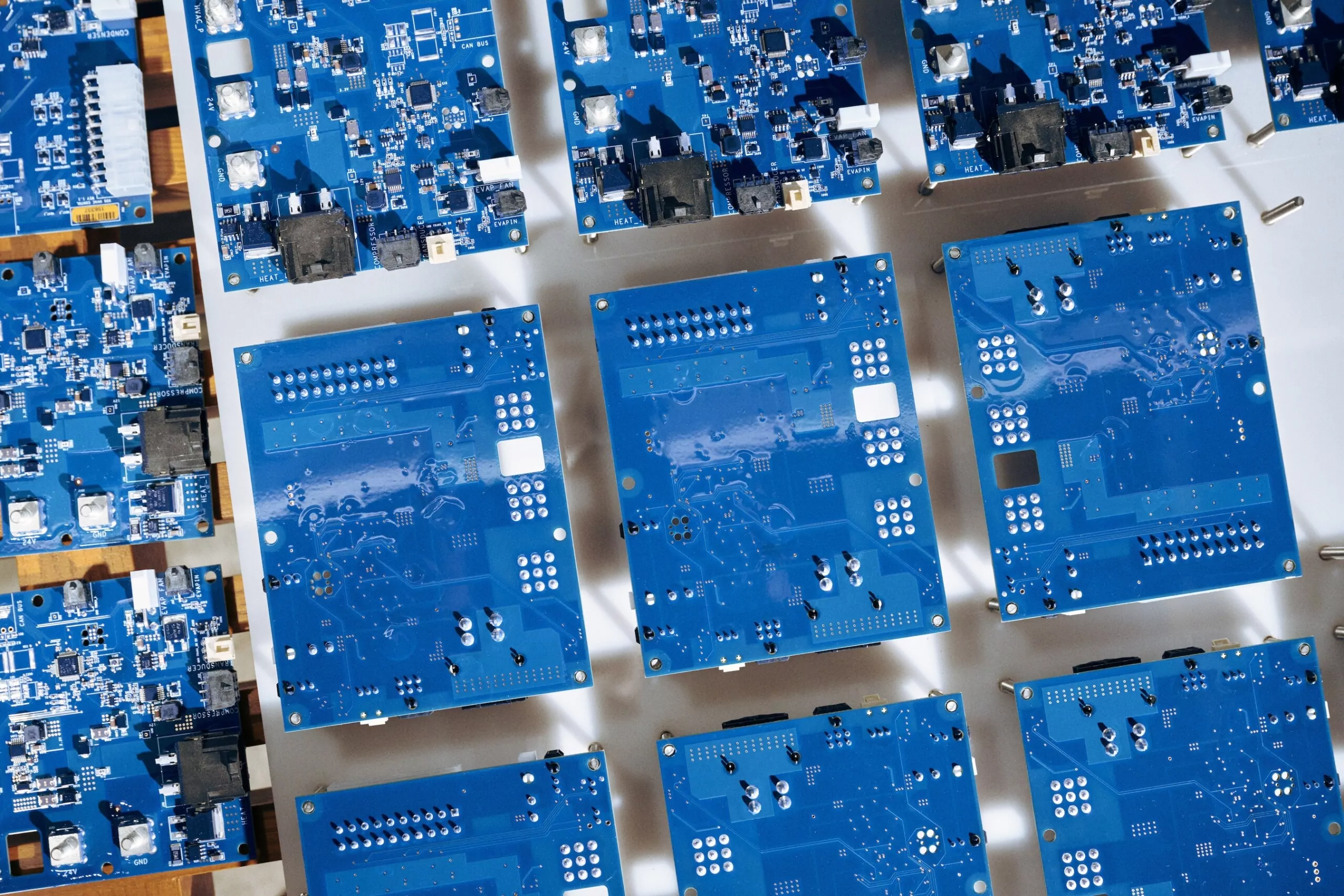
Online analytical processing (OLAP) database systems and artificial intelligence (AI) complement each other and can help enhance data analysis and decision-making when used in tandem. OLAP systems are designed to efficiently process and analyze large multidimensional datasets, while AI techniques extract insights and make predictions from OLAP data. As AI techniques continue to evolve, innovative applications in the OLAP domain are anticipated.
Defining OLAP today
OLAP database systems have significantly evolved since their inception in the early 1990s. Initially, they were designed for handling large volumes of multidimensional data, enabling businesses to perform complex analytical tasks, such as drill-down, zakasać i slice-and-dice.
Early OLAP systems were separate, specialized databases with unique data storage structures and query languages. This siloed approach often resulted in data redundancy and complexity, hampering integration with other business systems. In the 2010s, columnar OLAP (C-OLAP) and in-memory OLAP (IM-OLAP) technologies gained prominence. C-OLAP optimized data storage for faster query processing, while IM-OLAP stored data in memory to minimize data access latency and enable real-time analytics. These advancements further enhanced the performance and scalability of OLAP systems.
Today, OLAP database systems have become comprehensive and integrated data analytics platforms, addressing the diverse needs of modern businesses. They are seamlessly integrated with cloud-based data warehouses, facilitating the collection, storage and analysis of data from various sources.
Challenges of adopting cloud-based OLAP solutions
Cloud adoption for OLAP databases has become common due to scalability, elasticity and cost-efficiency advantages. However, organizations face challenges when adopting cloud-based OLAP solutions, such as:
- Migracja danych: Migrating large volumes of data to the cloud can be time-consuming and resource-intensive.
- Opóźnienie sieci: Geographic distances between data and users can introduce latency issues, affecting query performance.
- Optymalizacja kosztów: Optimizing cloud spending for OLAP resources can be challenging due to complex pricing models and resource utilization patterns.
- Bezpieczeństwo i zgodność: Ensuring data security and compliance with regulatory requirements in the cloud environment can be complex.
- Umiejętności i wiedza: Transitioning to cloud-based OLAP may require specialized skills and expertise in cloud computing and OLAP technologies.
Identifying best practices and benefits
In the realm of OLAP, AI’s role is increasingly important. To build a robust OLAP system, it should provide accessibility regardless of location and data type. It should also support various storage formats, such as block storage, object storage and file formats like Parquet, Avro and ORC.
OLAP database systems have evolved from specialized analytical tools into comprehensive data analytics platforms, empowering businesses to make informed decisions based on insights from large and complex datasets. Organizations can expect to reap the following benefits from implementing OLAP solutions, including the following.
1. Enhanced data analysis capabilities
- Multidimensional data exploration: OLAP allows users to explore data from multiple perspectives, identifying patterns and relationships that might not be apparent in traditional relational databases.
- Drill-down and roll-up analysis: OLAP enables users to drill down into specific data points or roll up to broader aggregates, gaining a comprehensive understanding of data trends.
- Slice-and-dice analysis: OLAP allows users to slice and dice data along various dimensions, isolating specific segments for in-depth analysis.
2. Lepsze podejmowanie decyzji
- Strategic planning and forecasting: OLAP helps businesses identify trends, patterns and potential risks, enabling better strategic planning and forecasting.
- Resource allocation optimization: OLAP provides insights into resource utilization and performance, enabling businesses to optimize resource allocation and improve efficiency.
- Performance benchmarking and trend analysis: OLAP allows businesses to benchmark performance against industry standards and identify areas for improvement.
3. Increased operational efficiency benefits
- Reduced data preparation time: OLAP data preparation capabilities streamline data analysis processes, saving time and resources.
- Wgląd w dane w czasie rzeczywistym: OLAP can provide real-time insights into business operations, enabling businesses to respond quickly to changing market conditions.
- Lepsze rozwiązywanie problemów: OLAP provides insights into the root causes of problems, enabling businesses to address issues more effectively.
4. Enhanced customer understanding benefits
- Segmentacja i targetowanie klientów: OLAP allows businesses to segment customers based on various characteristics, enabling targeted marketing campaigns.
- Customer lifetime value analysis: OLAP helps businesses identify high-value customers and develop strategies to retain them.
- Przewidywanie odejścia klientów: OLAP can identify customers at risk of churn, enabling businesses to implement retention strategies.
5. Przewaga konkurencyjna
Effective implementation of OLAP solutions can provide businesses with a competitive advantage by enabling them to gain a deeper understanding of market trends and customer behavior, identify new business opportunities and market segments, respond quickly to changing market conditions and customer demands and make more informed decisions about product development, pricing and marketing strategies.
Next-generation cloud OLAP database engines are expected to bring significant advancements. Here’s an overview of the key characteristics:
- Analityka oparta na sztucznej inteligencji: Integration of AI and machine learning capabilities into OLAP engines will enable real-time insights, predictive analytics and anomaly detection, providing businesses with actionable insights to drive informed decisions.
- Automated data preparation and cleansing: AI-powered data preparation tools will automate data cleaning, transformation and normalization, reducing the time and effort required for manual data preparation and improving data quality.
- Unified data fabric: OLAP systems will integrate seamlessly with cloud-based data warehouses and data lakes, providing a unified data fabric for comprehensive data analysis across various data sources.
- Real-time data processing and analytics: OLAP engines will handle real-time data streams and provide real-time insights, enabling businesses to make timely decisions based on up-to-date information.
- Hybrid transactional or analytical processing: OLAP systems will converge with transactional databases, enabling real-time analytics on transactional data, and providing a single platform for both operational and analytical processing.
- Skalowalność i elastyczność: OLAP engines will be highly scalable and elastic, automatically scaling up or down to handle fluctuating data volumes and user demands, optimizing resource utilization and cost efficiency.
- Architektura bezserwerowa: OLAP systems will adopt serverless architectures, eliminating infrastructure management and provisioning, allowing businesses to focus on data analysis rather than infrastructure maintenance.
- Ease of use and self-service analytics: OLAP systems will provide intuitive user interfaces, natural language query capabilities and self-service analytics features, enabling non-technical users to easily access and analyze data.
- Bezpieczeństwo i zgodność: OLAP systems will incorporate advanced security features, including data encryption, access controls and compliance with industry regulations to protect sensitive data and meet regulatory requirements.
- Cloud-native design and deployment: OLAP systems will be designed and optimized for cloud-native environments, leveraging cloud infrastructure and services for seamless deployment, management and scalability.
The future of OLAP database systems
In summary, the future of OLAP database systems is bright. Designed for cloud-native environments, they promise more efficient and data-driven decision-making for businesses, ushering in a new era of agility and insight.
IBM® watsonx.data™ is an enterprise-ready data store built on a data lakehouse architecture that enables hybrid cloud analytics workloads, such as data engineering, data science and business intelligence, through open source components with integrated IBM innovation. IBM watsonx.data is the next generation OLAP system that can help you make the most of your data.
Request a live IBM watsonx.data demo today
Więcej z danych i analityki

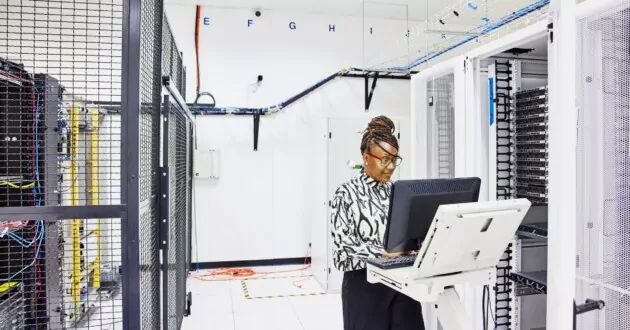

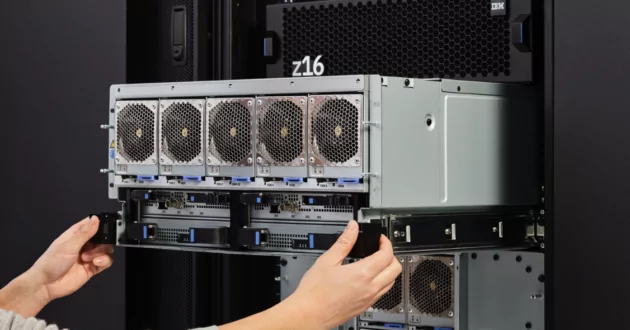
Biuletyny IBM
Otrzymuj nasze biuletyny i aktualizacje tematów, które dostarczają najnowszych informacji i spostrzeżeń na temat pojawiających się trendów.
Subskrybuj teraz
Więcej biuletynów
- Dystrybucja treści i PR oparta na SEO. Uzyskaj wzmocnienie już dziś.
- PlatoData.Network Pionowe generatywne AI. Wzmocnij się. Dostęp tutaj.
- PlatoAiStream. Inteligencja Web3. Wiedza wzmocniona. Dostęp tutaj.
- PlatonESG. Węgiel Czysta technologia, Energia, Środowisko, Słoneczny, Gospodarowanie odpadami. Dostęp tutaj.
- Platon Zdrowie. Inteligencja w zakresie biotechnologii i badań klinicznych. Dostęp tutaj.
- Źródło: https://www.ibm.com/blog/how-olap-and-ai-can-enable-better-business/
- :ma
- :Jest
- :nie
- :Gdzie
- $W GÓRĘ
- 1
- 17
- 2020
- 2023
- 28
- 29
- 300
- 31
- 39
- 400
- 43
- 50
- 80
- 9
- a
- O nas
- przyśpieszyć
- przyśpieszony
- dostęp
- dostępność
- dokładny
- w poprzek
- adres
- adresowanie
- przyjąć
- Przyjęcie
- Przyjęcie
- zaawansowany
- postępy
- zaliczki
- Korzyść
- Zalety
- przeciwny
- Reklama
- wpływający
- Po
- przed
- AI
- Zasilany AI
- Wszystkie kategorie
- przydział
- Pozwalać
- pozwala
- wzdłuż
- również
- Amazonka
- Amazon RDS
- wśród
- amp
- an
- analiza
- Analityczny
- analityka
- w czasie rzeczywistym sprawiają,
- i
- wykrywanie anomalii
- Przewiduje
- pozorny
- aplikacje
- podejście
- architektura
- SĄ
- obszary
- artykuł
- sztuczny
- sztuczna inteligencja
- Sztuczna inteligencja (AI)
- AS
- At
- publiczność
- autor
- zautomatyzować
- automatycznie
- dostępny
- z powrotem
- na podstawie
- BE
- stają się
- zanim
- zachowanie
- Benchmark
- Benchmarkingu
- Korzyści
- BEST
- Najlepsze praktyki
- Ulepsz Swój
- pomiędzy
- Blokować
- Blog
- Niebieski
- deska
- obie
- Przełamując
- Jasny
- przynieść
- Bringing
- szerszy
- budować
- Budowanie
- wybudowany
- biznes
- business intelligence
- Liderzy biznesu
- operacje biznesowe
- biznes
- przycisk
- by
- Kampanie
- CAN
- możliwości
- węgiel
- karta
- Kartki okolicznosciowe
- CAT
- Kategoria
- Przyczyny
- wyzwania
- wyzwanie
- wymiana pieniędzy
- Charakterystyka
- ZOBACZ
- koła
- klasa
- Sprzątanie
- Zamknij
- Chmura
- cloud computing
- infrastruktura chmurowa
- współpraca
- kolekcja
- kolor
- wspólny
- społeczność
- konkurencyjny
- Komplement
- kompleks
- kompleksowość
- spełnienie
- składniki
- wszechstronny
- komputer
- Wizja komputerowa
- computing
- Warunki
- Pojemnik
- kontynuować
- ciągły
- bez przerwy
- kontroli
- zbieżny
- rdzeń
- Koszty:
- CSS
- zwyczaj
- klient
- zachowanie klienta
- Klientów
- codziennie
- codzienne transakcje
- dane
- dostęp do danych
- analiza danych
- Analityka danych
- integracja danych
- punkty danych
- Przygotowywanie danych
- analiza danych
- jakość danych
- nauka danych
- bezpieczeństwo danych
- przechowywanie danych
- magazyn danych
- sterowane danymi
- Baza danych
- Bazy danych
- zbiory danych
- Data
- grudzień
- Podejmowanie decyzji
- Decyzje
- głębiej
- Domyślnie
- definicje
- dostarczyć
- wymagania
- Demo
- Wdrożenie
- opis
- Wnętrze
- zaprojektowany
- Wykrywanie
- rozwijać
- deweloperzy
- oprogramowania
- Wymiary
- bezpośrednio
- inny
- do
- domena
- na dół
- napęd
- z powodu
- każdy
- Wcześnie
- z łatwością
- gospodarka
- Efektywne
- faktycznie
- efektywność
- wydajny
- skutecznie
- wysiłek
- eliminując
- powstanie
- wschodzących
- uprawniającej
- umożliwiać
- Umożliwia
- umożliwiając
- szyfrowanie
- Inżynieria
- silniki
- wzmacniać
- wzmocnione
- zapewnić
- zapewnia
- zapewnienie
- Wchodzę
- przedsiębiorstwa
- Cały
- Środowisko
- środowiska
- Era
- szczególnie
- Eter (ETH)
- EVER
- ewolucja
- ewoluuje
- ewoluowały
- Podniecenie
- Wyjście
- oczekiwać
- spodziewany
- ekspertyza
- odkryj
- wyciąg
- tkanina
- Twarz
- ułatwienie
- fałszywy
- szybciej
- Korzyści
- karmienie
- czuć
- filet
- Skupiać
- obserwuj
- następujący
- czcionki
- W razie zamówieenia projektu
- przyszłościowe myślenie
- Fundacja
- Fundamenty
- Ramy
- od
- dalej
- przyszłość
- Wzrost
- zdobyte
- zyskuje
- generacja
- generatywny
- generatywne sieci przeciwne
- generatywna sztuczna inteligencja
- generator
- geograficzny
- otrzymać
- Krata
- Rosnąć
- miał
- uchwyt
- Prowadzenie
- Have
- Nagłówek
- wysokość
- pomoc
- pomaga
- wysoko
- W jaki sposób
- Jednak
- HTTPS
- Hybrydowy
- Chmura hybrydowa
- IBM
- ICO
- ICON
- zidentyfikować
- identyfikacja
- obraz
- zdjęcia
- ogromny
- Rezultat
- wdrożenia
- realizacja
- wdrożenia
- wykonawczych
- ważny
- podnieść
- ulepszony
- poprawa
- poprawy
- in
- informacje
- początek
- obejmuje
- Włącznie z
- włączać
- wzrosła
- coraz bardziej
- wskaźnik
- przemysł
- standardy przemysłowe
- Informacja
- poinformowany
- Infrastruktura
- początkowo
- Innowacja
- Innowacyjny
- wgląd
- spostrzeżenia
- integralny
- integrować
- zintegrowany
- integracja
- Inteligencja
- interfejsy
- najnowszych
- przedstawiać
- intuicyjny
- problemy
- IT
- iteracja
- JEGO
- samo
- John
- jpg
- Klawisz
- jezior
- język
- Języki
- duży
- Utajenie
- problemy z latencją
- firmy
- lider
- Przywódcy
- Przywództwo
- nauka
- lewarowanie
- dożywotni
- lubić
- relacja na żywo
- miejscowy
- lokalny
- lokalizacja
- maszyna
- uczenie maszynowe
- magia
- Mainstream
- konserwacja
- robić
- i konserwacjami
- podręcznik
- wiele
- rynek
- warunki rynkowe
- udział w rynku
- Trendy rynkowe
- Marketing
- Kampanie marketingowe
- Strategie marketingowe
- dojrzałość
- Maksymalna szerokość
- Może..
- Poznaj nasz
- Pamięć
- może
- migracja
- min
- zminimalizować
- minuty
- ML
- Aplikacje mobilne
- modele
- Nowoczesne technologie
- jeszcze
- bardziej wydajny
- większość
- wielokrotność
- O imieniu
- Naturalny
- Język naturalny
- Nawigacja
- wymagania
- sieci
- Nowości
- Newsletter
- Następny
- nietechniczne
- nic
- listopad
- już dziś
- z naszej
- przedmiot
- Przechowywanie obiektów
- of
- poza
- Oferty
- często
- on
- Online
- tylko
- koncepcja
- open source
- operacyjny
- operacje
- Szanse
- Optymizm
- Optymalizacja
- zoptymalizowane
- optymalizacji
- or
- organizacji
- Inne
- ludzkiej,
- przegląd
- strona
- wzmacniacz
- wzory
- wykonać
- jest gwarancją najlepszej jakości, które mogą dostarczyć Ci Twoje monitory,
- perspektywy
- PHP
- kawałek
- planowanie
- Platforma
- Platformy
- plato
- Analiza danych Platona
- PlatoDane
- grał
- wtyczka
- zwrotnica
- polityka
- Popularny
- popularność
- position
- Post
- potencjał
- praktyki
- Przewidywania
- proroczy
- Analityka predykcyjna
- przygotowanie
- pilny
- wycena
- pierwotny
- prywatność
- problemy
- wygląda tak
- procesów
- przetwarzanie
- Produkt
- rozwój produktów
- rozgłos
- obietnica
- chronić
- zapewniać
- zapewnia
- że
- jakościowy
- jakość
- ilościowy
- Kwartał
- pytanie
- Szybki
- szybko
- szybki
- raczej
- Czytający
- Prawdziwy świat
- w czasie rzeczywistym
- dane w czasie rzeczywistym
- królestwo
- zbierać
- niedawny
- redukcja
- Bez względu
- regulamin
- regulacyjne
- Relacje
- Renesans
- wymagać
- wymagany
- wymagania
- Badacze
- Zasób
- utylizacja zasobów
- zasobochłonne
- Zasoby
- Odpowiadać
- czuły
- wynikał
- zachować
- retencja
- dochód
- Ryzyko
- ryzyko
- roboty
- krzepki
- Rola
- Rolka
- zakasać
- korzeń
- run
- oszczędność
- Skalowalność
- skalowalny
- Skala
- skala ai
- skalowaniem
- nauka
- Naukowcy
- Ekran
- skrypty
- bezszwowy
- płynnie
- bezpieczne
- bezpieczeństwo
- widzieć
- poszukuje
- segment
- segmentacja
- Segmenty
- Samoobsługa
- wrażliwy
- seo
- oddzielny
- Bezserwerowe
- Usługi
- Share
- powinien
- znaczący
- znacznie
- wyciszony
- Silosy
- ponieważ
- pojedynczy
- witryna internetowa
- umiejętności
- Plaster
- mały
- Tworzenie
- Rozwiązania
- Źródło
- Źródła
- wyspecjalizowanym
- specyficzny
- Spędzanie
- Łącza
- kwadraty
- standardy
- początek
- przechowywanie
- sklep
- przechowywany
- Strategiczny
- strategie
- opływowy
- Strumienie
- zbudowany
- Struktury
- subskrybuj
- taki
- PODSUMOWANIE
- wsparcie
- Badanie
- SVG
- syntetyczny
- dane syntetyczne
- system
- systemy
- Żywy obraz
- Mówić
- Tandem
- ukierunkowane
- zadania
- Techniki
- Technologies
- trzeciorzędowy
- XNUMX
- niż
- że
- Połączenia
- Przyszłość
- świat
- ich
- Im
- motyw
- Te
- one
- to
- myśl
- myśl przywództwo
- Przez
- czas
- czasochłonne
- aktualny
- Tytuł
- do
- dzisiaj
- razem
- narzędzia
- Top
- aktualny
- tradycyjny
- Trening
- transakcyjny
- transakcje
- Transformacja
- przekształcony
- Transformatory
- przejście
- Trend
- Trendy
- biliony
- Zaufaj
- i twitterze
- rodzaj
- typy
- w trakcie
- zrozumienie
- Ujednolicony
- wyjątkowy
- nowomodny
- Nowości
- URL
- posługiwać się
- używany
- Użytkownik
- Użytkownicy
- wprowadzenie
- za pomocą
- wartość
- Wartości
- różnorodny
- Wideo
- wizja
- kłęby
- W
- sposoby
- we
- były
- jeśli chodzi o komunikację i motywację
- Podczas
- szerszy
- będzie
- w
- WordPress
- świat
- świat
- napisany
- lat
- ty
- Twój
- zefirnet