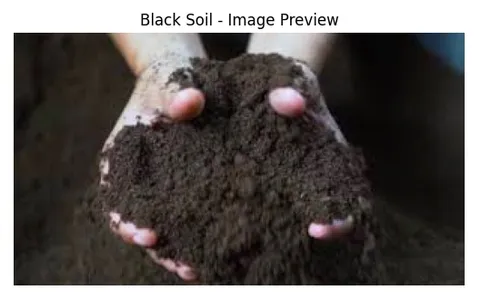
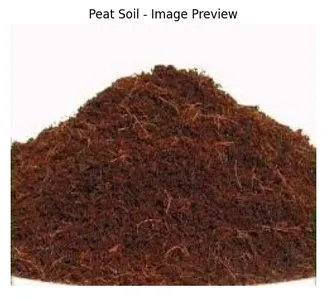
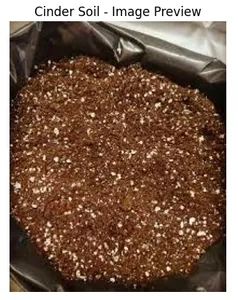
特征提取是构建良好深度学习模型的主要步骤之一。确定构建机器学习算法可能必需的特征非常重要。我们将使用 马哈塔斯 用于提取 Haralick 特征的库,这些特征具有图像的空间和纹理信息。
我们将使用 skimage 库将图像转换为灰度并提取对目标检测有用的梯度直方图(HOG)特征。最后,我们将特征值连接到一个数组中,然后将它们用于机器学习和深度学习算法。

import mahotas as mh
from skimage import color, feature, io
import numpy as np
# Function to extract features from an image
def extract_features(image_path):
img = io.imread(image_path)
gray_img = color.rgb2gray(img) # Converting image to grayscale
# Converting the grayscale image to integer type
gray_img_int = (gray_img * 255).astype(np.uint8)
# Extracting Haralick features using mahotas
haralick_features = mh.features.haralick(gray_img_int).mean(axis=0)
# Extracting Histogram of Gradients (HOG) features
hog_features, _ = feature.hog(gray_img, visualize=True)
# Printing the first few elements of each feature array
print("Haralick Features:", haralick_features[:5])
print("HOG Features:", hog_features[:5])
# Concatenating the features into a single array
all_features = np.concatenate((haralick_features, hog_features))
return all_features
image_path = '/kaggle/input/soil-classification-dataset/Soil-Dataset/Yellow Soil/20.jpg'
features = extract_features(image_path)
print("Extracted Features:", features)

土壤分类中的机器学习算法
现在,让我们使用从 Kaggle 获得的土壤图像构建一个机器学习模型。
首先,我们将导入所有库,然后构建一个名为的函数 提取特征 从图像中提取特征。然后导入并处理图像,其中包括转换为灰度,然后我们获得这些特征。然后,在为每个图像提取特征后,使用以下方法对标签进行编码 标签编码器。
import os
import numpy as np
import mahotas as mh
from sklearn.model_selection import train_test_split
from sklearn.preprocessing import LabelEncoder
from sklearn.ensemble import RandomForestClassifier, StackingClassifier
from sklearn.linear_model import LogisticRegression
from sklearn.svm import SVC
from sklearn.neighbors import KNeighborsClassifier
from sklearn.naive_bayes import GaussianNB
from sklearn.metrics import accuracy_score, classification_report
from skimage import color, feature, io
# Function to extract features from an image
def extract_features(image_path):
img = io.imread(image_path)
gray_img = color.rgb2gray(img) # Converting image to grayscale
gray_img_int = (gray_img * 255).astype(np.uint8)
haralick_features = mh.features.haralick(gray_img_int).mean(axis=0)
hog_features, _ = feature.hog(gray_img, visualize=True)
hog_features_flat = hog_features.flatten() # Flattening the HOG features
# Ensuring both sets of features have the same length
hog_features_flat = hog_features_flat[:haralick_features.shape[0]]
return np.concatenate((haralick_features, hog_features_flat))
data_dir = "/kaggle/input/soil-classification-dataset/Soil-Dataset"
image_paths = []
labels = []
class_indices = {'Black Soil': 0, 'Cinder Soil': 1, 'Laterite Soil': 2,
'Peat Soil': 3, 'Yellow Soil': 4}
for soil_class, class_index in class_indices.items():
class_dir = os.path.join(data_dir, soil_class)
class_images = [os.path.join(class_dir, image) for image in os.listdir(class_dir)]
image_paths.extend(class_images)
labels.extend([class_index] * len(class_images))
# Extracting features from images
X = [extract_features(image_path) for image_path in image_paths]
# Encoding labels
le = LabelEncoder()
y = le.fit_transform(labels)
# Splitting the data into training and testing sets
X_train, X_test, y_train, y_test = train_test_split(X, y, test_size=0.2, random_state=42)
# Initializing and training a Random Forest Classifier
rf_classifier = RandomForestClassifier(n_estimators=100, random_state=42)
rf_classifier.fit(X_train, y_train)
# Making predictions
y_pred_rf = rf_classifier.predict(X_test)
# Evaluating the Random Forest model
accuracy_rf = accuracy_score(y_test, y_pred_rf)
report_rf = classification_report(y_test, y_pred_rf)
print("Random Forest Classifier:")
print("Accuracy:", accuracy_rf)
print("Classification Report:n", report_rf)
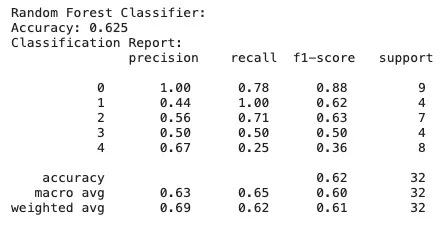
深度神经网络
它的工作原理基于计算单元和神经元的数量。每个神经元接受输入并提供输出。它用于提高准确性并做出更好的预测,而机器学习算法依赖于解释数据,并根据它们做出决策。
另请阅读: 深度学习和神经网络入门指南
现在,让我们构建使用 Keras 的 Sequential API 定义的模型。该模型将具有 Conv2D 卷积层、MaxPooling2D、扁平化层 Flatten 和密集层 Dense。
最后,使用以下命令编译模型 Adam 优化器和分类交叉熵损失。
import tensorflow as tf
from tensorflow.keras.preprocessing import image_dataset_from_directory
data_dir = "/kaggle/input/soil-classification-dataset/Soil-Dataset"
# Setting up data generators
batch_size = 32
image_size = (224, 224)
# Using image_dataset_from_directory to load and preprocess the images
train_dataset = image_dataset_from_directory(
data_dir,
labels='inferred',
label_mode='categorical',
validation_split=0.2,
subset='training',
seed=42,
image_size=image_size,
batch_size=batch_size,
)
validation_dataset = image_dataset_from_directory(
data_dir,
labels='inferred',
label_mode='categorical',
validation_split=0.2,
subset='validation',
seed=42,
image_size=image_size,
batch_size=batch_size,
)
# Displaying the class indices
print("Class indices:", train_dataset.class_names)
model = tf.keras.Sequential([
tf.keras.layers.Conv2D(32, (3, 3), activation='relu', input_shape=(224, 224, 3)),
tf.keras.layers.MaxPooling2D(2, 2),
tf.keras.layers.Flatten(),
tf.keras.layers.Dense(64, activation='relu'),
tf.keras.layers.Dense(len(train_dataset.class_names), activation='softmax')
])
model.compile(optimizer='adam', loss='categorical_crossentropy', metrics=['accuracy'])
# Training the model
epochs = 10
history = model.fit(train_dataset, epochs=epochs, validation_data=validation_dataset)
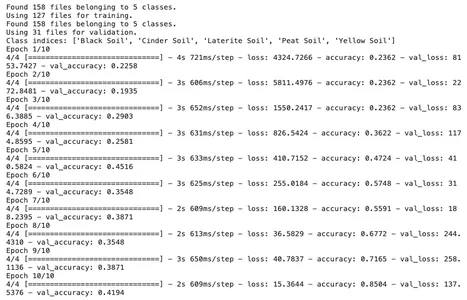
import numpy as np
from tensorflow.keras.preprocessing import image
# Function to load and preprocess an image for prediction
def load_and_preprocess_image(img_path):
img = image.load_img(img_path, target_size=image_size)
img_array = image.img_to_array(img)
img_array = np.expand_dims(img_array, axis=0)
img_array /= 255.0
return img_array
image_path = '/kaggle/input/soil-classification-dataset/Soil-Dataset/Peat Soil/13.jpg'
new_image = load_and_preprocess_image(image_path)
# Making predictions
predictions = model.predict(new_image)
predicted_class = np.argmax(predictions[0])
# Getting the class label based on the class indices
class_labels = {0: 'Black Soil', 1: 'Cinder Soil', 2: 'Laterite Soil',
3: 'Peat Soil', 4: 'Yellow Soil'}
predicted_label = class_labels[predicted_class]
# Displaying the prediction
print("Predicted Class:", predicted_class)
print("Predicted Label:", predicted_label)

正如您所看到的,预测的类别为 0,即黑土。因此,我们的模型正确地对土壤类型进行了分类。
提出的多堆栈集成学习模型架构
堆叠分类器 使用 baseClassifiers 和 a 进行初始化 逻辑回归 元分类器 最终估计器。这结合了基本分类器的输出来做出最终预测。然后,经过训练和预测,计算准确率。
base_classifiers = [
('rf', RandomForestClassifier(n_estimators=100, random_state=42)),
('knn', KNeighborsClassifier(n_neighbors=5)),
('svm', SVC(kernel='rbf', C=1.0, probability=True)),
('nb', GaussianNB())
]
# Initializing the stacking classifier with a logistic regression meta-classifier
stacking_classifier = StackingClassifier(estimators=base_classifiers,
final_estimator=LogisticRegression())
# Training the stacking classifier
stacking_classifier.fit(X_train, y_train)
# Making predictions with Stacking Classifier
y_pred_stacking = stacking_classifier.predict(X_test)
# Evaluating the Stacking Classifier model
accuracy_stacking = accuracy_score(y_test, y_pred_stacking)
report_stacking = classification_report(y_test, y_pred_stacking)
print("nStacking Classifier:")
print("Accuracy:", accuracy_stacking)
print("Classification Report:n", report_stacking)
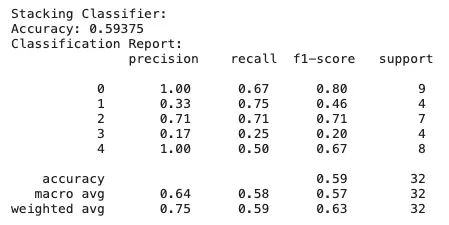
结论
土壤是获得好作物的重要因素。了解生产特定作物所需的土壤类型非常重要。因此,对土壤类型进行分类变得很重要。由于手动对土壤类型进行分类是一项耗时的任务,因此使用深度学习模型对其进行分类变得很容易。有许多机器学习模型和深度学习模型来实现这个问题陈述。选择最好的一个取决于数据集中存在的数据的质量和数量以及手头的问题陈述。选择最佳算法的另一种方法是评估每个算法。我们可以通过测量准确度来做到这一点,即测量它们对土壤进行正确分类的程度。最后,我们实现了一个 Multi-Stacking 集成模型,使用多个模型来构建最佳模型。
关键精华
- 为了有效地选择作物,人们应该完全了解土壤。
- 农业中的深度学习是一种强大的工具,从预测植物病害到土壤类型和水需求。
- 我们进行了特征提取,从土壤图像中获取特征。
- 在本博客中,我们探索了用于对土壤进行分类的机器学习和深度学习模型,以及用于提高准确性的多堆栈集成模型。
常见问题
答:这对于选择合适的作物和识别杂草生长非常重要。
A. 考虑的特征包括沙子、粘土、淤泥、泥炭和壤土。
答:深度学习允许模型做出智能决策,而传统机器学习通过解释数据来做出决策。
A. Multi-Stacking 集成模型提高了土壤类型分类的准确性。
本文中显示的媒体不属于 Analytics Vidhya 所有,其使用由作者自行决定。
相关
- :具有
- :是
- :不是
- $UP
- 1
- 10
- 13
- 224
- 32
- 33
- 43
- 46
- 51
- 7
- 9
- a
- 接受
- 横过
- Adam
- 影响
- 后
- 农业
- 算法
- 算法
- 所有类型
- 允许
- 烟雨
- 量
- an
- 分析
- 分析维迪亚
- 和
- 动物
- 另一个
- API
- 应用的
- 架构
- 保健
- 排列
- 刊文
- AS
- At
- 可用性
- 可使用
- 基于
- BE
- 成为
- 很
- 最佳
- 更好
- 之间
- 黑色
- 博客
- 博客马拉松
- 都
- 建立
- 建筑物
- by
- 计算
- CAN
- 选择
- 程
- 分类
- 分类
- 气候
- 颜色
- 结合
- 如何
- 编译
- 完全
- 计算
- 概念
- 条件
- 考虑
- 贡献
- 兑换
- 转换
- 正确地
- 作物
- 作物
- data
- 决定
- 深
- 深入学习
- 定义
- 密
- 依靠
- 检测
- 检测
- 决心
- 研发支持
- 不同
- 通过各种方式找到
- 酌处权
- 讨论
- 疾病
- 显示
- do
- 不
- 完成
- 两
- 每
- 易
- 经济
- 有效
- element
- 分子
- 出现
- 新兴经济体的新市场。
- 新兴技术
- 编码
- 编码
- 保证
- 时代
- 醚(ETH)
- 评估
- 所有的
- 勘探
- 探讨
- 提取
- 萃取
- 专栏
- 特征
- 少数
- 部分
- 最后
- 终于
- (名字)
- 针对
- 森林
- 申请
- 训练
- 基金会
- 止
- 功能
- 发电机
- 得到
- 越来越
- 非常好
- 得到了
- 渐变
- 灰度
- 事业发展
- 指南
- 手
- 有
- 有帮助
- 于是
- 历史
- 创新中心
- HTTPS
- 鉴定
- 鉴定
- 确定
- 图片
- 图片
- 非常
- 影响力故事
- 实施
- 实施
- 进口
- 重要性
- 重要
- 改善
- 改善
- in
- 包括
- 包含
- 增加
- 增加
- 印度
- 指数
- 信息
- 输入
- 智能化
- 成
- 介绍
- IT
- 它的
- 工作
- JPG
- 只是
- 凯拉斯
- 键
- 会心
- 标签
- 标签
- 土地
- 后来
- 层
- 层
- 信息
- 学习用品
- 学习
- 长度
- 库
- 自学资料库
- 谎言
- 生活
- 生计
- 加载
- 离
- 机
- 机器学习
- 制成
- 主要
- 主要
- 使
- 制作
- 制作
- 手动
- 许多
- 问题
- 可能..
- 手段
- 测量
- 媒体
- 指标
- 矿物质
- 模型
- 模型
- 监控
- 更多
- 许多
- 多
- 命名
- 必要
- 需要
- 邻居
- 神经
- 神经元
- 数
- 麻木
- 营养
- 对象
- 物体检测
- of
- on
- 一
- 操作
- 有机
- OS
- 其他名称
- 我们的
- 产量
- 输出
- 拥有
- 部分
- 径
- 照片
- 的
- 植物
- 工厂
- 柏拉图
- 柏拉图数据智能
- 柏拉图数据
- 潜力
- 强大
- 都曾预测
- 预测
- 预测
- 预测
- 当下
- 印刷
- 市场问题
- 处理
- 生产
- 建议
- 提供
- 出版
- 纯粹
- 质量
- 随机
- RBF
- 回归
- 依靠
- 报告
- 那些
- 保留
- 回报
- 机器人
- 角色
- 根
- 同
- SAND
- 秤
- 科学
- 看到
- 选择
- 套数
- 设置
- 应该
- 如图
- 自
- 单
- 网站
- 智能
- So
- 土壤
- 空间的
- 具体的
- 速度
- 堆叠
- 个人陈述
- 步骤
- 合适的
- 提供
- SUPPORT
- 磁化面
- SVC
- 系统
- 任务
- 专业技术
- tensorflow
- 测试
- 比
- 这
- 其
- 他们
- 然后
- 那里。
- 博曼
- 他们
- Free Introduction
- 通过
- 耗时的
- 至
- 工具
- 主题
- 传统
- 产品培训
- 类型
- 类型
- 理解
- 单位
- 无用
- 使用
- 用过的
- 运用
- 验证
- 价值观
- 是
- 水
- 方法..
- we
- 网页
- 野草
- 什么是
- 什么是
- 这
- 而
- 为什么
- 将
- 合作
- X
- 黄色
- 生产
- 产量
- 您
- 和风网